Table of Contents
In 2025, the connection between IoT (Internet of Things) and data analytics is changing business intelligence (BI) in a big way. Companies across all industries are moving away from old data and static dashboards. They now use real-time, predictive tools to make quicker and smarter decisions.
This change comes from billions of connected devices that send out data nonstop. Powerful analytics then turn this data into instant insights.
What Is IoT?
IoT stands for the Internet of Things. It means everyday machines like cars, refrigerators, or factory machines can connect to the internet and send data.
What Is Data Analytics?
Data analytics is how we study that data to learn something useful. We use it to spot trends, solve problems, or make predictions.
What is IoT Data Analytics?
how we study that data to learn something useful. We use it to spot trends, solve problems, or make predictions.
What is IoT Data Analytics?
IoT data analytics means gathering and studying data from devices that connect to the internet. This includes sensors, wearables, machines, and vehicles. These devices track everything from temperature to motion to user interactions.
But this raw data alone isn’t helpful until it’s processed and analyzed. That’s where AI-driven analytics platforms come in. They:
- Clean and structure the data
- Analyze patterns and trends
- Offer predictive and prescriptive insights
- Enable automation in real-time
How Are They Working Together?
- Faster Decisions: Sensors send live data. Businesses don’t need to wait for reports they can act now.
- Saving Money: Machines tell when they need repairs, so companies fix problems early.
- Smarter Customer Service: Companies know what customers like and give better suggestions.
- Better Planning: With data from devices, businesses can see what’s coming and get ready.
Types Of Data Analytics In IOT
IoT analytics can be categorized into four key types, depending on business needs and the analytical goals:
- Descriptive IoT Analytics
- Diagnostic IoT Analytics
- Predictive IoT Analytics
- Prescriptive IoT Analytics
1. Descriptive IoT Analytics
Answers: What happened?
- Focuses on historical data collected by IoT devices
- Helps track performance, workflow, inventory, sales, and revenue
- Example: Reviewing past sensor data to monitor machine uptime
2. Diagnostic IoT Analytics
Answers: Why did it happen?
- Investigate causes behind trends or anomalies
- Helps identify inefficiencies or failures in IoT-connected systems
- Example: Analyzing underperformance of a factory sensor to find root causes
3. Predictive IoT Analytics
Answers: What is likely to happen?
- Uses AI/ML models trained on historical data
- Detects patterns, performs trend analysis, and forecasts outcomes
- Example: Predicting engine failure in a vehicle based on sensor data
4. Prescriptive IoT Analytics
Answers: What action should be taken?
- Suggests best decisions or actions based on past, present, and predicted data
- Combines insights from all other types of analytics
- Example: Recommending maintenance scheduling or operational changes for cost savings
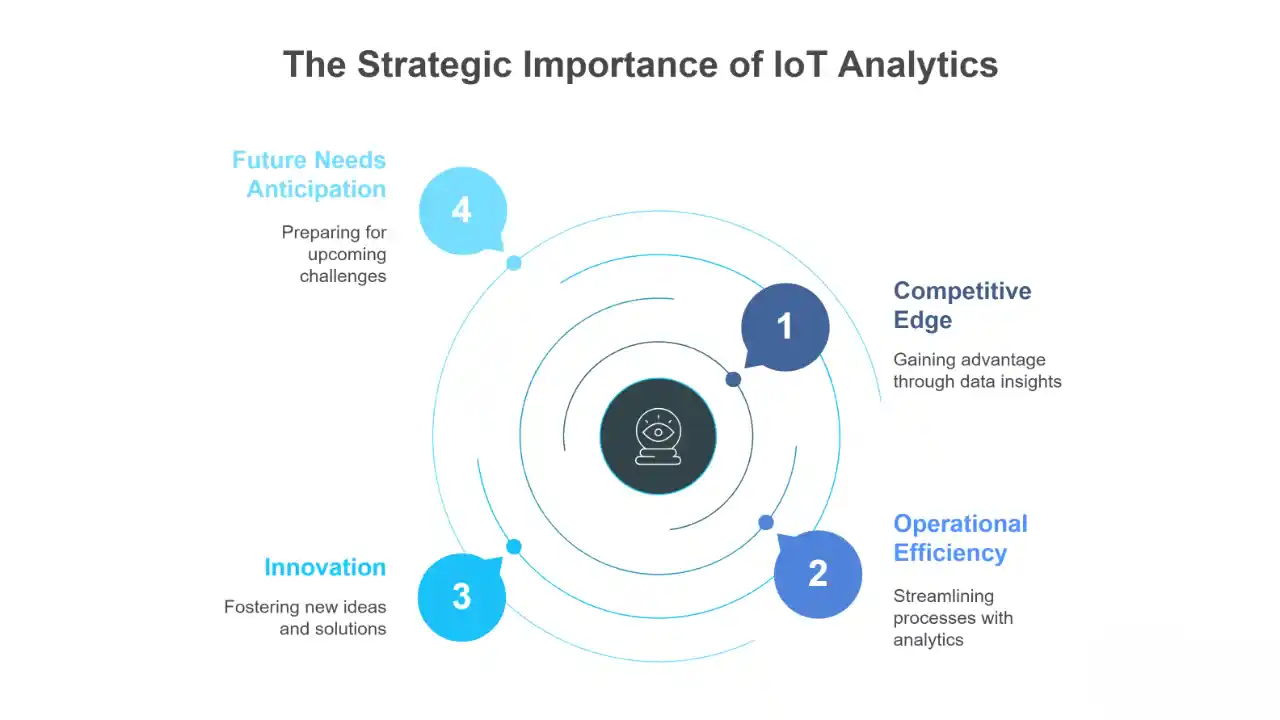
How IoT is Transforming Business Intelligence
Below are the five major ways IoT data analytics is redefining BI in 2025, along with real-world use cases to bring the changes to life.
1. Real-Time Decision-Making: Speed is the New Competitive Edge
Traditionally, BI tools pulled from historical databases and required manual report generation. IoT-connected systems send real-time data to analytics dashboards. This lets businesses act right away.
Real-World Example: DHL’s Smart Warehouses
DHL uses IoT sensors in their warehouses to track package movement, temperature, and staff efficiency. The system alerts managers when delivery delays occur or warehouse zones get too crowded. This allows instant re-routing of tasks, saving time and improving safety.
Result:
- 25% faster delivery times
- Real-time labor optimization
- Reduced inventory shrinkage
2. Predictive Intelligence: Seeing Problems Before They Happen
In 2025, businesses aren’t just reacting to problems they’re anticipating them. Machine learning algorithms analyse IoT data analytics. They predict equipment failure, demand spikes, or customer loss.
Real-World Example: GE Aviation
GE uses IoT sensors in airplane engines to monitor vibration, pressure, and fuel usage. Their analytics platform predicts engine failures ahead of time. This helps airlines schedule maintenance only when it’s needed.
Result:
- 30% reduction in unscheduled downtime
- $12B saved in predictive maintenance across clients
3. Personalized Customer Experiences: Real-Time Customization
Companies are now using IoT data analytics to offer customers hyper-personalized services. Data from smart devices, cars, and mobile apps is analyzed to tailor offerings in real time.
Real-World Example: Starbucks and IoT Beacons
Starbucks uses IoT-enabled beacons in its stores. When a customer with the mobile app walks in, the store knows their favorite drink and offers a customized discount via push notification. This drives repeat visits and loyalty.
Result:
- 15% boost in same-store sales
- 10M+ app users receive real-time offers
4. Operational Efficiency: Automating for Precision
IoT analytics allows organizations to improve internal operations automatically. Businesses are using connected sensors and AI. They aim to cut waste and boost quality in areas like energy use and manufacturing output.
Real-World Example: Siemens Digital Factory
Siemens uses IoT sensors on production lines in its electronics plants. The data is analyzed to identify process inefficiencies or errors in real time. Machines adjust their operations automatically, minimizing human involvement.
Result:
- 99.99885% manufacturing accuracy
- 75% fewer production errors
5. Autonomous BI: The Rise of Self-Optimizing Systems
In 2025, business intelligence tools don’t just offer insights they take automated action. AI systems now analyze IoT data Analytics and trigger workflows or adjustments without waiting for human approval.
Real-World Example: Tesla’s Autonomous Fleet Updates
Tesla’s vehicles constantly collect sensor data from cameras, radars, and GPS. No service center visits required.
Result:
- Faster feature rollouts
- Improved road safety
- Massive cost savings
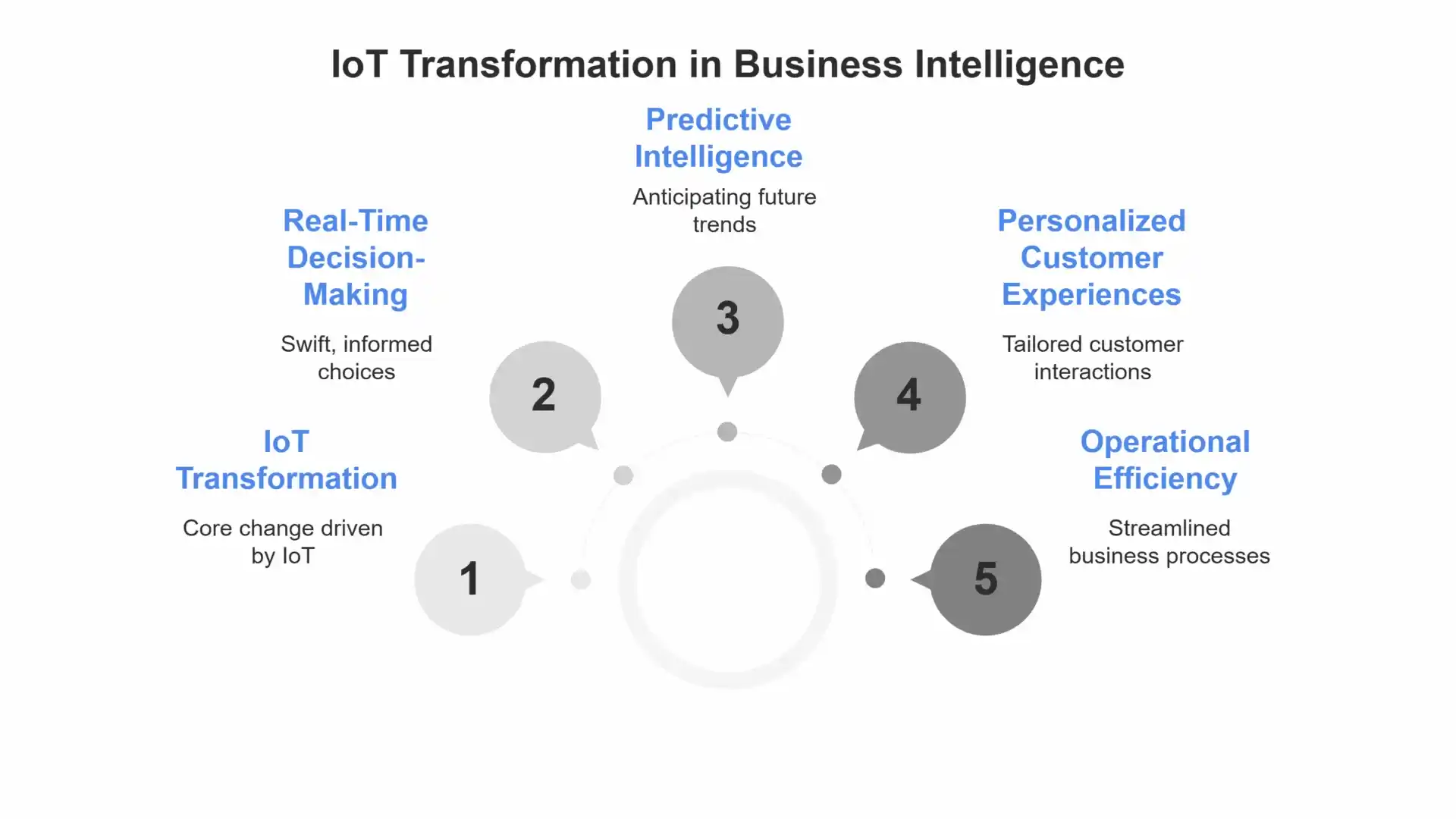
Key Technologies Enabling the Change
Several advanced technologies are working behind the scenes to make this revolution possible:
Edge Computing
Processes data closer to where it’s generated (e.g., in a device or sensor), reducing latency and network load.
AI & Machine Learning
Find patterns, predict outcomes, and make real-time decisions based on huge volumes of sensor data.
5G Connectivity
Provides the bandwidth and speed needed to connect thousands of devices with almost zero delay.
Cloud & Hybrid Storage
Helps businesses scale their analytics infrastructure across multiple locations securely.
How HyScaler Is Driving This Transformation
HyScaler, a global digital engineering and technology consulting firm, is playing a key role in helping organizations build intelligent IoT ecosystems that power business intelligence in real time.
Key Solutions Offered by HyScaler:
- Custom IoT Platform Development: Enabling real-time data collection and integration from devices
- AI-Powered Analytics Dashboards: Turning complex sensor data into useful business insights
- Predictive Maintenance Solutions: Minimizing operational downtime using machine learning
- Edge and Cloud Integration: Ensuring data flow is fast, secure, and scalable
Real Impact:
HyScaler helped a logistics client reduce warehouse costs by 28% using a sensor-based temperature monitoring and auto-alert system.
Industries Being Transformed in 2025
Manufacturing
- IoT-enabled equipment sends performance data
- Predictive analytics reduces breakdowns
- Production is optimized for demand
Healthcare
- Wearables and medical devices track real-time vitals
- Alerts notify doctors of emergencies before patients even arrive
- Hospital inventory managed with smart tracking systems
Logistics
- GPS sensors track delivery fleets
- Traffic and weather data optimize routes in real time
- Lost inventory is a thing of the past
Retail
- Smart shelves track product movement
- Personalized promotions based on in-store behavior
- Dynamic pricing based on demand and availability
Challenges to Overcome
While promising, IoT data analytics still faces some roadblocks:
- Data Privacy Concerns: Companies must ensure they are collecting and using data ethically.
- Integration Complexity: Linking legacy systems with IoT platforms requires careful planning.
- Security Risks: More connected devices mean more potential vulnerabilities.
- Data Overload: Without the right filters and tools, too much data can slow down decisions.
The Road Ahead: What to Expect Next
By 2030, experts expect:
- Over 75 billion IoT devices worldwide
- 90% of enterprise data processed in real time
- AI-based analytics to replace manual BI processes entirely
Companies that adapt early will have a huge advantage. They’ll be able to predict customer needs, stop problems before they start, and run leaner, smarter operations.
Conclusion: Smarter Devices, Smarter Decisions
IoT data analytics is no longer a futuristic concept it’s here, it’s growing, and it’s completely reshaping how business intelligence works in 2025.
Thanks to AI, edge computing, and real-time processing, companies now have the power to make smarter decisions at every moment. And with partners like HyScaler, businesses have access to the tools and expertise needed to thrive in this new era.
The future of BI isn’t just about data. It’s about knowing what to do with it right now.
FAQ
What is IoT and how does it relate to business intelligence?
IoT (Internet of Things) connects physical devices to the internet, enabling them to send and receive data. This data, when analyzed, powers real-time business insights and smarter decision-making.
How is data collected from IoT devices?
IoT devices have built-in sensors that gather data like temperature, movement, location, or how something is used. This data is then sent to analytics systems where it’s processed and turned into useful insights.
What is the role of AI in IoT data analytics?
AI helps clean, analyze, and interpret large volumes of IoT data analytics. It identifies patterns, predicts outcomes, and enables automated, intelligent responses in real-time.
How does IoT data analytics improve real-time decision-making?
By giving instant insights from live sensor data, businesses can react right away instead of waiting for old-style reports.
What industries benefit most from IoT data analytics?
Industries like manufacturing, healthcare, logistics, and retail get better results by working more efficiently, spotting problems early, and giving customers a better experience.