5 Big Data and ML in Enterprise Software Revolution
Table of Contents
The fusion of Big Data and Machine Learning in enterprise software development has ignited a revolutionary shift, fundamentally altering how businesses operate. By harnessing vast datasets and ML algorithms, organizations gain unprecedented insights, driving strategic decision-making and operational efficiency.
Real-world instances abound, from predictive maintenance in manufacturing, where ML algorithms anticipate equipment failures to minimize downtime, to dynamic pricing strategies in retail, leveraging Big Data analytics to optimize pricing models and maximize profits. This convergence heralds a new era of innovation, empowering enterprises to adapt and thrive in an increasingly data-driven world.
Big Data and ML in Enterprise Software
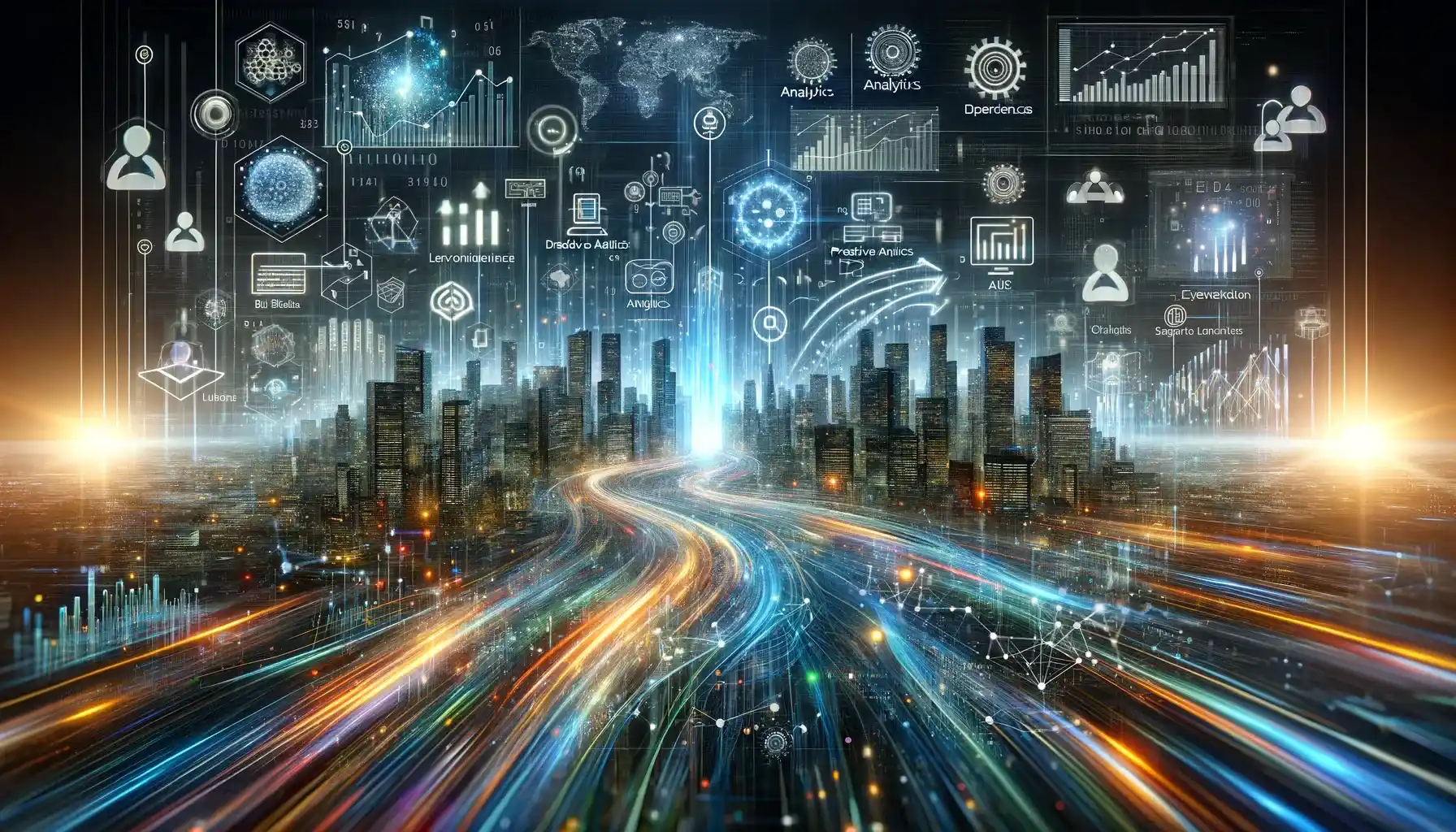
Big Data and ML in Enterprise Software revolutionize business operations by leveraging immense volumes of data generated from various sources such as customer interactions, transaction records, and operational metrics. This data serves as a valuable resource, providing insights into market trends, customer behavior, and operational efficiency.
ML algorithms analyze this Big Data, identifying patterns and deriving actionable insights. ML models continuously learn from the data, enabling them to make data-driven predictions and decisions.
1. Enhanced Decision-Making
In today’s dynamic business landscape, harnessing the power of Big Data and ML in enterprise software has become imperative for informed decision-making. Through advanced ML algorithms, organizations can sift through immense datasets with ease, uncovering hidden correlations and trends that may elude human analysis. This capability empowers businesses to make strategic decisions grounded in predictive analytics and real-time insights.
For instance, in retail, ML algorithms analyze customer purchasing patterns in real-time to predict future trends, enabling companies to adjust inventory levels and marketing strategies accordingly. Similarly, in finance, ML algorithms process vast streams of market data to identify investment opportunities and mitigate risks swiftly, ensuring timely and informed decisions that drive competitive advantage.
By integrating Big Data and ML into enterprise software, organizations can enhance decision-making processes, driving efficiency and profitability in today’s data-driven world.
2. Personalized Experiences
Big Data & Machine Learning revolutionize customer interactions within enterprise software by enabling personalized experiences at scale. Through analyzing vast datasets, these technologies discern individual preferences and behaviors, powering recommendation engines as seen in streaming platforms or e-commerce sites. ML algorithms drive dynamic user interfaces and facilitate personalized marketing campaigns, enhancing customer engagement and fostering loyalty.
For instance, platforms like Netflix utilize ML to suggest content based on viewing history, while e-commerce giants like Amazon tailor product recommendations based on past purchases and browsing behavior. This symbiotic fusion of Big Data and ML in enterprise software redefines customer experiences, making them more intuitive, relevant, and captivating.
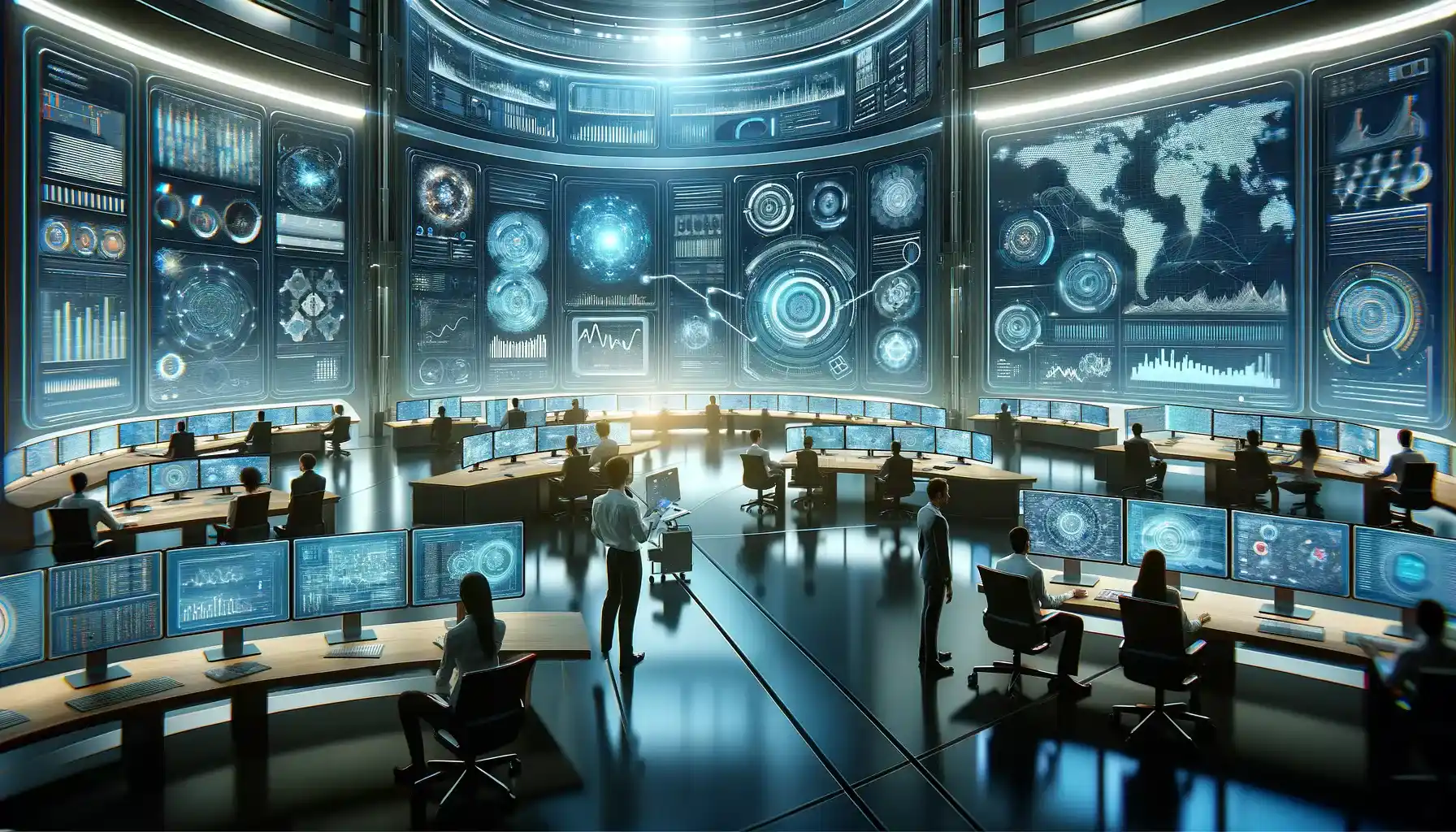
3. Operational Efficiency
Big Data analytics integrated with ML capabilities within Enterprise Software revolutionize operations by enhancing resource allocation, streamlining processes, and pinpointing avenues for enhancement. From optimizing supply chain management by predicting demand patterns to enabling predictive maintenance in manufacturing equipment, the synergy of Big Data and ML in Enterprise Software ensures maximal efficiency while minimizing costs.
For instance, companies like Amazon leverage Big Data analytics and ML algorithms to forecast customer demands and optimize inventory management, leading to reduced overheads and improved customer satisfaction. Similarly, predictive maintenance solutions by companies like General Electric utilize machine learning algorithms to anticipate equipment failures, thus minimizing downtime and maximizing operational uptime in industrial settings.
4. Risk Management
The integration of Big Data and ML capabilities revolutionizes risk management practices. By harnessing vast datasets, ML models can swiftly analyze complex patterns to assess risks, detect anomalies, and preempt potential threats. In financial sectors, this translates to robust fraud detection systems, where ML algorithms continuously scrutinize transactions for any irregularities, safeguarding assets against fraudulent activities.
Similarly, in cybersecurity, ML-powered enterprise software acts as a proactive shield, identifying and mitigating security breaches in real-time, thereby fortifying organizational defenses against cyber threats.
Instances include systems that monitor network traffic to flag suspicious activities indicative of potential cyber-attacks and platforms that employ anomaly detection algorithms to identify abnormal user behavior, preventing unauthorized access to sensitive data. Through the synergy of Big Data and ML in enterprise software, organizations can effectively bolster security measures, ensuring the protection of valuable assets and maintaining trust in their operations.
5. Continuous Improvement
Big Data and ML in Enterprise Software, continuous improvement isn’t just a concept—it’s a fundamental practice. ML models undergo iterative refinement to align with evolving business dynamics and market conditions. Through ingesting new data streams and fine-tuning algorithms, enterprise software adapts in real time, enhancing predictive accuracy and maintaining competitiveness.
For instance, in financial services, fraud detection systems continually update algorithms to detect emerging patterns of fraudulent behavior, ensuring robust security measures. Likewise, in e-commerce, recommendation engines evolve to personalize user experiences based on real-time browsing behaviors, enhancing customer satisfaction and retention. This iterative learning loop ensures that enterprise software remains agile and responsive to the ever-changing demands of the modern business environment.
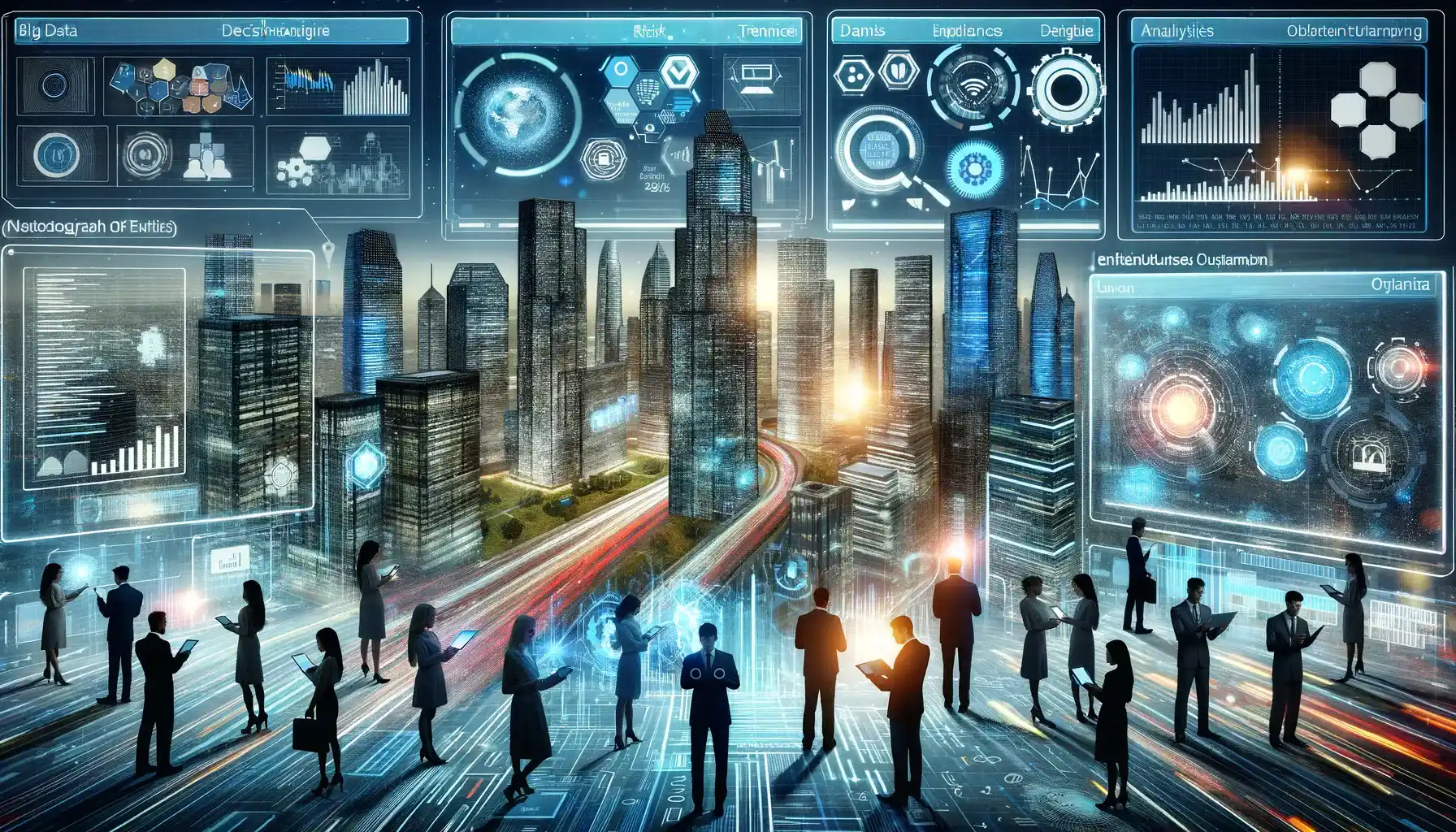
Challenges and Opportunities
Big Data and ML in enterprise software offer substantial opportunities for businesses across diverse industries. Big Data encompasses vast volumes of structured and unstructured data sourced from platforms like social media, sensors, devices, and transactions. In contrast, Machine Learning, a subset of artificial intelligence (AI), empowers systems to autonomously learn and enhance performance based on experience without explicit programming. Integrating Big Data and ML in enterprise software enables organizations to extract valuable insights, enhance decision-making, and optimize operations by leveraging the wealth of data available to them.
Here’s an elaboration on the opportunities, challenges, and strategies for leveraging Big Data and ML in enterprise software:
- Opportunities:
- Data-Driven Decision Making: Big Data & ML in software enterprises empower organizations to make data-driven decisions by extracting valuable insights from large datasets. For instance, in retail, companies can analyze customer purchase patterns to optimize inventory management and personalize marketing strategies.
- Predictive Analytics: ML algorithms can utilize historical data to forecast future trends and outcomes, a capability with significant value across sectors. In finance, these algorithms predict market trends, while in healthcare, they aid in early disease diagnosis using patient data. Integrating Big Data and ML in enterprise software enhances this predictive capacity, offering businesses actionable insights for strategic decision-making and innovation.
- Enhanced Customer Experience: the integration of Big Data and ML allows organizations to enhance their products and services by analyzing customer interactions and feedback. For instance, streaming platforms like Netflix utilize ML algorithms to recommend personalized content based on viewing history. This application showcases how Big Data and ML in enterprise software can drive personalized experiences, meeting customer needs and preferences more effectively.
- Operational Efficiency: Big Data analytics can streamline processes and optimize operations by identifying inefficiencies and areas for improvement. For instance, manufacturing companies use ML algorithms to predict equipment maintenance needs, reducing downtime and costs.
- Challenges:
- Data Security and Privacy: Big Data and ML in enterprise software involve handling large volumes of sensitive data, raising concerns about security breaches and unauthorized access. Organizations must implement robust security measures and compliance frameworks to protect data integrity and privacy. This entails ensuring that data is securely stored, transmitted, and accessed, with encryption and access controls in place. Additionally, continuous monitoring and auditing of data access and usage are essential to detect and mitigate potential security threats. By prioritizing data security and compliance within Big Data and ML initiatives, organizations can mitigate risks and build trust with customers and stakeholders.
- Skill Shortage: Big Data and ML in Enterprise Software, a critical challenge arises from the scarcity of proficient data scientists and ML engineers. This shortage hampers developing and deploying advanced analytics solutions essential for effective implementation. Therefore, recruiting and retaining talent with expertise in Big Data technologies is paramount to ensuring successful integration and leveraging the full potential of these technologies within enterprise software.
- Data Quality and Integration: Ensuring data quality and reliability when collecting data from diverse sources is a challenge in Big Data and ML in Enterprise Software. Integrating data from disparate sources into a unified system for analysis requires careful planning and robust data governance.
- Strategies for Success:
- Data Governance Frameworks: Establishing comprehensive data governance frameworks helps organizations ensure data quality, security, and compliance with regulations such as GDPR and CCPA.
- Investment in Talent Development: Organizations should invest in training programs and partnerships with academic institutions to develop a skilled workforce proficient in Big Data technologies and ML algorithms.
- Collaboration Across Departments: Collaboration between data scientists, IT professionals, and business stakeholders is essential for identifying use cases, designing analytics solutions, and deriving actionable insights from data.
- Continuous Innovation: Enterprises should foster a culture of innovation and experimentation to explore new applications of Big Data and ML technologies that drive business growth and competitive advantage.
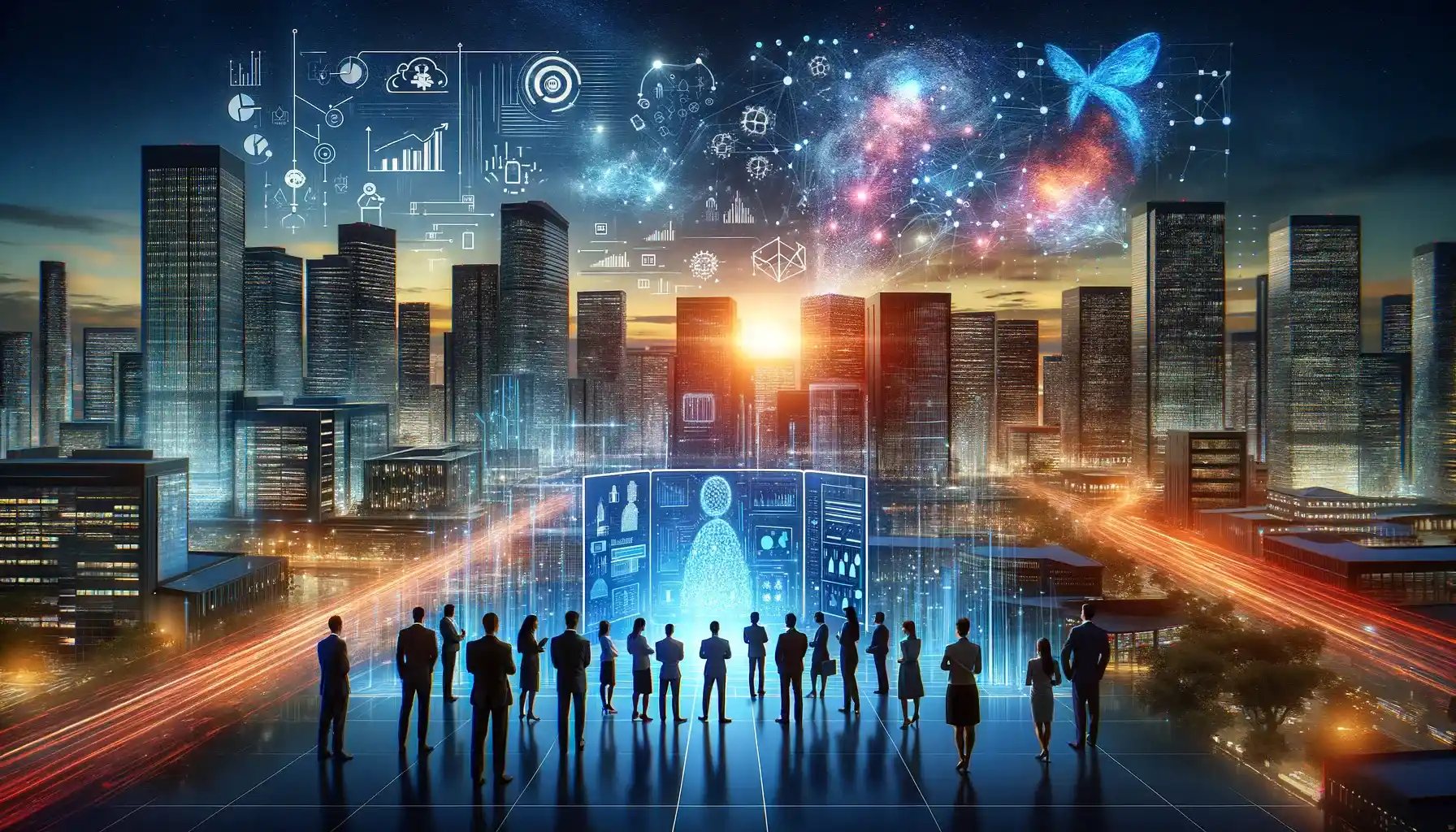
Conclusion
The fusion of Big Data and ML in enterprise software development heralds a new era of innovation, efficiency, and competitiveness. By harnessing the power of these technologies, businesses can unlock actionable insights, drive informed decision-making, and stay ahead in today’s dynamic market landscape.
As the revolution continues, organizations must embrace the transformative potential of Big Data and ML to thrive in the digital age.
Featured Insights
Integrating Real-Time Weather Data into Your Travel and Navigation App
The Future is Now: AI and ML Technologies Reshaping Healthcare
Introduction to Digital Twin Technology in Manufacturing with Unprecedented Efficiency and Innovation
Adobe’s Firefly AI: New Tools for Photoshop and Illustrator
Introducing Meta Imagine Me: Your Digital Doppelganger
AI in Gaming is Transforming the Industry