Table of Contents
In today’s data-driven world, organizations are facing increasing challenges in managing vast amounts of data. Traditional architectures such as centralized data warehouses or data lakes lead to bottlenecks, data silos, and scalability issues. To address these problems, a new paradigm called Data Mesh has emerged. It advocates a decentralized approach, empowering individual domains to manage their own data as a product. This shift allows organizations to scale, be agile, and align data with business goals.
What is Data Mesh?
It is the next generation of modern data architecture over monolithic data systems. It is combined with four key principles. This involves:
- Domain-Oriented Decentralized Data Ownership: Each domain, for example marketing, sales, and operations, owns and manages its data, being more aligned with domain-specific needs.
- Data as a Product: Data is treated as a product with clear ownership, quality standard, and ease of access.
- Self-Serve Data Infrastructure: Teams are given tools and platforms to manage and consume data without much dependence on central IT teams.
- Federated Computational Governance: Governance policies are implemented across domains to ensure compliance, security, and interoperability.
It is not like traditional architectures that focus on decentralizing ownership and operational responsibilities.
What problems does Data Mesh solve?
It is the removal of conventional centralized data architecture limitations because it decentralizes the ownership of data, scales better, and removes bottlenecks, thus improving accountability, data quality, and easier integration due to reduced data silos. It also optimizes governance, reduces costs on infrastructure, and allows for modern use cases in AI, ML, and real-time analytics.
It addresses these challenges by:
- Decentralizing data ownership, so that domain teams can treat their data as a product
- Ensuring interoperability through standardized governance and self-serve data platforms.
- Eliminating bottlenecks through a centralized data team.
- Scalability and efficiency increase as data becomes more accessible and adaptable for business needs.
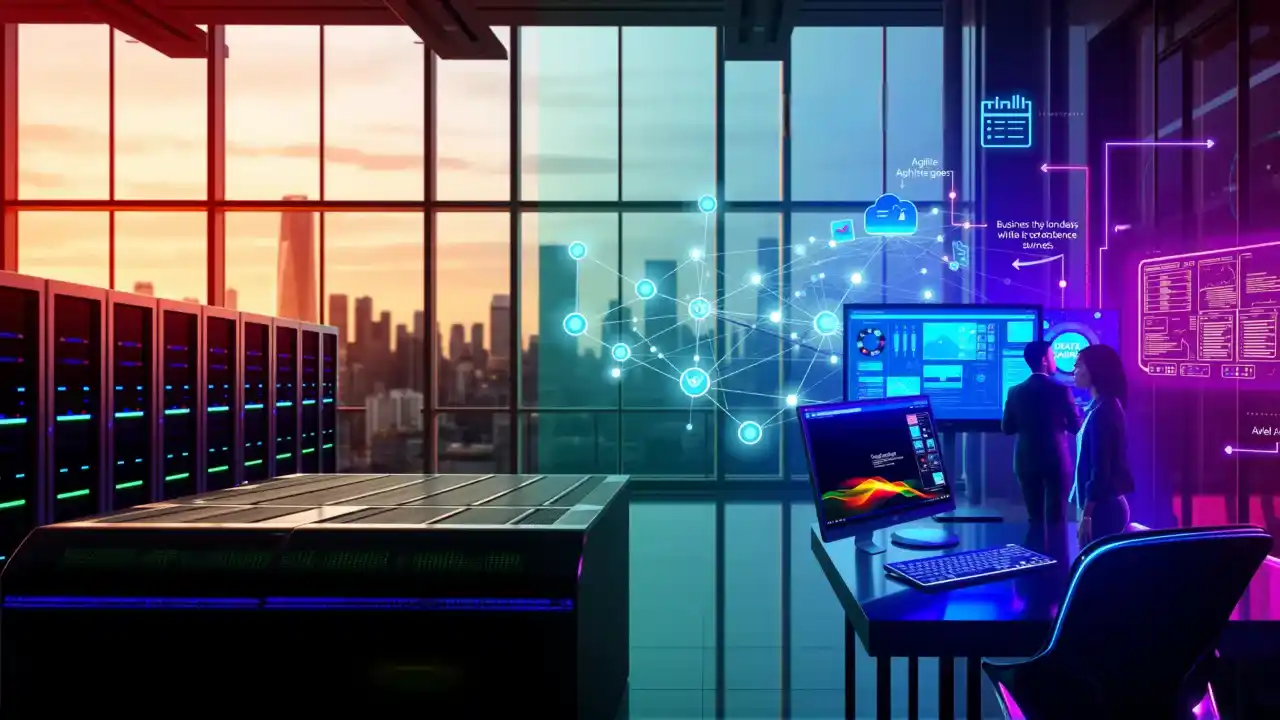
How can AWS boost your Data Mesh?
AWS provides a set of services and tools that help support the Data Mesh architecture through decentralized data ownership, interoperability, self-serve data infrastructure, and governance. Here’s how AWS can assist:
- Decentralized Data Storage & Access – Amazon S3 acts as a scalable and secure data lake that lets domain teams store and manage their own data autonomously.
- Data as a Product & Interoperability – AWS Glue offers serverless data integration, ETL, and cataloging for cross-domain interoperability.
- Self-Serve Data Infrastructure – Amazon API Gateway provides data product APIs where access to domain-owned data is secure and managed.
- Governance, Security & Compliance – Access to the data products is controlled by AWS IAM (Identity and Access Management) for security and compliance.
- Real-Time & AI/ML Capabilities – Amazon Kinesis supports real-time data streaming for event-driven architectures.
AWS provides a scalable and efficient architecture of Data Mesh by providing decentralized storage, data interoperability, self-serve analytics, governance, and AI/ML capabilities. Organizations will be able to move from monolithic data architectures toward domain-driven and productized data ecosystems.
Benefits of a Data Mesh Architecture
- Scalability: By decentralizing data ownership, organizations can handle data growth without centralized bottlenecks.
- Improved Data Quality: Domain-specific ownership guarantees the accuracy, relevance, and up-to-datedness of data.
- Enhanced Agility: Teams can independently build and access data products, thus reducing delays and accelerating decision-making.
- Business Alignment: It closes the gap between technical data management and business outcomes, ensuring that data serves strategic goals.
Key Components of a Data Mesh
A successful implementation of it rests on many fundamental building blocks.
- Domains: Each business unit becomes an independent data owner and manager, which enables domain-specific innovation.
- Data Products: Data is wrapped up as easily accessible, reusable, and discoverable products with proper documentation.
- Self-Serve Platform: Centralized infrastructure tools support decentralized data operations. This helps avoid silos.
- Governance: Policies and standards are developed in order to preserve security, compliance, and interoperability between domains.
Challenges in Implementing Data Mesh
- Cultural Shifts: Going decentralized needs a change in organizational mind-set and structure.
- Technical Consistency: Interoperability and technical standards across domains are complex.
- Infrastructure Requirements: Building a robust self-serve platform demands significant investment and expertise.
- Balancing Decentralization with Governance: It is critical to get the right amount of autonomy versus oversight.
Comparing Data Mesh to Other Architectures
- Data Mesh vs. Data Lake: Data Mesh decentralized ownership and operation, whereas the data lakes have centralized storage for data but result in silos and poor quality.
- Data Mesh vs. Data Warehouse: Data Mesh focuses on domain-driven ownership and scalability, unlike the traditional data warehouse.
- Hybrid Approaches: Organizations can transition gradually by integrating it’s principles into existing architectures.
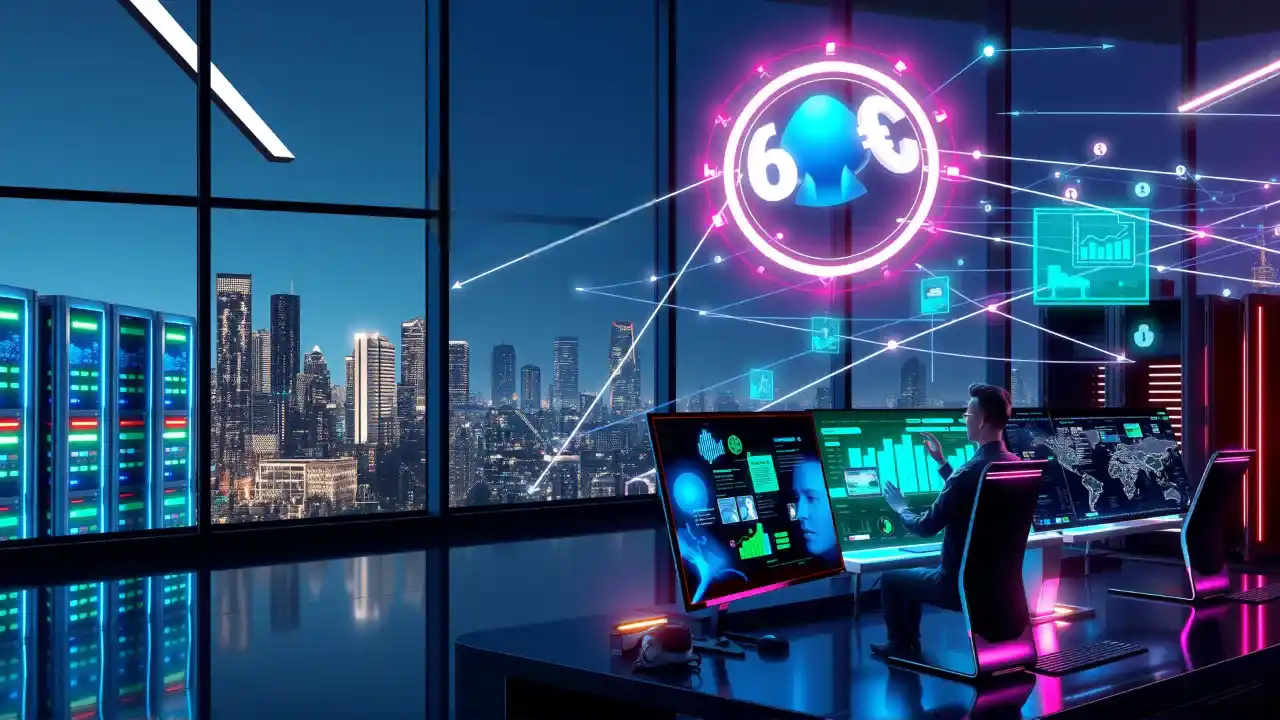
Difference between Data mesh and Data fabric
Aspect | Data Mesh | Data Fabric |
---|---|---|
Architecture | Decentralized, domain-oriented | Centralized, unified data management |
Data Ownership | Owned and managed by domain teams | Centralized ownership, managed by IT teams |
Use Case Suitability | Best for large, complex organizations with domain-driven data needs. | Suitable for organizations requiring unified, integrated data access. |
Case Studies and Industry Examples
Many organizations have been able to implement Data Mesh to transform their data ecosystems.
- JPMorgan Chase: The financial services giant aligned its data technology solutions with its data product strategy through a data mesh architecture. This allows data sharing across the enterprise while ensuring data owners are in control and have visibility of their data.
- Netflix: It has empowered individual teams to manage their specific data domains by decentralizing its data infrastructure and creating domain-oriented platform teams. This shift has increased autonomy and faster decision-making processes, which enables Netflix to scale operations while maintaining flexibility and agility.
- LinkedIn restructured the company’s data infrastructure into smaller independent units called “data product teams.” These cross-functional teams assume end-to-end ownership of their specific products-including data pipelines and analytics services. Through this structure, LinkedIn is able to deliver customized insights more efficiently while encouraging collaboration among other business units.
- Uber: Transitioning from monolithic centralized systems to a data mesh framework, Uber adopted domain-oriented distributed architectures. This change improved scalability and reduced bottlenecks in processing massive amounts of real-time streaming data, leading to more accurate ride estimations, optimized driver routes, and enhanced user experiences.
The key lessons from these examples are the need for clear definitions of the domain, robust infrastructure, and a strong governance framework.
Steps to Implement a Data Mesh
- Assess Organizational Readiness: Review current data architecture, workflows, and team structures.
- Define Domains: Identify business units and align data ownership with domain expertise.
- Build a Self-Serve Infrastructure: Develop tools and platforms that allow people to perform their data operations.
- Establish Governance Models: Federated policies to be implemented to ensure compliance and consistency.
- Iterate and Measure Success: At all times refine the system based on feedback and performance metrics.
Future of Data Mesh
As data volumes grow and business needs evolve, it will play a central role in the modern management of data. Some emerging trends include:
- Integration with AI and Automation: Using machine learning to augment data products and governance.
- Adoption of Cloud-Native Tools: Simplify infrastructure by adopting cloud-based solutions.
- Focus on Business Value: Aligning it implementations with measurable business outcomes.
Conclusion
It is an evolution in managing data at scale, as decentralized ownership, product-thinking about data, and self-serve infrastructure create the capability for businesses to bypass the problems traditional architectures throw in their path. Challenges aside, scalability, agility, and the ability to directly align with business objectives all add up to why forward-thinking organizations may find it an appealing proposition.
READ MORE:
Nuclear Energy: The Backbone of the Tech Revolution
Synthetic Media: Inspiring the Future of IT Training through Limitless Virtual Reality