Table of Contents
Overview of ZenML’s Mission
ZenML is striving to become the connective tissue uniting the myriad of open-source AI tools available. This unique open-source framework is designed to facilitate the creation of pipelines, promoting collaboration among data scientists, ML engineers, and platform engineers in crafting innovative AI models.
What sets ZenML apart is its capability to offer businesses the autonomy to construct bespoke models tailored to their specific needs, even if they might not match the complexity of solutions like GPT 4. This initiative could also decrease corporate reliance on API providers, including prominent names such as OpenAI and Anthropic.
Louis Coppey from Point Nine Capital expressed that as the initial surge of enthusiasm for exclusive API solutions like OpenAI subsides, it could provide an avenue for organizations to craft their custom stacks.
Financial Backing and Development
ZenML recently secured additional funding in an extended seed round, supported by Point Nine and previous investor Crane. Since its establishment in Munich, the company has garnered a total of $6.4 million in investments.
Technical Foundation and Philosophy
The inception of ZenML stemmed from the combined efforts of founders Adam Probst and Hamza Tahir, who previously collaborated on crafting ML pipelines tailored for distinct sectors. Probst shared that their experience in repeatedly developing machine learning solutions inspired them to devise a flexible system that could cater to diverse conditions and client needs, culminating in the creation of ZenML.
ZenML is also paving the way for budding engineers in the machine learning domain. The framework’s philosophy, termed MLOps by the ZenML team, draws parallels to the DevOps approach but is uniquely tailored to ML.
As Probst explains, ZenML seamlessly integrates various open source tools, catering to diverse stages in the ML pipeline construction process, all while leveraging the capabilities of major platforms like AWS and Google, and also in-house solutions.
ZenML’s core revolves around its pipeline-centric architecture. These pipelines can be run locally or deployed via renowned open-source platforms like Airflow or Kubeflow. It’s designed for compatibility with managed cloud services, encompassing EC2, Vertex Pipelines, Sagemaker, and more, while also interfacing with prevalent ML tools, including Hugging Face, MLflow, TensorFlow, and PyTorch.
Hamza Tahir, the CTO of ZenML, elaborates that their offering provides a unified experience that spans multiple vendors and cloud platforms, emphasizing its role in ensuring traceability and transparency in ML processes.
Originally introduced on GitHub as an open-source initiative, ZenML has accumulated significant recognition with over 3,000 stars on the platform. Moreover, the company has expanded its reach with a cloud variant, with plans to introduce continuous integration and deployment (CI/CD) capabilities shortly.
ZenML has garnered attention in various sectors, ranging from industrial applications to e-commerce recommendation engines and medical image recognition.
Challenges in Harnessing the Full Potential of MLOps Platforms
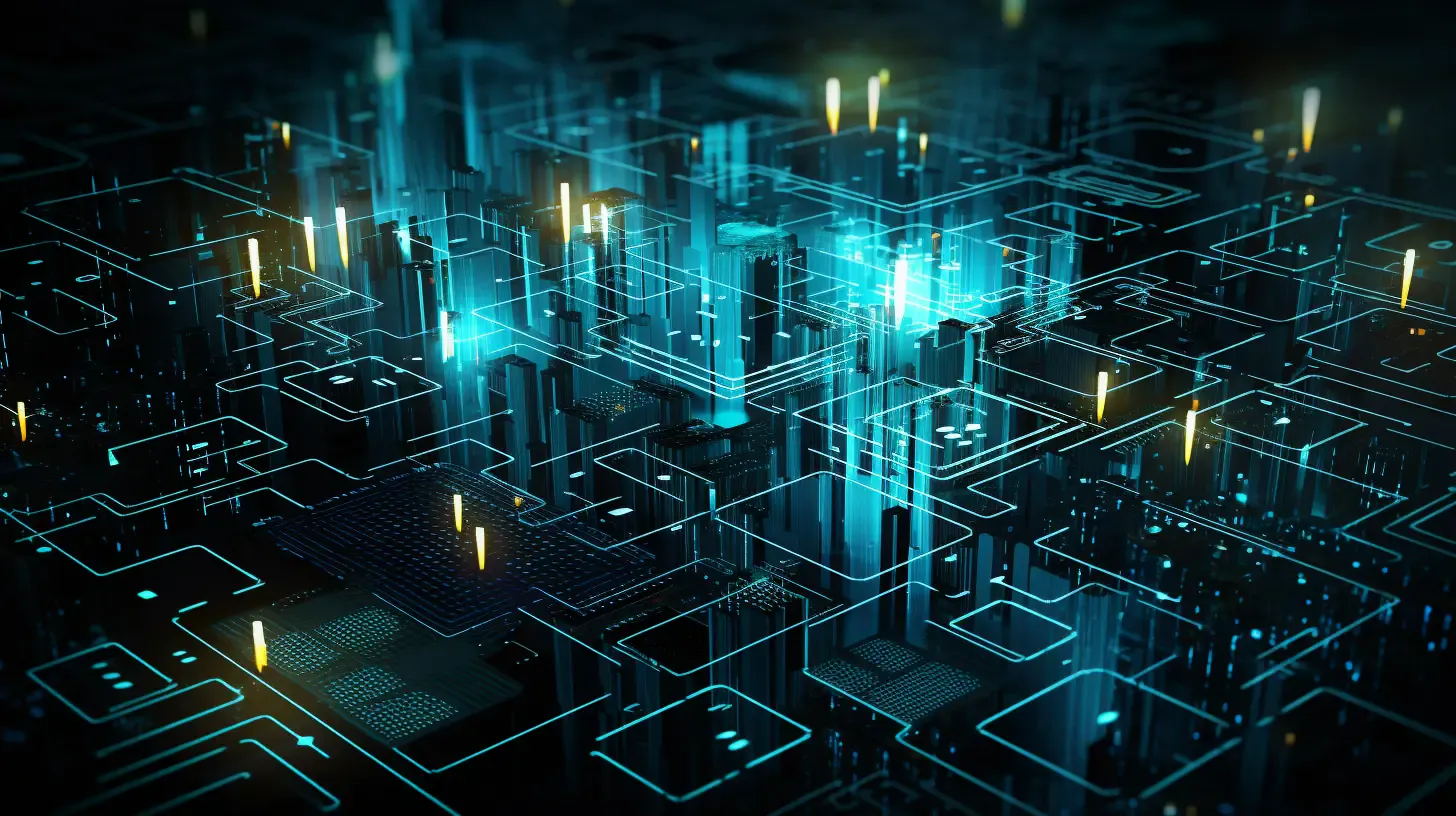
Everyone dreams of harnessing the power of their own ChatGPT, but crafting a universal MLOps platform that effectively caters to diverse infrastructures, tools, and individual needs remains a significant challenge.
Despite 90% of businesses expressing a desire to implement machine learning internally, the surge in GenAI popularity has highlighted how crucial applied machine learning has become as a strategic advantage. Still, 91% of firms are yet to fully establish their ML infrastructure.
A vast majority grapple with the intricacies of prolonged PoCs for specialized tools and feel tethered by the commitments to high-cost, end-to-end cloud solutions. Furthermore, while 92% of enterprises show keen interest in leveraging an MLOps platform, they face persistent integration issues. Over half of these businesses encounter challenges in seamlessly integrating various tools into a cohesive, unified workflow, emphasizing the complexities in the evolving ML landscape.
Shift Towards Bespoke AI Models
As ZenML charts its path in the AI arena, it’s essential to contextualize its potential role within the dynamically evolving AI ecosystem. At present, numerous firms are integrating AI capabilities by leveraging OpenAI’s API. This has manifested in various products, from features that succinctly summarize extensive text to automated responses designed for customer service engagements.
Adam Probst believes that while entities like OpenAI will persist, a significant portion of the market will gravitate towards bespoke solutions tailored to their needs.
However, the prevailing approach to using these APIs has its limitations. They can be too intricate and carry a hefty price tag.
“The large language models like OpenAI, developed in stringent environments, are conceived for a broader spectrum of applications. They are excessively refined and exorbitantly priced for niche use cases.”
Adam Probst
This underlines a crucial market gap that ZenML aims to address, emphasizing the significance of tailored, industry-specific AI models.