Table of Contents
Introduction to Machine Learning
Machine Learning (ML) is a fascinating field of Artificial Intelligence (AI) research and practice where we investigate how computer agents can improve their perception, cognition, and action with experience. Machine Learning is about machines improving from data, knowledge, experience, and interaction. Machine Learning utilizes a variety of techniques to intelligently handle large and complex amounts of information build upon foundations in many disciplines, including statistics, knowledge representation, planning and control, databases, causal inference, computer systems, machine vision, and natural language processing.
Understanding Machine Learning in Healthcare
The healthcare sector has long been an early adopter of and benefited greatly from technological advances. These days, Machine Learning (ML) algorithms enhance our ability to understand diseases, diagnose them, and treat them. We’re standing on the brink of a new age where we can further improve healthcare using Machine Learning. The application of Machine Learning in Healthcare has been a significant breakthrough and is transforming the healthcare industry rapidly. Healthcare providers now leverage complex algorithms derived from machine learning to gain insights from their data, leading to improved outcomes for patients.
How ML Algorithms Work
Machine Learning algorithms, or models, are essentially mathematical or statistical methods. The primary purpose of ML models is to find patterns or structures within input data. From a statistical perspective, ML involves finding data distributions or boundaries in data sets. If you’ve ever used a trending news feed, photo tagging feature, voice-activated assistant, recommendation system, or targeted advertising, then you’ve interacted with machine learning algorithms. In the context of healthcare, these algorithms can be used to predict a multitude of outcomes, from diseases like cancer to hospital readmissions.
Importance of ML in Healthcare
Machine Learning is causing a revolution in healthcare, being able to handle massive amounts of data, and identify patterns that humans cannot.
Innovations in the realm of artificial intelligence and machine learning are propelling significant enhancements and progress in the healthcare sector. These advancements span across various aspects of healthcare, such as refining clinical procedures, accelerating the process of drug discovery and distribution, enhancing surgical methods, improving medical assistance, and optimizing data handling.
As per data from Statista, the global healthcare market’s AI segment was valued at more than $11 billion in 2021. Projections suggest a surge to an impressive $187 billion by 2030, indicating a compound annual growth rate (CAGR) of 37% from 2022 to 2030. This substantial growth underscores the increasing reliance and integration of AI technologies in healthcare systems worldwide.
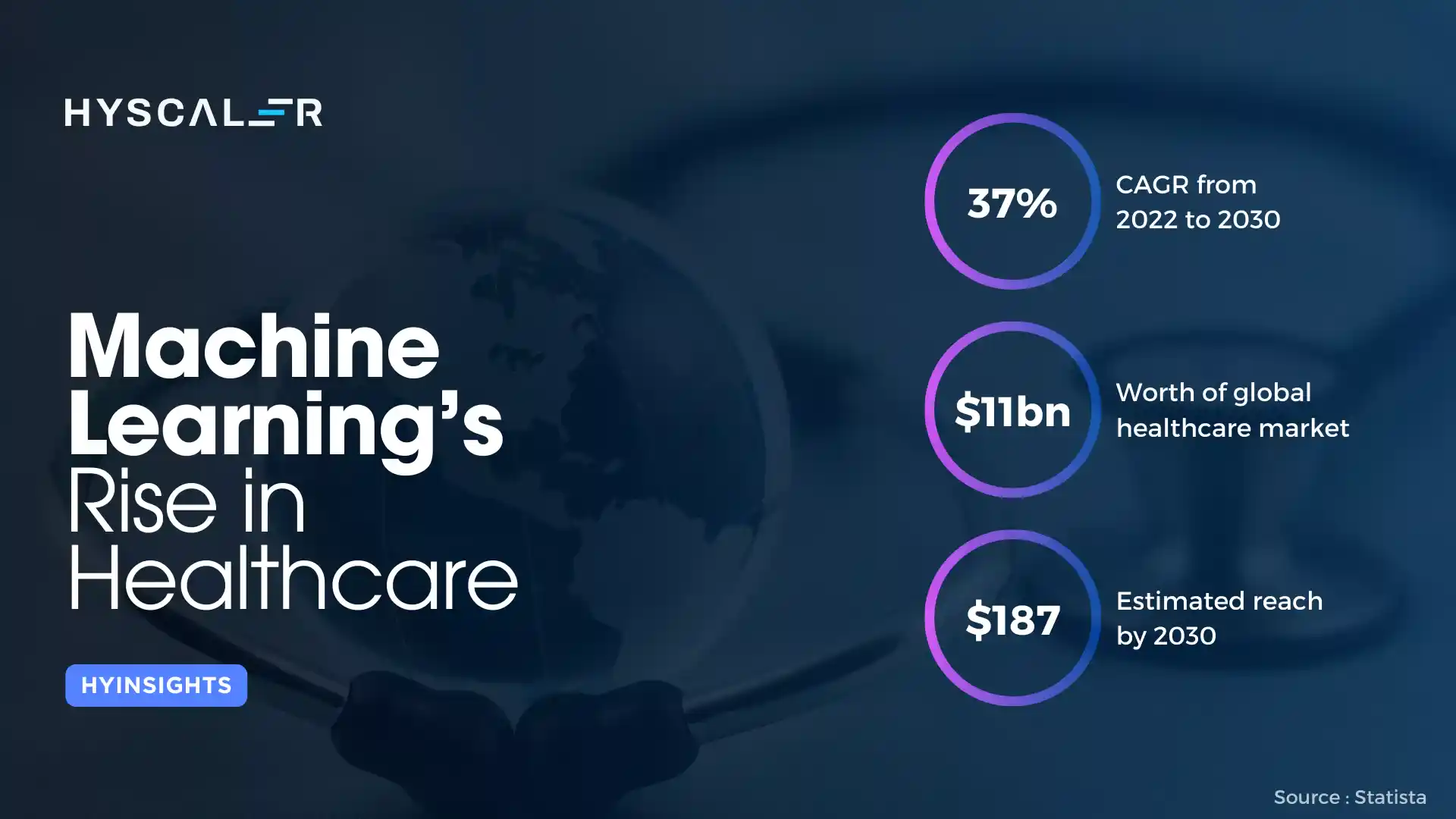
The Role of AI in Healthcare
Artificial Intelligence (AI) in healthcare is the use of complex algorithms and software to emulate human cognition in the analysis of complicated medical data. Specifically, AI is the ability of computer algorithms to approximate conclusions without direct human input. The primary aim of health-related AI applications is to analyze relationships between prevention or treatment techniques and patient outcomes.
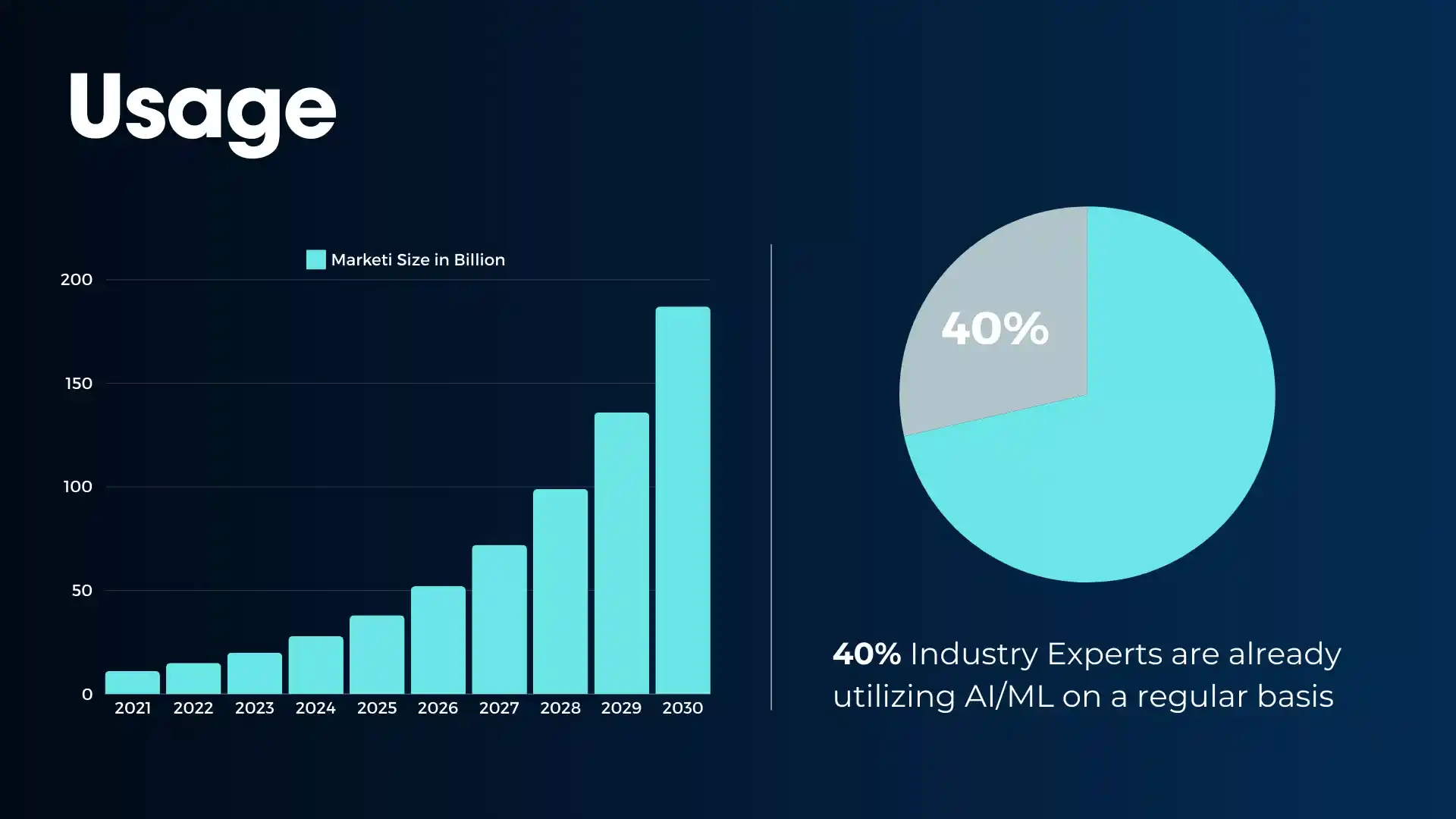
Benefits of Machine Learning in Healthcare
Improved Accuracy
Machine Learning can increase the accuracy of diagnoses and predictions, leading to better patient outcomes. These algorithms can be trained to recognize patterns in medical images, predict disease progression from medical records, and even generate novel drug candidates.
Cost Reduction
One of the significant potential benefits of Machine Learning in healthcare is cost reduction. Machine Learning algorithms can predict patient admission rates, helping hospitals manage staff resources more effectively. They can also help doctors prioritize patient needs, reducing the length of hospital stays and decreasing unnecessary testing.
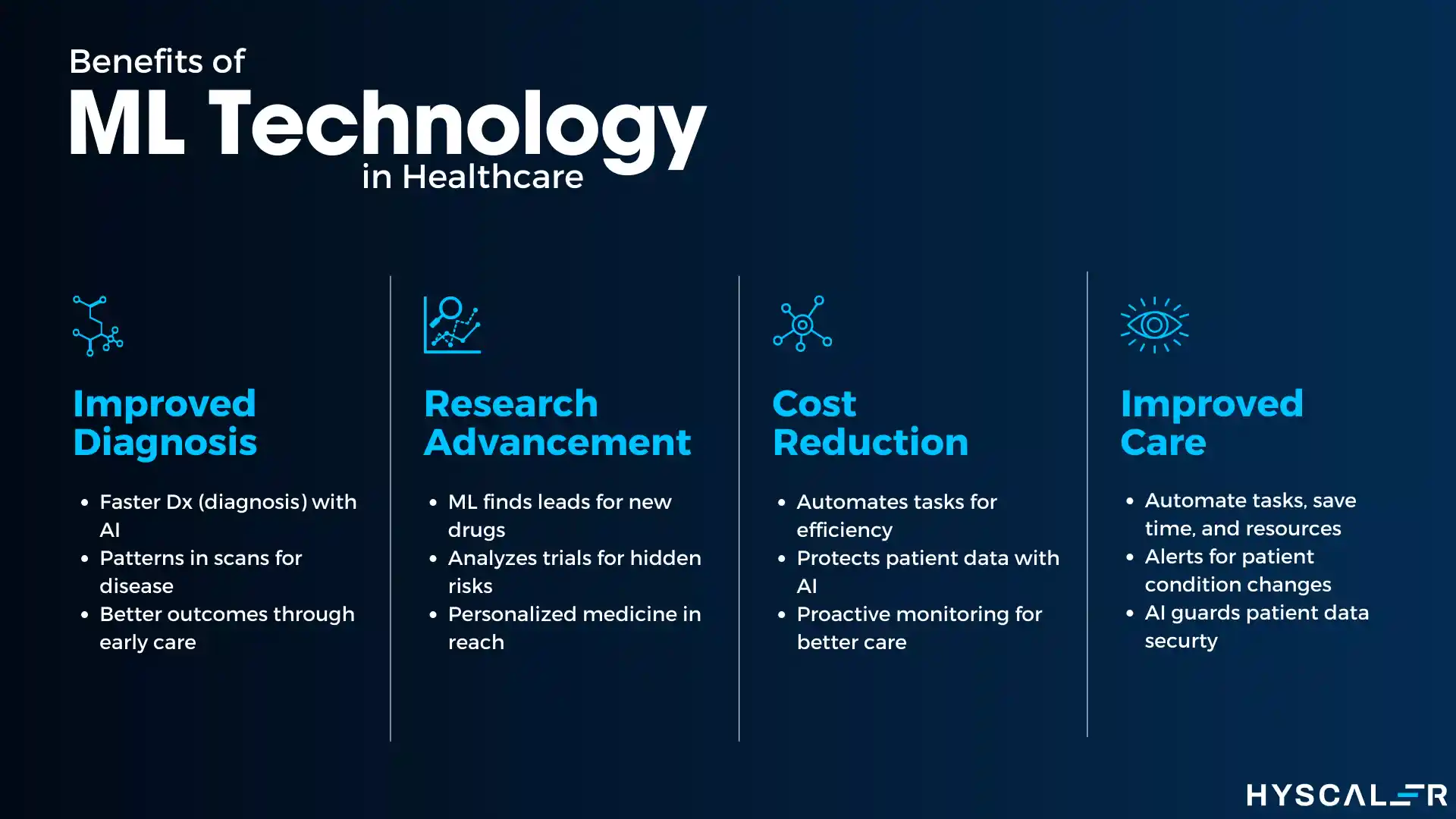
Personalized Care
Machine Learning can also enable personalized care. By analyzing a patient’s genetic makeup, lifestyle, and personal preferences, Machine Learning can create personalized treatment plans. This can lead to more effective treatments and better health outcomes for patients.
Predictive Treatment
Predictive analytics is one of the most promising benefits of Machine Learning in healthcare. These algorithms can predict disease progression and patient response to treatment. This can help doctors make proactive decisions about patient care, potentially catching complications before they become serious.
Application of Machine Learning in Healthcare
Disease Diagnosis and Predictive Analytics
Machine Learning is particularly good at recognizing patterns, which is deeply relevant to detecting diseases or predicting patient outcomes. Many companies are developing predictive algorithms to assist doctors in diagnosing diseases and predicting patient outcomes.
Drug Discovery and Development
Machine Learning can also be used in the discovery and development of new drugs. Pharmaceutical companies are using machine learning algorithms to shorten the drug discovery process. These algorithms can analyze molecular data and predict the effectiveness of potential drugs, significantly reducing the time and cost of drug discovery.
Personalized Treatment Plans
Personalized medicine, or precision medicine, is a medical model that separates patients into different groups—with medical decisions, practices, interventions, and/or products being tailored to the individual patient based on their predicted response or risk of disease.
Healthcare Operations and Management
From a healthcare provider’s perspective, Machine Learning can also be used to improve healthcare operations. It can optimize scheduling, reduce patient wait times, and improve patient flow. In addition, Machine Learning can be used to predict patient no-shows, helping to reduce wasted resources.
Limitations of ML in Healthcare
Privacy and Security of Data
One of the major concerns with using Machine Learning in healthcare is the privacy and security of patient data. There are strict regulations in place to protect patient information, and any breach could have serious consequences.
Lack of Interoperability
Another challenge is the lack of interoperability between different healthcare systems. This can make it difficult to integrate Machine Learning models into existing workflows.
Bias
Machine Learning models can be biased if the data they are trained on is not representative of the population they are intended to serve.
Skill Gap and Training
There is a significant skill gap in healthcare when it comes to understanding and implementing Machine Learning models. This can make it difficult to adopt these technologies in a clinical setting.
AI/ML Trends in Healthcare 2024
Remote Monitoring
The rise of wearable technology and telemedicine has made remote patient monitoring a practical reality. Machine Learning algorithms can scrutinize data from these devices, enabling them to predict health outcomes and provide personalized care based on the data.
Early Disease Detection
Machine Learning is playing a pivotal role in early disease detection by analyzing patient symptoms and medical history. This can lead to timely intervention and improved patient outcomes. For instance, researchers from MIT and MGH have developed an AI tool named Sybil, which can predict the likelihood of developing lung cancer within a year with an accuracy rate of 86% to 94%. Additionally, a health tech start-up won the Innov8 Talks pitch competition at the Florida International Medical Expo (FIME) 2023 for its AI solutions in early disease detection.
Personalized Treatment Plans
Machine Learning can formulate personalized treatment plans by analyzing a patient’s genetic makeup, lifestyle, and personal preferences. The integration of AI and personalized medicine is reshaping patient care by customizing healthcare to individual needs. This approach is particularly impactful in cancer treatment, where AI aids in diagnosing cancer, personalizing treatments, and monitoring survivors.
Streamlined Operations
Machine Learning can enhance healthcare operations by optimizing scheduling, reducing patient wait times, and improving patient flow. Hospitals are increasingly adopting AI to improve patient care and resource allocation. For example, the Cleveland Clinic has partnered with Palantir Technologies to launch a Virtual Command Center. In India, AI is streamlining hospital operations, automating administrative tasks, optimizing resource allocation, and enhancing decision-making through predictive analytics.
Data Security
With the increasing use of electronic health records, data security has become a major concern. Machine Learning can help detect and prevent data breaches by analyzing network traffic and identifying suspicious activity. The healthcare sector faces challenges with data breaches, which have reached an average cost of nearly $11 million. AI’s role is to balance healthcare advancement with the protection of sensitive information.
Healthcare Accessibility
AI is expected to play a vital role in improving healthcare accessibility. It can handle more patient requests such as providing basic medical information, answering questions, prescribing medications, and scheduling appointments. This can potentially reduce healthcare costs and improve access, outcomes, and efficiency.
These developments indicate a rapidly evolving landscape where AI is becoming an integral part of healthcare, offering solutions for early disease detection, personalized treatment, improved accessibility, operational efficiency, and enhanced data security. Is your organization fully equipped to leverage AI to stay at the forefront of healthcare in 2024? Experts in the field can help determine what’s necessary to get you there.
Conclusion
The use of Machine Learning in Healthcare has the potential to revolutionize the industry by improving patient care, reducing costs, and enhancing operational efficiency. However, there are also challenges that need to be addressed, such as data privacy, lack of interoperability, and the need for skilled professionals. As we move forward, it will be interesting to see how these technologies evolve and shape the future of healthcare.
FAQs
1. How is ML used in healthcare?
ML is used in healthcare to analyze large amounts of data and make predictions about patient health outcomes. This can help doctors make informed decisions about patient care.
2. What is ML in pharma?
In the pharmaceutical industry, ML is used to analyze molecular data and predict the effectiveness of potential drugs. This can accelerate the drug discovery process.
3. What is the concept of ML?
ML is a subset of artificial intelligence that uses statistical techniques to give computers the ability to learn from data without being explicitly programmed.
4. What are the benefits of AI ML in healthcare?
The benefits of AI and ML in healthcare include improved accuracy, cost reduction, personalized care, and predictive treatment.
5. What is ML in telemedicine?
In telemedicine, ML is used to analyze data from wearable devices and predict patient health outcomes. This can provide personalized care and early intervention.
6. What is ML in manufacturing?
Manufacturers are leveraging ML to solve specific business challenges such as tracing manufacturing defects, reducing waste, and optimizing production processes. This guide explores the impact of machine learning in manufacturing, highlighting key trends, applications, and implementation strategies for 2024.