Table of Contents
Cybersecurity threats aren’t slowing down. They’re getting smarter, faster, and harder to trace.
That’s why many companies are turning to machine learning (ML) in 2025. We’re seeing traction in areas like anomaly detection, automated response, and phishing defense.
In this post, we’ll explore the concrete ways ML is rewriting the rules of online security and how it can be a key weapon in your digital arsenal.
What Machine Learning Means for the Future of Cybersecurity
62% of companies recognize how machine learning could enhance their cybersecurity efforts, according to MixMode. Machine learning offers the ability to analyze vast amounts of data, detect patterns humans might miss, and respond to malicious activities in real time.
Let’s look at how organizations are using machine learning right now to strengthen cybersecurity and what that means for the future of data protection.
Smarter, Faster Threat Detection
Traditional threat detection relies on predefined rules and known threat signatures. That works for threats we’ve already seen.
Machine learning shifts the focus to behavioral analysis. It learns what normal looks like across users, devices, and networks. Then, it flags deviations that may indicate an attack. This allows systems to identify suspicious activity in real time, even if you’ve never encountered the threat before.
Whether it’s unusual login behavior, irregular file movement, or odd patterns in network traffic, ML-based detection shortens the time between breach and response. That speed matters. It gives your organization a chance to stop a threat before it becomes a full-scale incident.
To improve threat detection, you can use public web data to simulate attacks or uncover vulnerabilities before they escalate. Platforms like Bright Data help security teams gather real-time data at scale, which can sharpen machine learning models for phishing detection, credential leaks, or abnormal behavior across the web.
Machine learning models detect anomalies, phishing sites, malware signatures, and unusual behavior in real time—before a threat causes damage.
Bright Data enables real-time web scraping of malicious domains, IP addresses, forums, and dark web marketplaces and provides training data for ML algorithms.
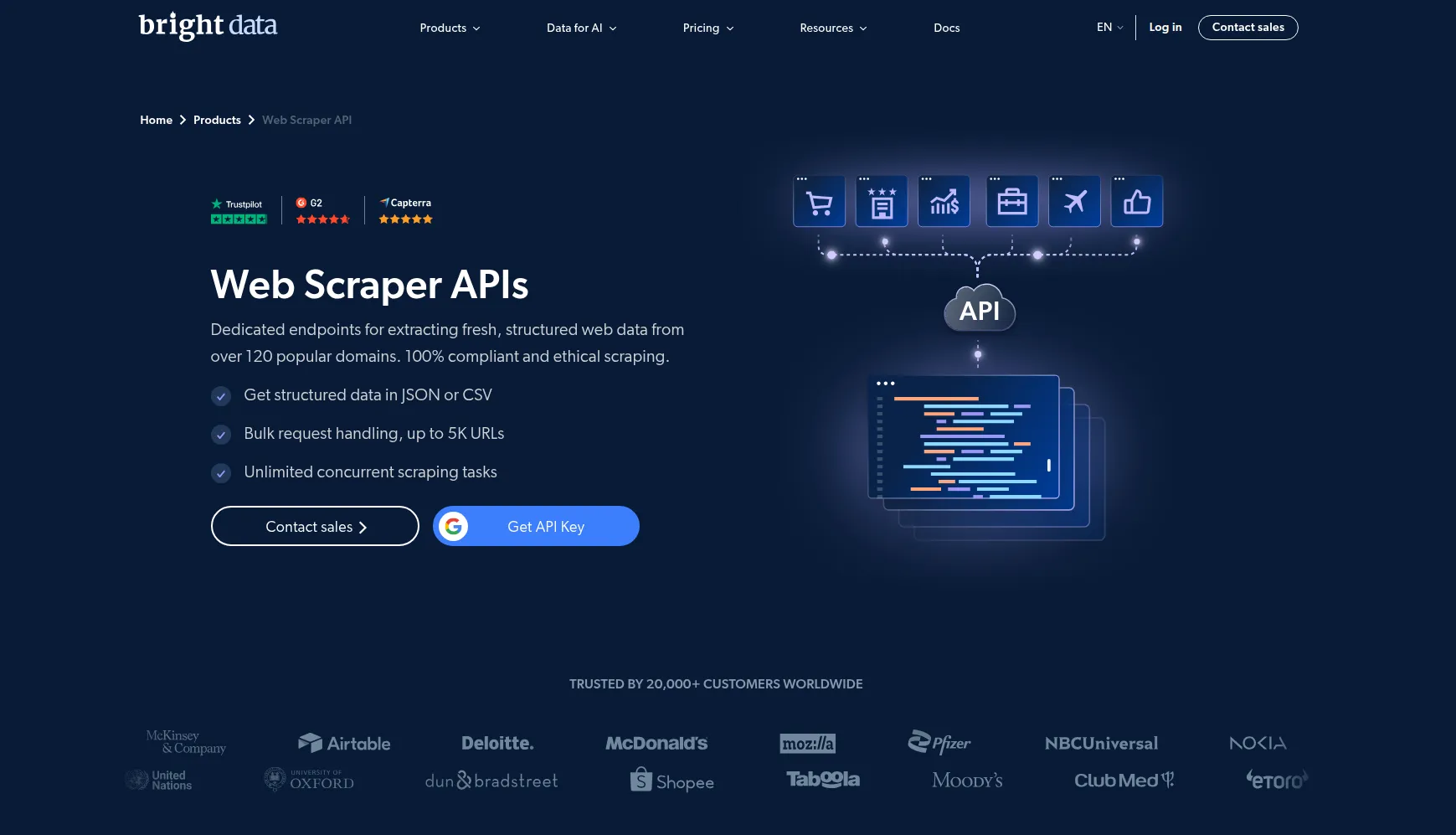
This, in turn, enables cybersecurity firms to build up-to-date threat intelligence datasets for the early detection of phishing and malware campaigns. IT can also help train ML models by providing massive volumes of clean, structured, and location-specific data across the web. As a result, cybersecurity teams can train models on real-world scenarios.
That way, they can gather global threat patterns, which allows models to adapt to localized attacks or region-specific vulnerabilities.
Automated Incident Response
The faster your organization can respond to an attack, the less damage it suffers. Once ML-powered systems identify a threat, they can automatically trigger containment actions like isolating endpoints, disabling compromised accounts, or blocking IP addresses as part of the incident response process. They can do all this without waiting for manual approval.
These automated workflows are based on real-time analysis and predefined risk thresholds, which help prevent cyber threats from spreading through the system. In high-volume environments where thousands of alerts are generated daily, automation ensures urgent incidents get the attention they need.
Adaptive Defense Systems
Most security solutions are built to react. ML introduces a more proactive model. It doesn’t rely on periodic updates or manual rule changes. Instead, these models are constantly evolving based on new data. As attacks change tactics, the system learns and adjusts.
This is key in complex environments where cyber threats can come from any direction, whether internal, external, deliberate, or accidental.
Adaptive systems can fine-tune firewalls, update access policies, and modify detection thresholds based on new user behavior patterns, all without human intervention.
Phishing and Social Engineering Prevention
In a 2023 global survey by Statista, 30% of cybersecurity professionals ranked natural language processing (NLP) as the second most promising artificial intelligence (AI) technique for enhancing defenses (detecting malware was number one). They see strong potential in using NLP to detect advanced phishing attempts more effectively.
And this couldn’t have come at a better time. Phishing emails are now even more difficult to detect, especially as attackers use more convincing language and design.
These attacks have significant consequences, from network outages to reputational damage. Below are the most common issues businesses face after a successful phishing attack.
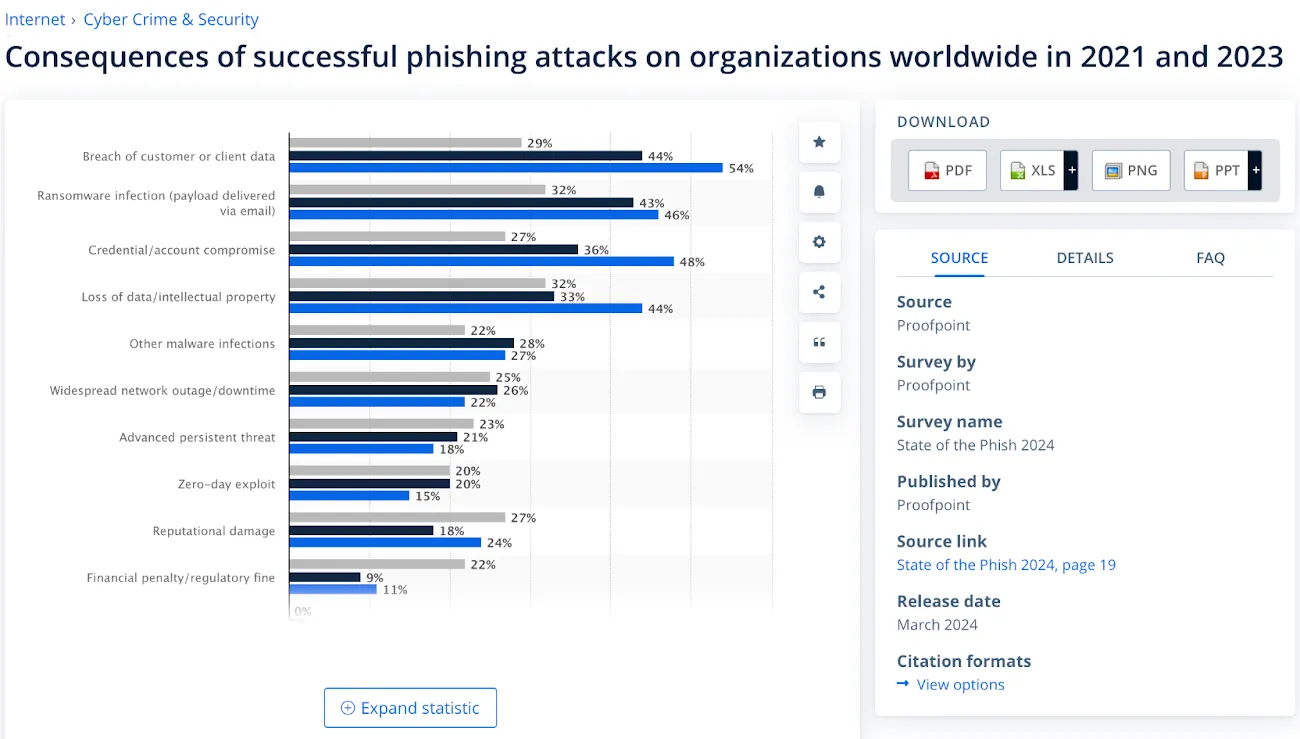
NLP, a form of machine learning, is helping systems analyze the content of emails and messages in ways traditional filters can’t.
NLP models evaluate sentence structure, tone, urgency cues, and sender behavior to determine whether a message might be attempting to deceive the user.
Identity and Access Management (IAM)
ML is redefining what secure access looks like. Instead of relying solely on passwords or basic multi-factor authentication (MFA), ML-based IAM systems monitor how users interact with systems over time.
These models build behavioral profiles. They track things like typing speed, login times, device usage, and geographic location. When a user’s behavior doesn’t match their established pattern, the system can flag it as suspicious or require additional verification.
Reducing False Positives and Alert Fatigue
Traditional tools create a large volume of alerts, which often overwhelm security teams. Many of these alerts are low-priority or harmless.
However, they still demand time and attention. Machine learning helps reduce this noise by classifying events based on risk and learning which patterns are actually suspicious.
Over time, the system improves its accuracy. It surfaces only high-priority alerts that require human review. This can help your security team improve efficiency, reduce burnout, and ensure you’re not missing critical threats because they were buried under false positives.
How You Can Implement Machine Learning into Your Cybersecurity Strategy Today
Below are the steps that security teams are using to make machine learning part of their everyday defense strategy.
Start With the Right Data
Machine learning is only as good as the data it learns from. Begin by evaluating what data your organization already collects. This might include:
- Network logs
- User behavior analytics
- Incident reports
- Access records
- Email traffic
Prioritize clean, well-labeled data that covers diverse environments and threat types. If your data is fragmented or you store it across systems, invest in integration.
Identify High-Impact Use Cases
Not every part of your security stack needs machine learning. Focus on areas where ML offers clear value, such as phishing detection, anomaly detection, automated response, or access control.
Pick one or two use cases to pilot. This allows your team to learn the system, track ROI, and build internal confidence before expanding further.
Choose Tools With Explainable AI
Choose ML platforms or vendors that provide transparency. For example, this could be clear explanations of how decisions are made, confidence scores, or options for analyst feedback.
This helps your team trust the system, refine its accuracy, and ensure compliance with internal policies and regulations.
Integrate With Your Existing Security Stack
ML tools should enhance your SIEM, SOAR, EDR, and IAM systems. Look for platforms that support open APIs or built-in integrations with the tools you already use.
Document Everything From Day One
As you implement machine learning into your workflow, document each step. That includes the data sources you use, the models you deploy, the rules you set, the thresholds you test, and team feedback.
This helps ensure compliance, supports auditing, and makes it easier to troubleshoot or retrain your models down the line. Clear documentation also helps future-proof the process for turnover or scaling.
Plan for Model Maintenance or Drift
Machine learning models don’t run on autopilot forever. Over time, the patterns they’ve learned may become outdated as attackers change tactics or your infrastructure evolves.
Build a plan for monitoring performance, retraining models, and making regular updates. Assign responsibility for reviewing false positives, false negatives, and edge cases.
Build Human-Machine Collaboration
The goal of using ML is to enhance your cybersecurity efforts. You never want to use them to replace human oversight.
Assign security analysts to monitor ML-driven alerts, validate decisions, and fine-tune thresholds. Encourage regular feedback between humans and machines so the model continues to improve in accuracy and relevance.
Upskill Your Security Team
As you make ML more of a staple in your day-to-day security operations, your team needs to understand how it works. According to Nikola Roza, 94% of business management and leaders say they want to upskill employees by 2026. Offer training on how to interpret ML outputs, work with AI-driven platforms, and manage the risks of automation.
Work With Trusted Vendors and Partners
Not every organization has the internal resources to build machine learning algorithms from scratch. And that’s okay. Many cybersecurity vendors now offer machine learning features built into their platforms. The key is to vet how their models work, offer real-time support, and provide transparency into model updates and tuning.
Measure, Refine, and Scale
Once you’ve deployed ML in one area, track its performance. Are response times faster? Are false positives down? Is the team spending less time on low-risk noise?
Use these insights to optimize the setup, adjust parameters, and expand ML into other parts of your security strategy.
Final Thoughts
There was a time when machine learning was an experimental add-on in cybersecurity. Now, it’s becoming a key part of how modern organizations defend against complex, fast-moving potential threats.
Integrating machine learning into your defenses can help your organization respond faster, prioritize threats more effectively, and reduce strain on your teams.
Is your current security setup keeping pace with the complexity of today’s threats?
Now is the time to move past passive monitoring and adapt tools that can think, learn, and act with you.