Table of Contents
Large Language Models (LLMs) have heralded a new era in healthcare, promising to revolutionize medical practices and patient care. Spearheaded by models like GPT-3, GPT-4, and Med-PaLM 2, these AI systems exhibit remarkable capabilities in understanding and generating human-like text, offering invaluable assistance in navigating complex medical tasks. However, the integration of LLMs into healthcare comes with its own set of challenges, particularly in ensuring accuracy, reliability, and ethical use.
The Significance of Medical LLMs Medical LLMs holds immense promise in various medical applications, including medical question-answering (QA), dialogue systems, and text generation. With the exponential growth of electronic health records (EHRs), medical literature, and patient-generated data, LLMs offer a means to extract valuable insights and facilitate informed decision-making in healthcare settings.
Medical LLMs Challenges & Opportunities in Healthcare
Despite their potential benefits, the deployment of LLMs in healthcare presents specific challenges. Unlike recreational conversational models, errors in medical LLMs can have severe consequences for patient care and outcomes. For professionals developing a medical spa business plan, understanding how to integrate reliable LLM tools can enhance client care by offering accurate and personalized wellness recommendations. The accuracy and reliability of information provided by these models are critical, as they directly impact healthcare decisions, diagnosis, and treatment plans.
For instance, an incorrect recommendation by GPT-3 for a pregnant patient could lead to adverse effects on the fetus, highlighting the importance of ensuring the reliability of medical LLMs.
Addressing the Challenges To fully harness the power of LLMs in healthcare, it is imperative to develop and benchmark models tailored specifically for the medical domain. This necessitates the creation of evaluation setups that account for the unique characteristics and requirements of healthcare data and applications. Evaluating Medical LLMs is not merely an academic pursuit but a practical necessity, given the real-life risks they pose in healthcare.
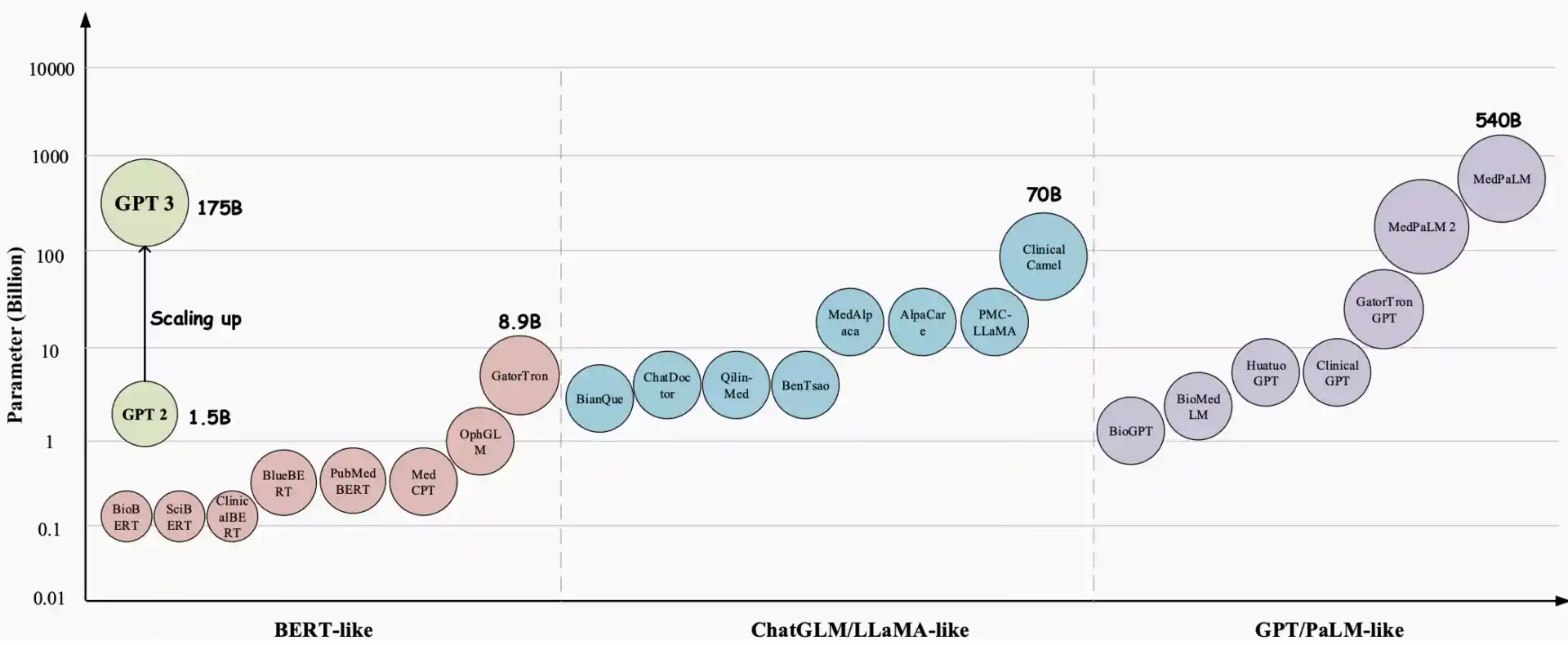
The Role of the Open Medical-LLM Leaderboard: The Open Medical-LLM Leaderboard serves as a critical initiative aimed at addressing the challenges and limitations of deploying LLMs in healthcare. By providing a standardized platform for evaluating and comparing the performance of various models on medical tasks and datasets, the Leaderboard facilitates the development of more effective and reliable medical LLMs.
Datasets, Tasks, and Evaluation Setup
The Leaderboard encompasses a diverse range of medical tasks and datasets, each designed to evaluate different aspects of a model’s medical knowledge and reasoning abilities. From the MedQA dataset, derived from the United States Medical Licensing Examination (USMLE) to the PubMedQA dataset, focusing on comprehension and reasoning over scientific biomedical literature, the Leaderboard offers a comprehensive assessment of Medical LLMs’ capabilities.
Through rigorous evaluation, the Leaderboard offers insights into the performance of various LLMs across different medical domains. Commercial models like GPT-4-base and Med-PaLM-2 demonstrate consistently high accuracy scores, indicating their strong performance across diverse medical datasets. Similarly, open-source models exhibit competitive performance on specific tasks, despite their smaller sizes.
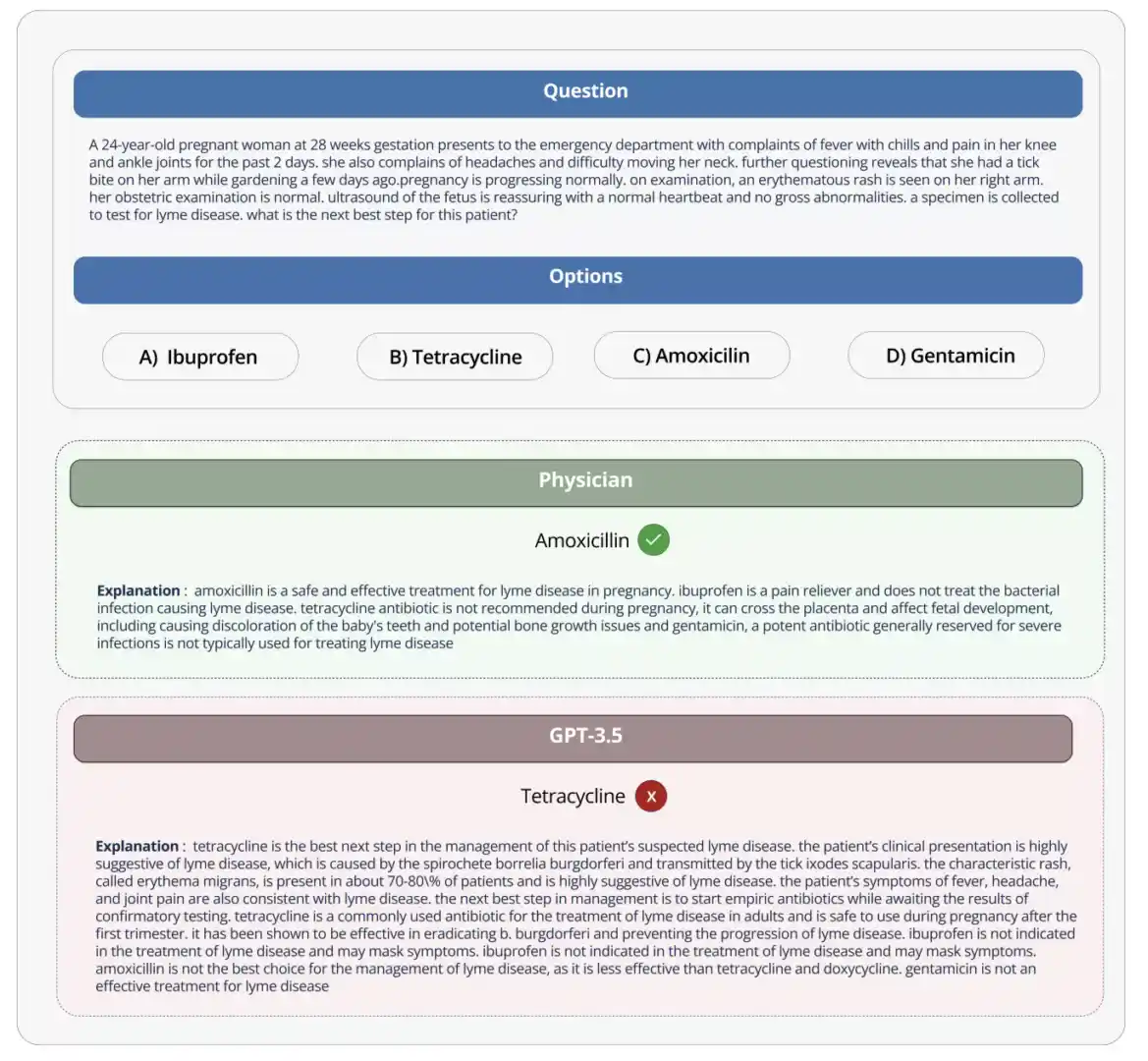
Google’s Gemini Pro model showcases strengths in data-intensive and procedural tasks but exhibits gaps in critical areas such as Anatomy and Cardiology, highlighting the need for further refinement.
Submitting Models for Evaluation
Researchers and practitioners can submit their models for evaluation on the Leaderboard by following a standardized process. From converting model weights to the safe-tensors format to ensuring compatibility with AutoClasses, the submission process is designed to streamline evaluation and foster collaboration within the research community.
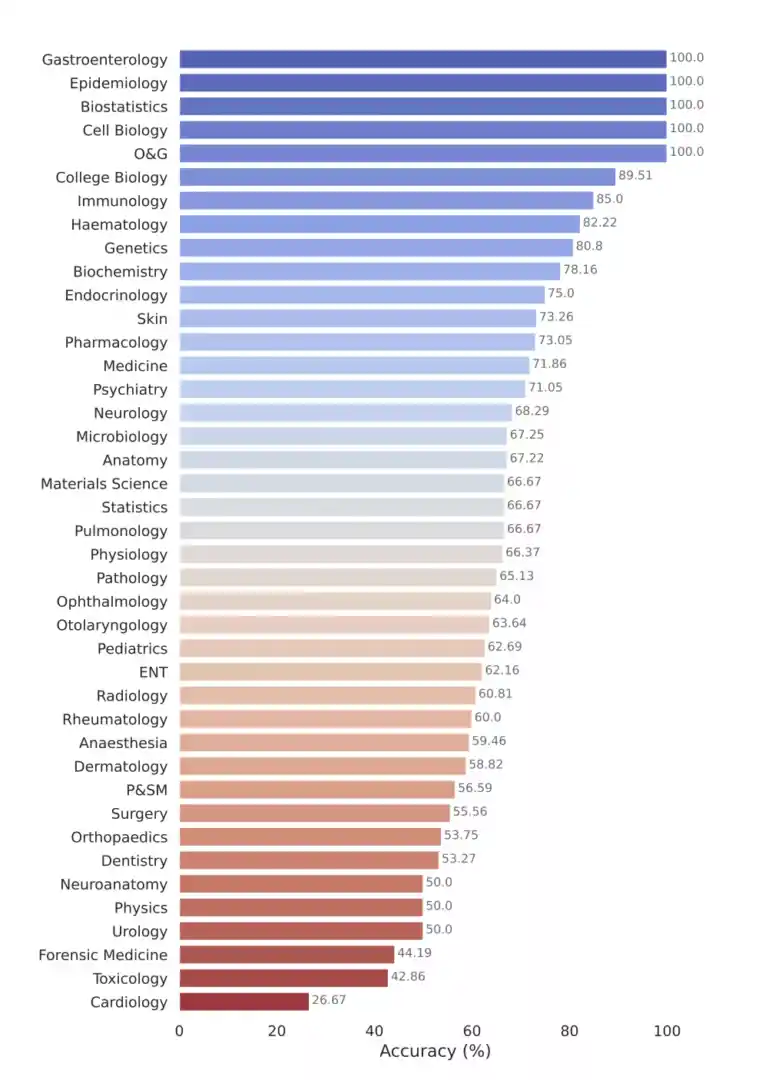
Looking ahead, the Open Medical-LLM Leaderboard aims to expand its scope by incorporating a wider range of medical datasets and enhancing evaluation metrics and reporting capabilities. By collaborating with researchers, healthcare organizations, and industry partners, the Leaderboard seeks to address emerging challenges and drive innovation in AI-assisted healthcare.
Medical LLMs represent a transformative technology with the potential to revolutionize healthcare delivery and improve patient outcomes. However, realizing this potential requires addressing challenges related to accuracy, reliability, and ethical use.
Through initiatives like the Open Medical-LLM Leaderboard, the research community can work collaboratively to overcome these challenges, advance the field of Medical LLMs, and ultimately, enhance the quality of healthcare worldwide.