The Impact of Machine Learning in the Manufacturing Industry: 2024 Guide
Table of Contents
Machine learning (ML) is transforming the manufacturing industry by significantly increasing efficiencies and creating new business opportunities. Manufacturers are leveraging ML to solve specific business challenges such as tracing manufacturing defects, reducing waste, and optimizing production processes. This guide explores the impact of machine learning in manufacturing, highlighting key trends, applications, and implementation strategies for 2024.
Machine Learning Trends in Manufacturing
According to McKinsey, 50% of companies that embrace AI over the next five years have the potential to double their cash flow through manufacturing efficiencies.
The global machine learning market size was valued at $19.20 billion in 2022 and is projected to grow to $225.91 billion by 2030, exhibiting a CAGR of 36.2%. This rapid growth underscores the importance of Machine Learning in the Manufacturing Industry.
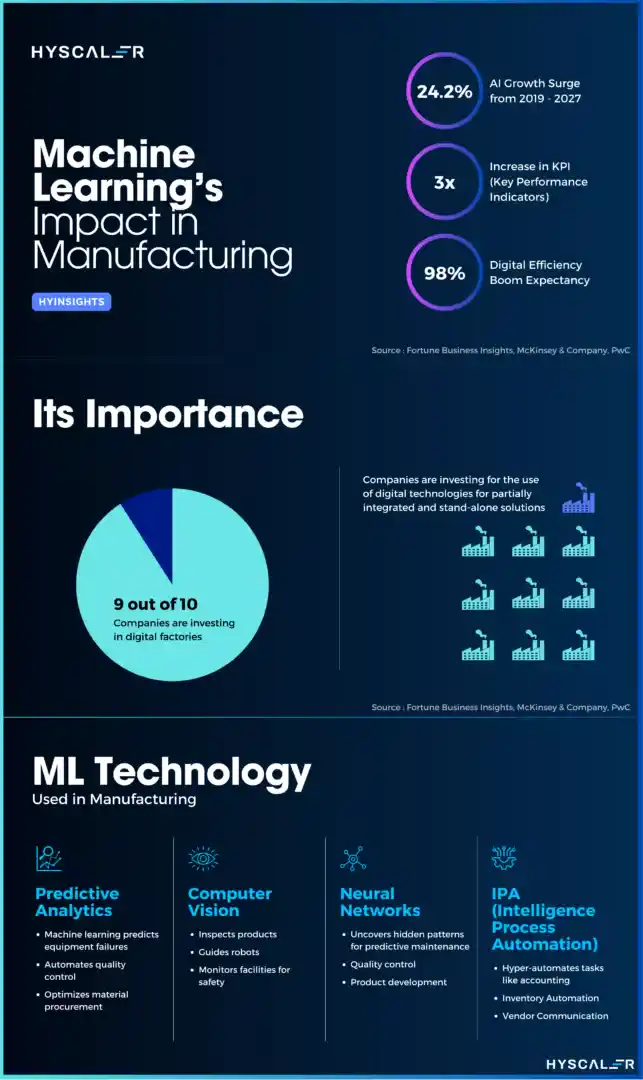
Importance of ML Adoption in Manufacturing
Adopting machine learning in manufacturing is crucial for staying competitive and innovative. ML technologies enable manufacturers to improve quality, enhance predictive maintenance, optimize production, and better forecast demand. By integrating ML, manufacturers can reduce operational costs, increase productivity, and deliver higher-quality products to market faster.
Applications of Machine Learning in Manufacturing
Error Detection
Machine learning algorithms can detect errors early in production, significantly reducing waste and ensuring higher product quality. By analyzing patterns and identifying anomalies, ML systems can pinpoint defects that might be missed by human inspectors.
Risk Management
ML models can predict potential risks in the manufacturing process by analyzing historical data and identifying trends. This proactive approach helps manufacturers mitigate risks before they escalate into significant issues, ensuring smoother operations and reducing downtime.
Aftermarket Services
Machine learning enhances aftermarket services by predicting when parts will need replacement and optimizing inventory management. This leads to improved customer satisfaction and reduced costs associated with overstocking or stockouts.
Customer Service
ML can help manufacturers understand customer needs and preferences better by analyzing customer feedback and usage patterns. This enables the development of more tailored products and services, enhancing customer satisfaction and loyalty.
Machine Learning Technologies Used in Manufacturing
Manufacturers use various ML technologies to drive innovation and efficiency, including:
- Predictive analytics
- Natural language processing (NLP)
- Computer vision
- Reinforcement learning
- Deep learning
Use Cases of Machine Learning in Manufacturing
Here is a list of use cases wherein, the use of ML in the manufacturing industry is followed:
Predictive Maintenance
ML algorithms predict equipment failures before they occur, allowing for timely maintenance and reducing unplanned downtime. This increases the lifespan of machinery and ensures uninterrupted production.
Example: General Electric uses predictive maintenance to monitor its aircraft engines and industrial machinery, significantly reducing maintenance costs and improving reliability.
Quality Control
ML enhances quality control by identifying defects and ensuring that products meet high standards. Automated visual inspection systems, powered by ML, offer higher accuracy and consistency compared to manual inspections.
Example: BMW uses machine learning to analyze images of car components, ensuring that each part meets stringent quality requirements.
Demand Forecasting
ML models analyze historical sales data and market trends to predict future demand accurately. This helps manufacturers optimize inventory levels, reduce excess stock, and meet customer demand more effectively.
Example: Amazon uses ML for demand forecasting to manage its vast inventory and ensure timely delivery to customers.
Contract Management
ML streamlines contract management by analyzing contract terms and conditions, identifying risks, and ensuring compliance. This reduces the administrative burden and helps manufacturers manage contracts more efficiently.
Example: IBM’s Watson uses ML to analyze contracts, identify key terms, and highlight potential risks, making contract management more efficient.
Product Development
ML accelerates product development by analyzing market trends and customer preferences. This enables manufacturers to design products that meet market demands and reduces the time to market.
Example: Procter & Gamble uses ML to analyze consumer feedback and market trends, speeding up the development of new products.
Production Optimization
ML optimizes production processes by identifying bottlenecks and recommending improvements. This leads to increased efficiency, reduced waste, and higher output.
Example: Tesla uses ML to optimize its production lines, ensuring maximum efficiency and minimal downtime.
Cybersecurity
ML enhances cybersecurity by detecting and responding to threats in real-time. This protects manufacturing systems from cyberattacks and ensures data integrity.
Example: Siemens uses ML to monitor network traffic and detect unusual patterns that may indicate a cyberattack.
Robotics
ML-powered robots improve automation by performing complex tasks with high precision. This increases production speed and consistency, reducing the reliance on manual labor.
Example: Fanuc uses ML in manufacturing its robotic arms to enhance precision and adaptability in manufacturing processes.
Order Management
ML streamlines order management by predicting demand, optimizing inventory, and improving order fulfillment. This ensures timely delivery and enhances customer satisfaction.
Example: Walmart uses ML to manage its supply chain and ensure that products are always in stock and delivered on time.
Digital Twin
A digital twin is a virtual replica of a physical asset or process. ML enhances digital twins by providing real-time insights and predictive analytics, enabling manufacturers to optimize operations and improve decision-making.
Example: Rolls-Royce uses digital twins to monitor and optimize the performance of its jet engines.
When to Adopt Machine Learning
Manufacturers should consider adopting ML when facing challenges such as unsafe working environments, inefficient equipment maintenance, inaccurate demand forecasting, and limited design options. ML can address these issues by improving safety, optimizing maintenance schedules, enhancing forecasting accuracy, and expanding design capabilities.
Indicators for ML Adoption:
- Unsafe Working Environments: Robots embedded with advanced computer vision systems can differentiate between objects and people, significantly increasing on-site employee safety.
- Inefficient Equipment Maintenance: Assisted by IoT sensors and machine learning, technicians can save time and resources by conducting maintenance checks only when equipment has a high probability of failure.
- Inefficient Visual Inspection: ML-enabled visual inspection tools are not thrown off by environmental changes and can continuously learn, improving visual inspection precision.
- Limited Design Options: ML-based generative design systems can provide a broader range of design options, exceeding human outputs.
- Inaccurate Demand Forecasting: ML systems process enormous amounts of data in real time, making demand predictions more accurate.
Step-by-Step Implementation Guide
Define
Clearly define the problem you want to solve with ML and set measurable goals. This step involves understanding the business problem and determining how ML can provide a solution.
Gather Data
Collect relevant data from various sources, ensuring it is comprehensive and high-quality. The success of an ML model depends heavily on the quality and quantity of data available.
Data Cleaning and Formatting
Clean and format the data to ensure it is suitable for analysis. This step involves removing inconsistencies, handling missing values, and ensuring the data is in a usable format.
Data Visualization
Visualize the data to identify patterns and insights that can inform your ML model. Data visualization helps in understanding the underlying trends and relationships in the data.
Model Training
Train your ML model using the prepared data. Choose the appropriate algorithms and tune the parameters for optimal performance. This step involves selecting the right model and training it to learn from the data.
Model Validation and Deployment
Validate the model to ensure it performs well on new data. Deploy the model into your production environment for real-time predictions. Model validation ensures that the model generalizes well to new, unseen data.
Continuous Model Retraining
Regularly retrain the model with new data to maintain accuracy and adapt to changing conditions. Continuous retraining ensures that the model remains effective over time as new data becomes available.
Conclusion
Machine learning has revolutionized various industries such as healthcare, logistics, automobiles, and others. Machine learning is revolutionizing the manufacturing e-commerce industry by enhancing efficiency, quality, and innovation. By adopting Machine Learning in manufacturing, manufacturers can solve complex problems, optimize processes, and stay competitive in a rapidly evolving market. Implementing ML in manufacturing requires a strategic approach, but the benefits far outweigh the challenges, making it a critical investment for the future.
Featured Insights
Integrating Real-Time Weather Data into Your Travel and Navigation App
The Future is Now: AI and ML Technologies Reshaping Healthcare
Introduction to Digital Twin Technology in Manufacturing with Unprecedented Efficiency and Innovation
Adobe’s Firefly AI: New Tools for Photoshop and Illustrator
Introducing Meta Imagine Me: Your Digital Doppelganger
AI in Gaming is Transforming the Industry