Table of Contents
In the ever-evolving landscape of scientific discovery, researchers continually seek to push the boundaries of knowledge. However, even the most brilliant minds can sometimes overlook crucial insights due to the sheer volume and complexity of data available. This is where Artificial Intelligence (AI) steps in as a transformative force, offering a fresh perspective and the potential to unveil hidden research blind spots.
The Research Landscape: Vast and Complex
Research, across all disciplines, relies on the formulation of hypotheses. These hypotheses act as guiding lights, leading researchers on a journey of exploration and experimentation. While human creativity and intuition have fueled countless breakthroughs, they are not without limitations.
In the age of information overload, researchers are confronted with an abundance of data, publications, and variables to consider. This complexity can inadvertently obscure crucial connections and insights. Despite rigorous methodologies and expertise, research blind spots can persist, leaving questions unanswered and opportunities unexplored.
AI in Research as a Hypothesis Generator
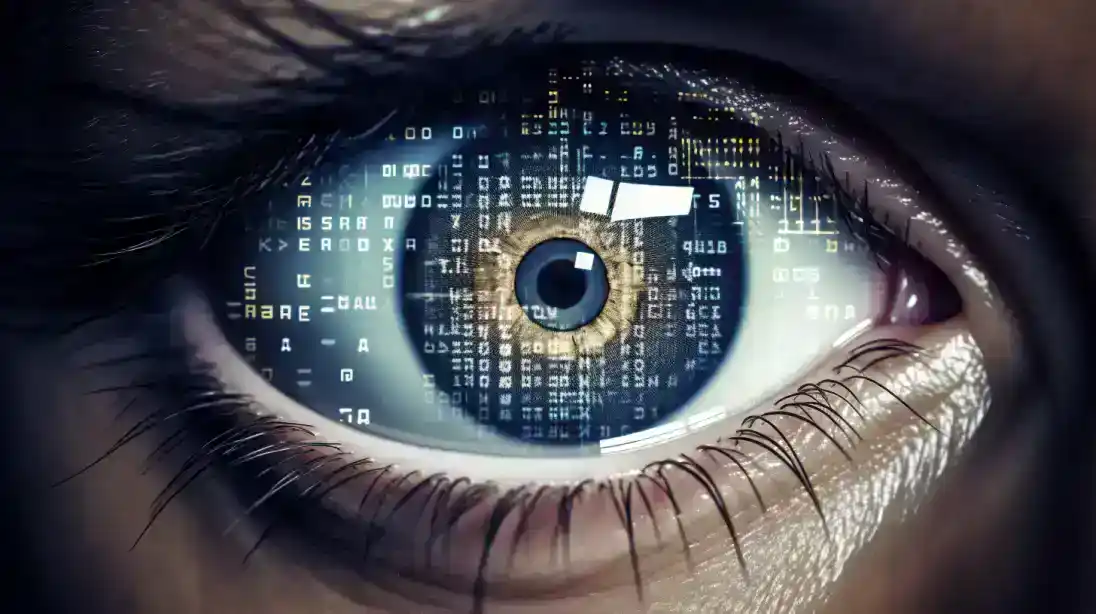
AI, particularly machine learning algorithms, has emerged as a powerful ally in the realm of research. By ingesting vast datasets, AI systems can identify patterns, correlations, and anomalies that may elude human perception. This ability positions AI as an invaluable hypothesis generator, capable of suggesting research directions and hypotheses that researchers may not have considered.
The AI Advantage of AI in Research
1. Data-driven Insights: AI leverages data as its primary resource. It can analyze vast datasets from diverse sources, identifying trends and patterns that human researchers may overlook. Doing so helps formulate hypotheses based on evidence rather than intuition alone.
2. Cross-disciplinary Connections: AI transcends the boundaries of individual disciplines. It can draw connections between seemingly unrelated fields, paving the way for interdisciplinary research and the discovery of innovative hypotheses.
3. Continuous Learning: AI systems learn and adapt over time. As they process more data and gather feedback, they become increasingly adept at generating hypotheses that align with emerging research trends and knowledge gaps.
4. Accelerating Discovery: AI’s ability to swiftly process and analyze data accelerates the research process. It not only identifies existing hypotheses but also proposes new ones, streamlining the path to discovery.
5. Reducing Bias: AI approaches research with objectivity, minimizing the influence of human biases. This impartiality can lead to the formulation of hypotheses that challenge conventional thinking, fostering innovative breakthroughs.
6. Exploring the Unexplored: AI can explore uncharted territories within a research domain. By venturing into the unknown, it uncovers novel hypotheses that might have remained hidden within the vast realm of data.
7. Predictive Insights: Through predictive analytics, AI can anticipate future research directions and suggest hypotheses that align with upcoming trends, enabling researchers to stay at the forefront of their fields.
8. Hypothesis Validation: AI not only generates hypotheses but can also assist in their validation. By conducting simulations, experiments, and predictive modeling, AI can test the viability of hypotheses, saving time and resources.
9. Iterative Improvement: AI’s learning capabilities extend to refining hypotheses iteratively. As it collects more data and refines its algorithms, AI becomes more precise in proposing hypotheses that align with evolving research landscapes.
10. Collaborative Potential: AI fosters collaboration by facilitating knowledge-sharing among researchers. It can identify shared interests and connect researchers from different backgrounds, leading to the co-creation of hypotheses and interdisciplinary breakthroughs.
Incorporating AI in research endeavors introduces an array of advantages that extend beyond hypothesis generation. Its capacity to analyze data comprehensively, make cross-disciplinary connections, and adapt to evolving research trends positions AI as an indispensable partner in the pursuit of knowledge and innovation. As researchers embrace these AI-driven enhancements, the future of research appears promising, marked by a deeper understanding of complex phenomena and the discovery of solutions to some of humanity’s most pressing challenges.
AI in Action
One notable example of the impact that ai in research brings on hypothesis generation, is from a recent study published in Nature. Researchers used AI algorithms to analyze vast repositories of scientific literature. The AI system identified research gaps and proposed hypotheses that could lead to breakthroughs in understanding complex diseases. This instance underscores AI’s potential to complement human expertise and drive scientific progress.
The Collaborative Future
It’s essential to recognize that AI doesn’t replace researchers but augments their capabilities. The future of research will likely be a collaborative endeavor, with AI and human researchers working hand in hand. AI can tackle the formidable task of processing and analyzing data, allowing human researchers to focus on experimental design, interpretation, and the pursuit of novel discoveries.
As we embrace AI’s role in hypothesis generation, the research landscape is poised to become more dynamic and productive. With AI in research, we have the opportunity to shed light on previously unnoticed research blind spots, unveiling insights that could transform the world of science and knowledge. As AI in research continues to evolve, it’s clear that the future holds exciting possibilities for discovery and innovation.