Table of Contents
Green AI is the key to harnessing the power of artificial intelligence while minimizing its environmental impact. Think of a tirelessly running machine, solving complex problems, translating languages, diagnosing diseases, and even creating art. Now think of the energy behind this machine in the form of servers that hum, the screens that glow, electricity flowing, and data transmitted through invisible forces. AI is sometimes conceptualized as a bridge for mankind to their future, and therefore very often paid for in energy. With the world trying to grow greener, therein lies the pertinent question: How do you drive innovation without further harming the Earth?
This is where Green AI enters the picture: the responsible asking of innovation to involve smarter and leaner AI systems in terms of performance and energy consumption. Green AI is not just an academic proposition, it is a necessity in a warming climate and surging energy demand.
The Weight of Intelligence: AI’s Carbon Problem
To understand Green AI, it is here that we should broach the question of the environment and the costs involved with present trajectories. Training a large AI model such as GPT-3 by OpenAI consumed over 1,287 MW/h of electricity, permitting one comparison: this is enough electricity to power 120 average U.S. homes for entirely one year. The emissions were around 552 tons of CO2 equivalent, an amount comparable to what would have been emitted by five round trips from New York to London.
Data centers, which are the backbones of AI, already consume roughly around 1% of global electricity, a figure that is possibly bound to increase as AI-enabled services gather steam in the global market. The alien hunger for energy lies in the need for deep learning models if AI is to traverse such gigantic datasets and exercise its computational power potential. Each suggestion made by a Netflix advisory or a query put through a virtual assistant leads to thousands of petite notable operations, all nutritionally accounted for in this dispassionate energy tide.
Shrinking the Giant: The Principles of Green AI
Green AI brings about efficiency without compromise. It’s a philosophy that aims to effect a merger of innovation and sustainability by ensuring the worthiness of every watt expended on computation in terms of its value to human progress. This approach may therefore be seen in two ways:
- Algorithmic Efficiency
Traditional AI models are akin to SUVs; very powerful but gas-guzzlers. Green AI has, thus, set about building electric vehicles instead. Model pruning reduces the number of neurons within a neural network that is vested in any one task, thereby creating smaller models with retention of their precision. For instance, researchers at MIT demonstrated that model pruning could reduce computational costs by up to 90% while retaining performance levels similar to the larger model.
Another method is knowledge distillation. This means that a smaller model learns from a larger one, effectively appropriating that latter’s intelligence, thus dispensing with the need for retraining it on huge datasets.
- Energy-Conscious Hardware
AI hardware has moved towards a state of prioritizing energy efficiency. Today’s GPUs and TPUs, designed for AI workloads, include special-purpose cores that perform computations far faster and consume less energy. NVIDIA, a leader in the space, has recently announced that its Ampere architecture GPUs provide 2x the energy efficiency of their predecessors.
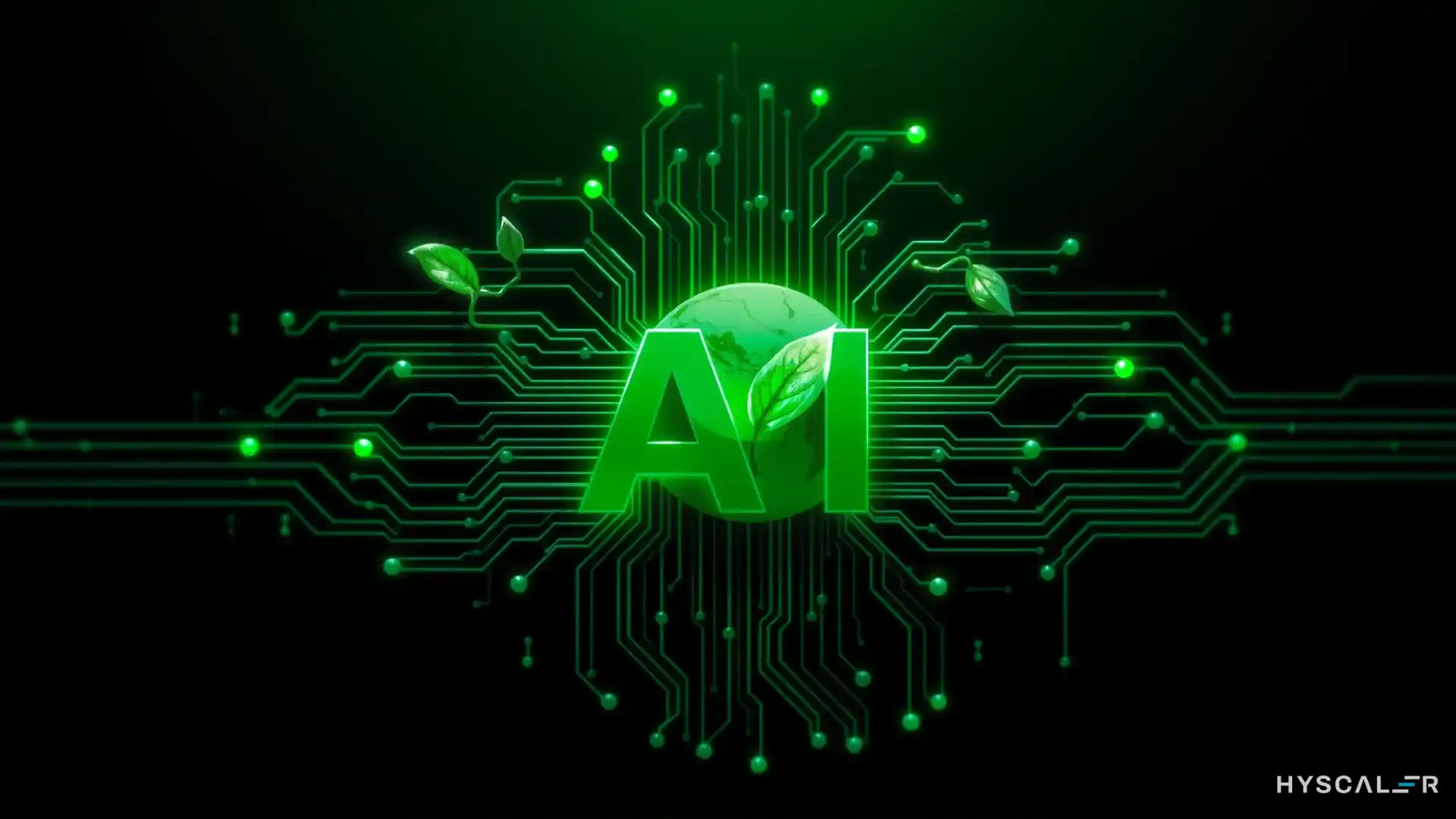
Where the Cloud Meets the Sun
One often-unnoticed angle is how data centers operate from the perspective of electricity consumption. The largest cloud service providers, like Google, Amazon, and Microsoft, are on a continued effort to ensure their plants run on renewable energy. Google claims to have been carbon-neutral since 2007, and by the decade’s end, aims at total operational autonomy on clean energy.
Among the standouts is Google’s DeepMind, which has managed to apply AI to cooling system optimization and resulted in a mere 40% reduction in energy consumption for cooling alone-here is clear proof of AI’s potential to solve its energy problems.
Meanwhile, companies are tinkering around by experimenting with new cooling methods that include immersion of servers in non-conductive liquids or transfer of heat in naturally cold areas (like the Arctic). The slight changes help shrink the carbon footprint for AI operations strikingly.
Moreover, evermore companies are making the move to liquid cooling systems, in which the servers are submerged in a non-conductive fluid that dissipates heat way more effectively than air. This method vastly reduces the need for conventional air conditioning which consumes a relatively large portion of electrical energy in data centers. Early trials have demonstrated this technology’s notable improvement in cooling efficiency, with estimates suggesting that liquid cooling may realize energy savings of up to 40% when measured against air-based systems.
From Code to Conservation: The Power of Green AI
Increased Energy Efficiency
Green AI often makes AI models and systems different forms of energy consumption-optimizing their power consumption through approaches such as model compression, pruning, and quantization-reducing the amount of computational power required for training and running models, thereby reducing the total energy footprint of AI technologies. For instance, Google’s AI-powered cooling system increases data center operational efficiency by optimizing cooling processes.
Enhanced AI Algorithm Sustainability
This refers to Green AI, designed not only for its efficiency but also for being energy-friendly. By optimizing the complexity of AI algorithms, firms reduce their computing power, resulting in lower energy consumption and the least possible devastation caused to the surroundings.
AI and Environmental Challenges
Green AI makes significant contributions to solving global environmental problems, as AI uses the underlying models to optimize energy use, detect climate changes, predict impending natural disasters, and adopt sustainable agricultural practices to enhance transport and waste management. It can aid in spotting inefficiencies in operations, maximizing the allocation of resources, and engineering solutions to achieve lower carbon footprints.
Renewable Energy Optimization through AI
Green AI’s optimization extends to the integration of renewable energy. AI schedules the energy demand and optimizes the production from renewable energy resources. AI also contributes to enhancing storage systems for surplus energy while improving the reliability and scalability of renewable sources.
Lowering Carbon Emissions from AI Operations
With the use of renewable energy sources for feedstock powering AI data centers and energy-efficient cooling systems, Green AI will reduce the carbon footprint of AI operations. Microsoft and Google are already committing to having data centers run on clean energy to minimize the environmentally risky footprint of AI-related activity.
Unlocking Progress: Overcoming Challenges with Creative Solutions
Immersion Cooling: High Initial Investment
Challenge: Immersion cooling systems in which servers are immersed in non-conductive liquids generate substantial energy reductions. Nevertheless, the purchase cost of these special systems is high upfront. An IEEE (2018) study estimates that the cost of moving to immersion cooling can be 3 times greater than the current air cooling systems.
Solution: Immersion cooling systems are beneficial in the long run, even if they have a high initial equipment cost. As described by Asetek, companies operating with immersion cooling reported up to 50% energy savings. With increasing demand for more energy-efficient solutions, economies of scale will drive down these upfront costs, making immersion cooling more feasible for smaller companies.
Geographic Diversification: Logistical and Scalability Issues
Challenge: Transporting data centers to the earth’s naturally cooler regions (the Arctic ice) offers a potential cost-saving opportunity but comes with serious logistical challenges. Potentially at high risk is the transportation of hardware to remote sites and the continuous provision of reliable power. According to Microsoft’s research, setting up data centers in the Arctic would require massive investment in infrastructure and may not be scalable in the long term.
Solution: Modular data centers offer flexibility in such environments. For instance, Google’s Edge Computing model allows for closer data processing at the data source, thus reducing the demand for remote large-scale data centers. [also]In particular, underwater data centers—such as Microsoft’s Project Natick—have been demonstrated as effective tools for harnessing natural ocean cooling, reducing power usage, and eliminating land-based logistic challenges.
Data Centers in Cold Climate: Logistical Challenges
Challenge: Cold climate data centers contend with ill weather, transport disruption, and equipment maintenance. According to Project Natick by Microsoft, a submerged data center can render equipment durability and moisture control impossible in cold climates. These challenges must be addressed to scale the deployment of cold climate data centers and make them a more viable option for Green AI practices globally.
Solution: To tackle these hurdles, companies are now building modular and scalable data centers. Submerged data centers like Microsoft’s underwater experiment are cooled efficiently by the cold ambiance, leading to lower energy usage. Modular data centers, such as those found in AWS, are smaller, transportable, and can easily be brought to cold climates where ambient air cooling could be used, thus avoiding the construction of an elaborate infrastructure.
The Future is Green: Embracing AI for a Sustainable Tomorrow
The worldwide market size for AI is expected to become more than $190 billion by 2025. If so, it is expected to consume considerably more energy. Data centers and transmission networks presently consume about 1 percent of the total world demand for electricity, and as AI applications grow, this number will surely rise. So far, companies like Google and Amazon Web Services have made definite commitments to renewable energy, for instance, by reducing their cooling energy consumption by up to 40% using AI optimization through Google’s DeepMind.
It is not simply a concept; it is also an important much-needed direction toward balancing technological advancement with environmental responsibility. To date, Green AI-driven solutions like immersive cooling systems, the use of cold climate data centers, and AI-oriented energy management have reshaped how we think about efficiency and sustainability. Therefore, as Green AI continues to evolve to integrate with green technologies, the combination of AI and green technologies will present the best transformational opportunity to transition into the future.
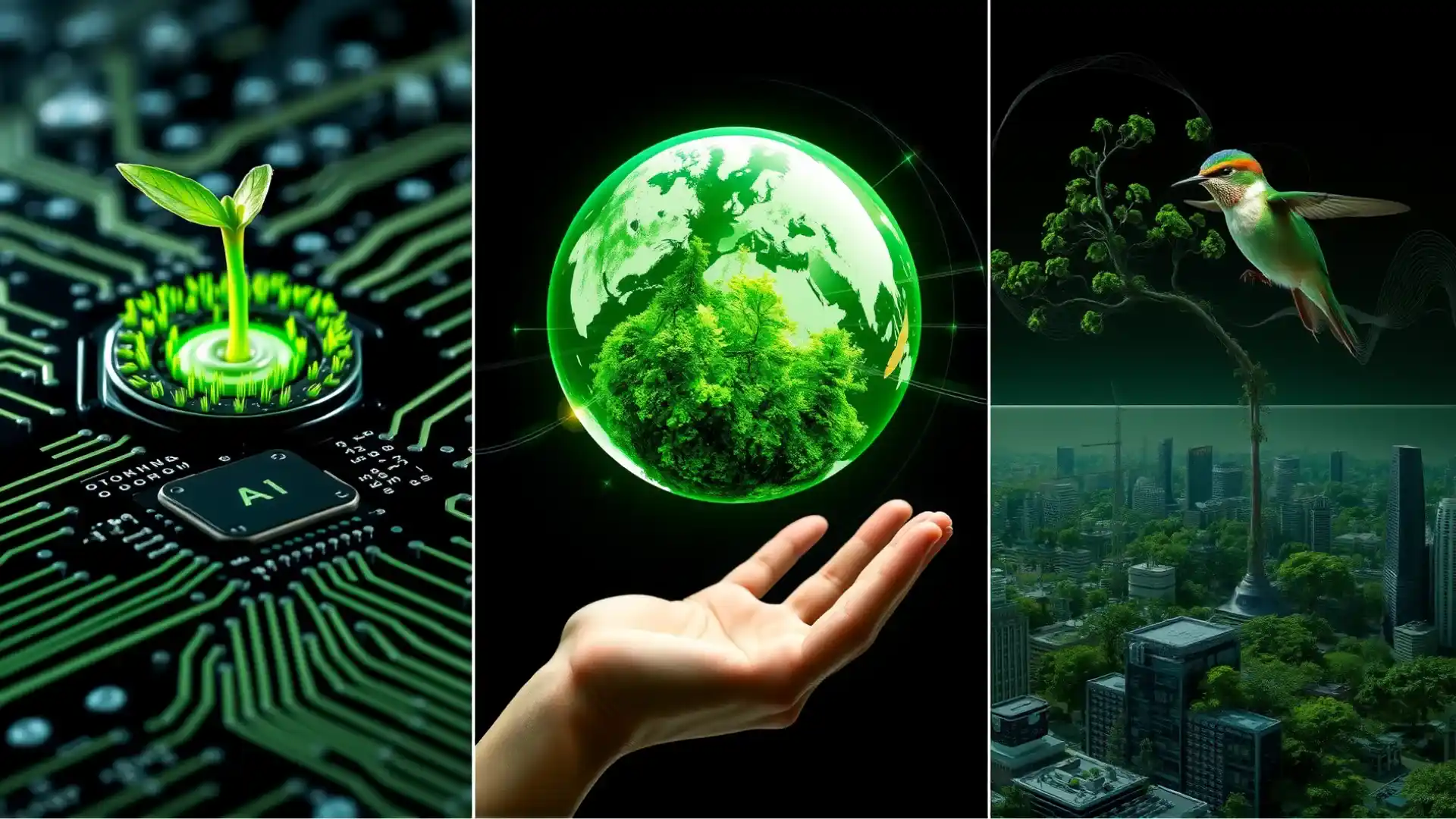
The path to a sustainable AI future is clear. It’s time for companies, innovators, and policymakers to collaborate and integrate Green AI practices into their strategies. Ready to explore how Green AI can revolutionize businesses or projects?