Table of Contents
In the ever-evolving landscape of pharmaceutical research, the integration of machine learning (ML) algorithms has emerged as a game-changer. This article dives deeper into the transformative impact of ML in drug discovery, focusing on its revolutionary role in expediting processes within the realm of pharmaceutical research.
Pharmaceutical research has a rich history, evolving from traditional herbal remedies to sophisticated modern drug discovery processes. In ancient times, medicinal knowledge was often based on empirical observations and traditional remedies. The development of systematic pharmaceutical research gained momentum during the Middle Ages and the Renaissance, with the compilation of pharmacopeias and the establishment of apothecaries.
The ultimate goal of pharmaceutical research is to develop effective treatments that improve patient outcomes. By leveraging machine learning, the drug development process becomes more efficient, reducing the time it takes for promising drugs to reach the market. This acceleration not only benefits pharmaceutical companies by reducing costs and increasing productivity but, more importantly, it has a direct positive impact on patients. Improved efficiency in drug discovery means that potentially life-saving medications can reach those in need more swiftly, addressing unmet medical needs and enhancing overall patient care.
1. Traditional Challenges in Pharmaceutical Research
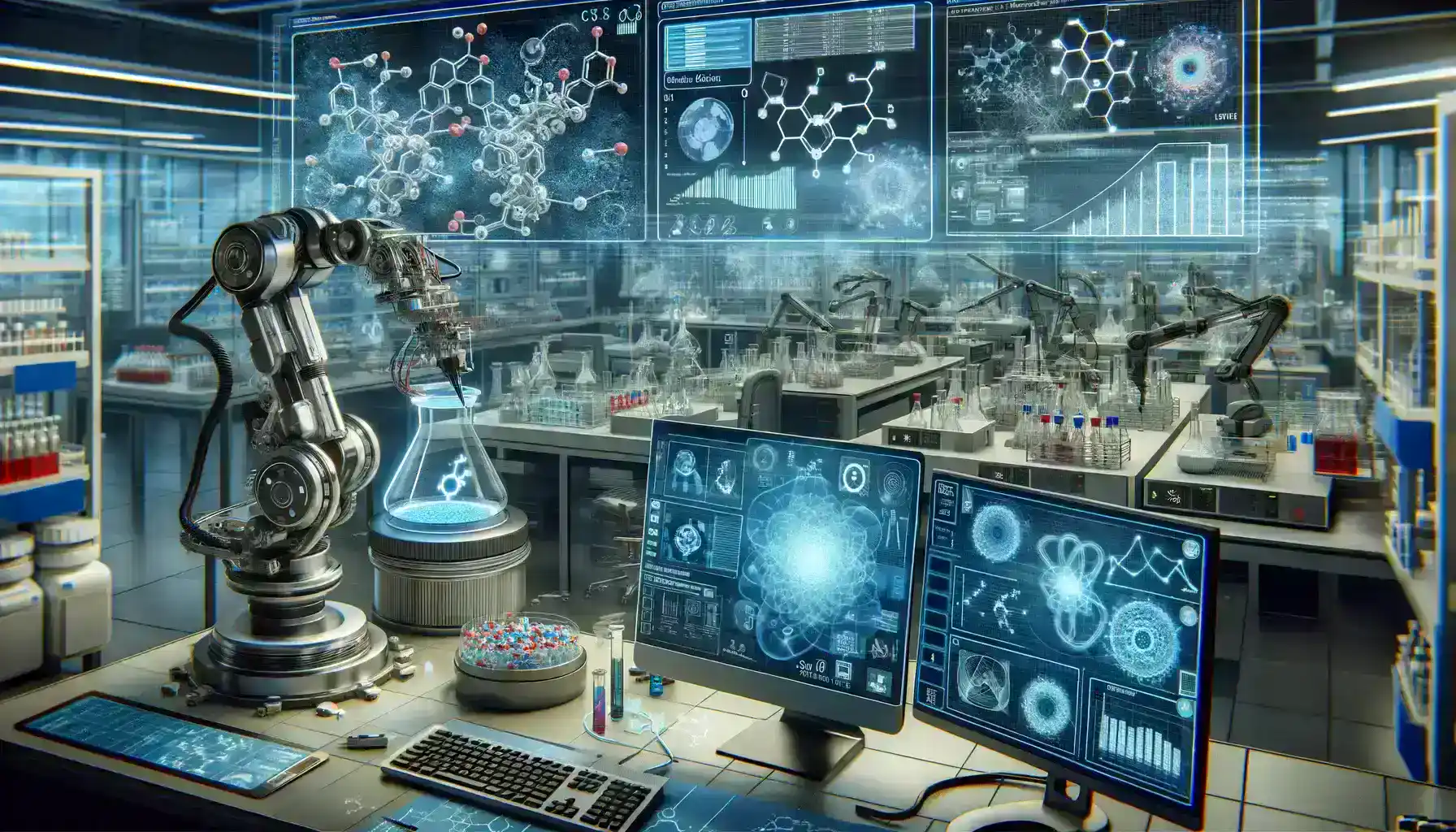
Intricate and Time-Consuming
Complex Processes: Traditional process involves a series of intricate and interconnected processes, including target identification, lead optimization, and clinical trials. Each step demands meticulous attention and can be time-consuming.
Laborious Development: The development of potential drug candidates requires extensive research, experimentation, and validation, adding layers of complexity to the overall process.
BenevolentAI uses machine learning to analyze biological data and identify novel drug targets for diseases like amyotrophic lateral sclerosis (ALS) and Parkinson’s.
High Costs
Research and Development Expenses: Pharmaceutical research is associated with substantial financial investments, covering costs such as laboratory equipment, personnel, and the acquisition of research materials.
Technological Infrastructure: Keeping pace with advancements in technology and maintaining state-of-the-art infrastructure adds to the overall cost burden on pharmaceutical companies.
Novartis, a global pharmaceutical company, has been at the forefront of gene therapy research. Their investments in this area encompass exploring innovative therapeutic approaches, including personalized gene therapies.
Low Success Rates
Clinical Trial Failures: A significant proportion of drug candidates fail to progress beyond clinical trials due to factors like insufficient efficacy, unexpected side effects, or inadequate safety profiles.
Regulatory Hurdles: Strict regulatory standards for drug approval contribute to the high attrition rate, as drugs must meet rigorous safety and efficacy criteria to advance to the market.
Alzheimer’s Drug Aducanumab: The FDA faced challenges in evaluating the drug’s efficacy and safety, leading to a complex approval process
2. Introduction of Machine Learning in Pharmaceutical Research
Paradigm Shift
Transformative Impact: The integration of machine learning in pharmaceutical research signifies a fundamental shift in the traditional approaches to drug discovery.
Novel Perspectives: Machine learning introduces novel perspectives by leveraging computational capabilities to analyze complex data sets, allowing researchers to extract insights and patterns that might be challenging to discern through conventional methods.
AlphaFold leverages machine learning to predict protein folding, a critical aspect in drug discovery. In 2020, AlphaFold made headlines by accurately predicting the 3D structures of proteins, a longstanding challenge in biology. This breakthrough has profound implications for understanding diseases at the molecular level and designing drugs more precisely.
Streamlining Processes
Optimizing Resource Utilization: Machine learning offers unprecedented opportunities to streamline and optimize key stages of drug discovery, starting from target identification to clinical trial design.
Efficiency Gains: By automating repetitive tasks and providing predictive modeling capabilities, machine learning enables researchers to focus on more complex, strategic aspects of drug development, leading to efficiency gains.
Atomwise, a company specializing in AI for drug discovery, employs machine learning to predict the binding affinity of small molecules to specific protein targets. Their technology analyzes the structural features of compounds and predicts their potential efficacy, significantly reducing the time and resources required for hit identification.
Enhancing Decision Making
Data-Driven Insights: Machine learning equips researchers with data-driven insights, guiding decision-making processes throughout drug discovery.
Iterative Learning: Machine learning models can iteratively learn from new data, continuously refining their predictions and insights. This iterative learning process enhances the adaptability of drug discovery strategies in response to emerging trends or new information.
Real-Time Data Integration: As new viral strains emerge, genomic data, clinical trial results, and patient outcomes are continuously fed into the machine-learning model in real time. This influx of data reflects the dynamic nature of viral evolution and the need for up-to-date information in drug discovery.
Accelerating Iterative Cycles
Quick Iterative Feedback: Traditional drug discovery often involves lengthy experimental cycles. Machine learning facilitates quick and iterative feedback loops, allowing researchers to adapt their strategies based on real-time insights.
Early Identification of Promising Leads: Predictive modeling capabilities in machine learning accelerate the identification of promising drug candidates, enabling researchers to prioritize the most viable options early in the process.
Insilico Medicine, a biotechnology company, has leveraged machine learning to revolutionize drug discovery through its innovative platform. The company utilizes deep learning algorithms and generative adversarial networks (GANs) to predict and generate molecular structures with the potential to become effective drugs.
3. Key Benefits of Machine Learning in Pharmaceutical Research
Data Processing Power
Handling Large Datasets: Traditional pharmaceutical research involves massive volumes of data from various sources, including genomics, proteomics, and clinical trials. Machine learning algorithms excel in efficiently processing and managing these large datasets, overcoming the limitations of manual processing and analysis.
Real-time Analysis: Machine learning enables real-time analysis of data streams, providing timely insights into ongoing experiments, clinical trials, and other research activities. This real-time capability enhances decision-making and allows for quick adjustments in research strategies.
Clinical Trial Monitoring: Researchers can promptly adjust the trial parameters, dosage levels, or even inclusion criteria based on real-time insights, ensuring participant safety and optimizing the trial’s success.
Pattern Recognition
Identifying Complex Patterns: Machine learning algorithms are adept at recognizing intricate patterns and correlations within diverse datasets. In the context of pharmaceutical research, these patterns may be subtle interactions between genes, proteins, or other biological entities that are challenging for human researchers to identify manually.
Target Identification Assistance: In the early stages of drug discovery, identifying suitable drug targets is a complex task. Machine learning’s pattern recognition capabilities can sift through biological data to pinpoint potential targets, helping researchers focus their efforts on the most promising avenues.
Multi-Omics Integration: Machine learning excels at integrating data from various ‘omics’ levels, such as genomics, proteomics, and metabolomics. By identifying cross-level patterns, these algorithms can unveil comprehensive insights into the complex interplay of biological entities in cancer, potentially leading to the discovery of novel therapeutic targets or diagnostic markers
Accelerated Drug Discovery
Task Automation: Machine learning automates various aspects of the drug discovery pipeline, reducing manual labor and expediting routine tasks. This includes tasks such as data preprocessing, feature selection, and even aspects of experimental design.
Predictive Insights: Machine learning models can provide predictive insights based on historical data, aiding researchers in making informed decisions about the likelihood of success for specific drug candidates. This predictive capability streamlines the decision-making process, allowing researchers to prioritize resources effectively.
Predictive Toxicology: Assessing the potential toxicity of compounds is a crucial step in drug development. Machine learning models trained on historical toxicity data can predict the toxicity of new compounds, automating the evaluation process. This helps researchers focus on compounds with safer profiles early in the drug discovery pipeline.
4. Synergy of Human Expertise and Machine Learning in Pharmaceutical Research
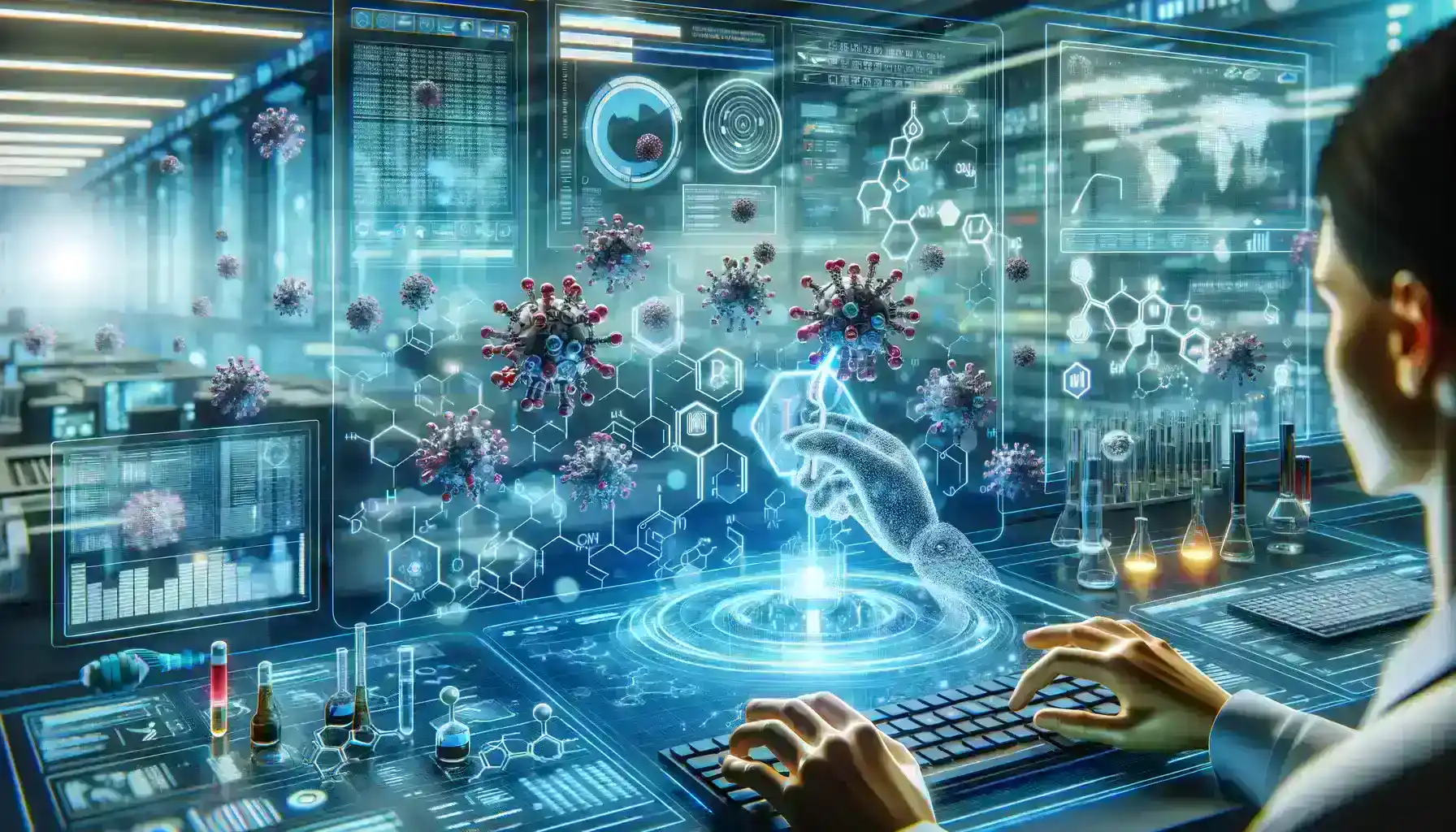
Complementary Roles
Human Expertise: Human researchers bring a wealth of knowledge, experience, and intuition to the table. Their ability to understand the nuances of biological systems, interpret complex data, and apply contextual knowledge is invaluable.
Machine Learning Contribution: On the other hand, machine learning excels in processing vast amounts of data quickly and identifying patterns that may elude human observation. It automates repetitive tasks, allowing researchers to focus on higher-level decision-making.
IBM Watson for Drug Discovery is an AI-driven platform that processes vast amounts of biomedical literature, including research papers, clinical trial reports, and scientific articles.
Analytical Prowess
Human Creativity: Human researchers possess creative problem-solving skills, enabling them to devise innovative hypotheses, experimental designs, and novel approaches to drug discovery. They can adapt to unexpected challenges and think outside the box.
Machine Learning Analytical Capabilities: Machine learning algorithms excel in analytical tasks, such as predictive modeling and data mining. They can process and analyze data at a scale and speed that would be impractical for humans, providing valuable insights into complex datasets.
Clinical Trial Optimization by Owkin: Owkin uses machine learning to optimize the design and execution of clinical trials. This analytical capability assists in identifying optimal trial designs, patient populations, and potential challenges, ultimately improving the efficiency and success rates of clinical trials.
Enhanced Decision-Making
Human Judgment: Human researchers provide the critical judgment needed to interpret results, consider ethical implications, and make decisions based on a nuanced understanding of the broader scientific and societal context.
Machine Learning Support: Machine learning aids in decision-making by providing data-driven insights and predictions. It can prioritize potential drug candidates, optimize experimental designs, and assist in identifying optimal conditions for success.
AlphaFold employs deep learning to predict the 3D structure of proteins. Understanding protein structures is crucial in drug discovery, as it helps researchers design drugs that interact more effectively with target proteins. By providing accurate predictions, AlphaFold aids researchers in making informed decisions about potential drug candidates.
5. Future Outlook
Continued Evolution
Advancements in Algorithms: As technology continues to progress, machine learning algorithms are anticipated to become more sophisticated and capable. This evolution may lead to improved accuracy in predictive models and a better understanding of complex biological systems.
Integration of New Technologies: The incorporation of complementary technologies, such as advanced imaging techniques, high-throughput screening, and omics technologies (genomics, proteomics, etc.), with machine learning is likely to enhance the overall drug discovery process.
Quantum computing, when integrated with machine learning, can simulate molecular interactions with unprecedented speed and accuracy. This allows for more efficient virtual screening of compounds and prediction of their behavior in biological systems. The combination of quantum computing and machine learning holds the potential to revolutionize molecular simulations in drug discovery.
Synergistic Relationship
Human-Machine Collaboration: The future of drug discovery will see an increasing emphasis on collaboration between machine-learning algorithms and human expertise. Rather than replacing human researchers, machine learning will serve as a powerful tool to augment and amplify their capabilities.
Interdisciplinary Collaboration: The future will likely witness increased collaboration between data scientists, computer scientists, biologists, chemists, and other experts. This interdisciplinary approach is essential for developing holistic solutions that consider both the technical and biological aspects of drug discovery.
Project DataSphere is a global initiative in oncology that involves collaboration between data scientists, oncologists, and bioinformaticians. It aims to share and analyze clinical trial data to accelerate the development of cancer treatments. By pooling data and expertise from different disciplines, the platform facilitates the identification of patterns and insights that can inform more effective cancer therapies.
Application in Specific Therapeutic Areas
Personalized Medicine: Machine learning’s role in tailoring treatments to individual patients based on their genetic makeup, lifestyle, and other factors is expected to grow. This personalized medicine approach has the potential to significantly improve treatment efficacy and reduce adverse effects.
Rare Diseases and Neglected Conditions: Machine learning can contribute to the discovery of treatments for rare diseases and neglected conditions by uncovering patterns in limited datasets and identifying potential drug candidates that might have been overlooked using traditional methods.
Pharmacogenomics involves studying how an individual’s genetic makeup influences their response to drugs. Machine learning is applied to understand the complex relationships between genetic variations and drug responses.
Conclusion
Machine learning serves as a catalyst for accelerated innovation within the pharmaceutical research landscape. The ability of machine learning algorithms to rapidly process and analyze vast amounts of data expedites key stages of drug discovery. This acceleration translates into quicker identification of potential drug candidates, optimized clinical trial designs, and faster decision-making throughout the research and development process.
The ultimate goal of pharmaceutical research is to develop effective treatments that improve patient outcomes. By leveraging machine learning, the drug development process becomes more efficient, reducing the time it takes for promising drugs to reach the market. This acceleration not only benefits pharmaceutical companies by reducing costs and increasing productivity but, more importantly, it has a direct positive impact on patients. Improved efficiency in drug discovery means that potentially life-saving medications can reach those in need more swiftly, addressing unmet medical needs and enhancing overall patient care.
In conclusion, the integration of machine learning represents not just an incremental improvement but a revolutionary leap forward in pharmaceutical research. This leap accelerates innovation, improves patient outcomes, enhances precision and personalization, and fosters a collaborative and adaptive research environment. As the pharmaceutical industry continues to embrace and refine these technologies, the future holds great promise for more effective, efficient, and personalized therapeutic interventions.