Table of Contents
Election Prediction outcomes have become essential in modern politics, offering insights that influence campaign strategies, media narratives, and public opinion. Accurate election prediction not only helps voters make informed choices but also guides candidates and analysts in understanding key issues and voter priorities.
However, election forecasting is no easy task; it requires combining a variety of data sources and analytical methods to create reliable insights. From polling data and economic indicators to sentiment analysis and machine learning, forecasters employ diverse strategies to capture the dynamics of voter behavior and political trends.
In this guide, we’ll explore five proven strategies for election prediction, highlighting key factors, methodologies, and ways to boost accuracy.
Key Factors in Election Forecasting
- Election predictions require analyzing a range of data sources.
- Political analysts look at:
- Historical data
- Voter demographics
- Economic indicators
- Current events
- Social media trends and public sentiment are increasingly influential.
- Forecasting effectiveness depends on identifying patterns that align with election results.
- Understanding the weight of each factor in different contexts is crucial for refining accuracy.
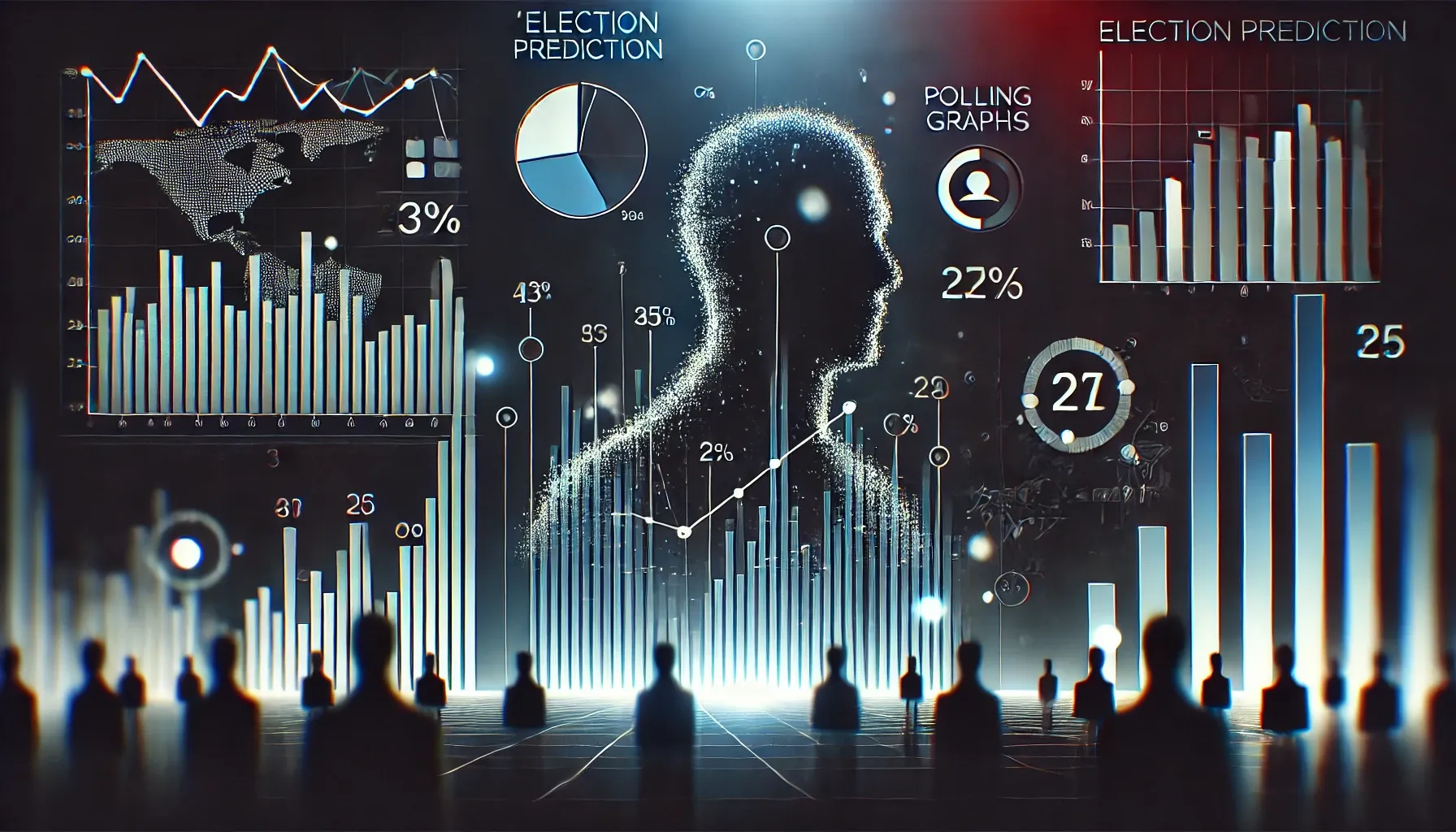
Importance of Polling in Election Prediction
- Polling is one of the most common tools in election forecasting.
- Provides a snapshot of voter intentions and highlights key issues for the electorate.
- Helps predict leading candidates and assess campaign strategies.
- Potential biases and sampling errors mean polls alone aren’t always accurate.
- Online and mobile polling introduce new challenges and opportunities.
- Combining polls with other indicators is essential for a comprehensive electoral view.
Methods for Calculating Weighted Poll Averages
- Averaging multiple polls helps balance outliers and provides a clearer picture.
- Weighted poll averages prioritize reliable sources and recent data.
- Factors influencing weight include:
- Sample size
- Polling methodology
- Polling firm’s track record
- Poll timing
- Weighted averages reduce biases and improve overall prediction accuracy by emphasizing credible sources.
5 Proven Strategies for Election Prediction
- Historical Data Analysis: Historical data analysis is foundational for understanding patterns in voter behavior. By studying past election outcomes and demographic shifts, analysts can identify trends likely to influence future results. For example, certain regions may show consistent support for a particular party or candidate type, while others may fluctuate based on economic conditions or social issues. Historical trends also help pinpoint which factors (e.g., employment rates, public sentiment) typically align with election outcomes, enabling a deeper understanding of voting cycles.
- Weighted Polling Averages: Calculating weighted polling averages can mitigate the effects of outliers and provide a more accurate forecast. Polls from different organizations often show variation, so averaging them provides a balanced perspective. Weighting polls by reliability, sample size, and recency further improves accuracy. This method allows forecasters to prioritize reputable polling sources, giving voters a clearer picture of candidate standings. Weighted averages also provide resilience against biases inherent in individual polls, making this an essential technique for election prediction.
- Economic Indicators: Economic factors such as unemployment rates, inflation, and consumer confidence often influence voter behavior, making them critical components of election prediction. Research shows that economic downturns tend to favor opposition candidates, while periods of growth may benefit incumbents. Analysts track these indicators closely to predict voter priorities and likely swing regions. By understanding how economic factors sway voter sentiment, forecasters can predict shifts in voting patterns, especially in economically sensitive regions.
- Sentiment Analysis on Social Media: Social media has become a powerful tool for gauging public opinion, especially among younger voters. Sentiment analysis, which uses natural language processing to interpret attitudes in online posts and comments, provides real-time insights into voter sentiment. While not always definitive, social media trends can reveal shifts in public opinion and highlight emerging issues. By analyzing keywords, hashtags, and trending topics, sentiment analysis can supplement polling data to create a fuller picture of voter priorities.
- Machine Learning Models for Predictive Analysis: Machine learning models are increasingly used in election forecasting due to their ability to process vast amounts of data and detect complex patterns. By training algorithms on past election data, social trends, and economic indicators, these models identify correlations that may go unnoticed in traditional analysis. Machine learning models can also adjust to changing conditions, making them adaptable and accurate. These models continuously improve as they analyze new data, providing an up-to-date snapshot of voter sentiment.
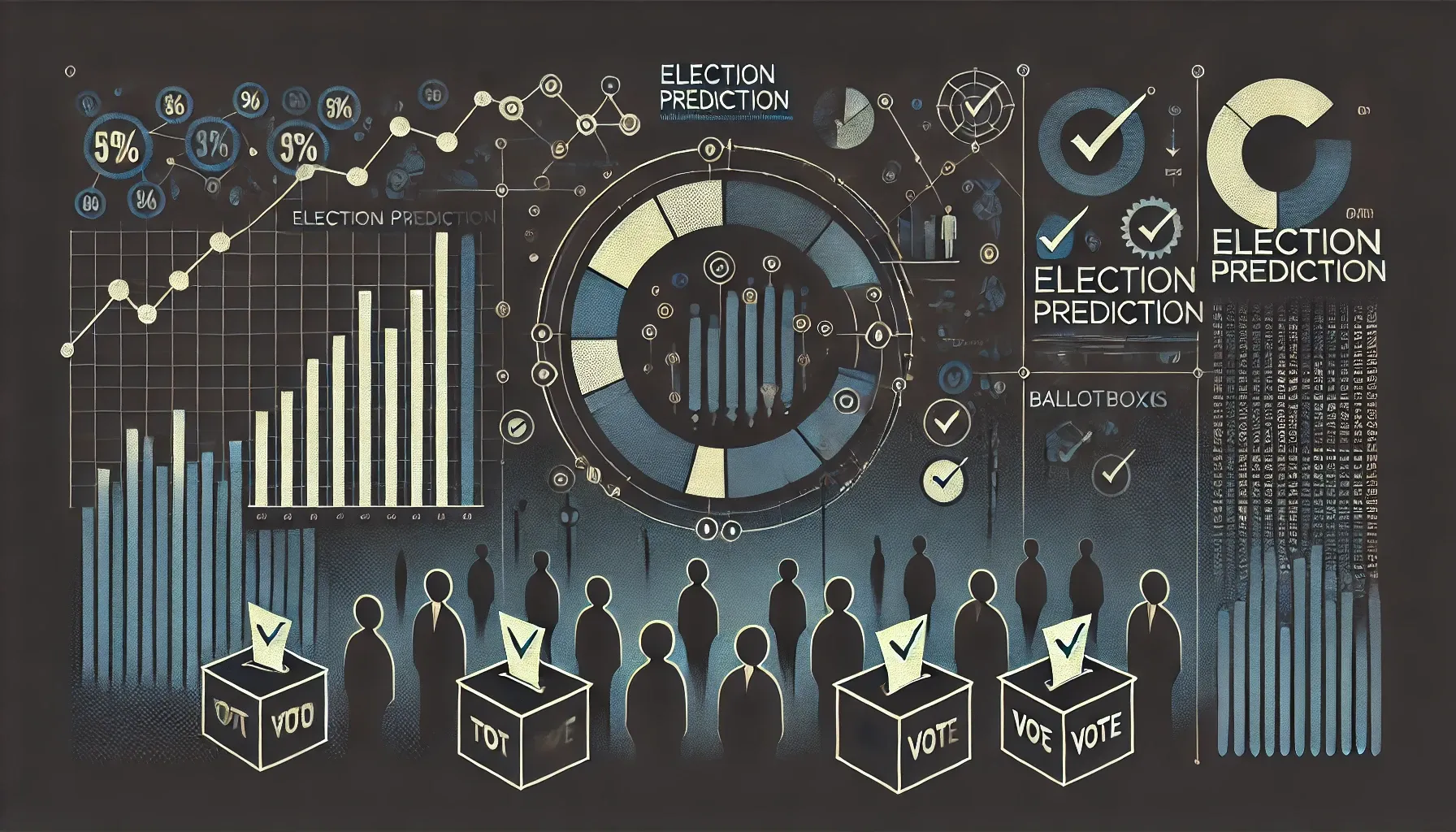
Conclusion
Election prediction is a complex and evolving field that draws on data from a variety of sources. By combining historical data, weighted polling, economic indicators, sentiment analysis, and machine learning, forecasters can create robust, adaptive models that offer a clearer view of likely election outcomes.
Each of these strategies provides unique insights, and when used together, they can significantly improve the accuracy of election predictions. As data availability and analytical tools continue to advance, election prediction will become an even more reliable tool for understanding political landscapes and voter behaviour.