Table of Contents
The Unexplored Challenge of Image Recognition Difficulty
In an era where artificial intelligence (AI) powers critical applications across healthcare, transportation, and everyday devices, the concept of image recognition difficulty for humans has remained largely unexplored. Despite the significant role of visual data understanding, little attention has been given to understanding the inherent complexity of recognizing images.
Deep learning-based AI has thrived on the availability of datasets, but our understanding of how data influences progress in large-scale deep learning is limited. Humans consistently outperform AI models in practical scenarios that demand visual data comprehension. Even though current datasets are designed to challenge AI with debiased images or distribution shifts, the problem endures due to a lack of guidance on image and dataset difficulty.
To bridge this knowledge gap, David Mayo, an MIT PhD student in electrical engineering and computer science and a CSAIL affiliate, delved deep into the realm of image datasets to understand why some images pose greater challenges in recognition. He emphasizes the importance of comprehending how the human brain processes such images and how this relates to machine learning models. This exploration is pivotal in advancing machine vision models and comprehending the relationship between human perception and AI.
Mayo’s research culminated in the creation of a groundbreaking metric known as the “minimum viewing time” (MVT), which quantifies the difficulty of recognizing an image based on how long a person needs to view it before making a correct identification. To assess this metric’s effectiveness, the team conducted experiments using subsets of ImageNet, a popular dataset in machine learning, and ObjectNet, a dataset designed to test object recognition robustness. Participants were shown images for durations ranging from a mere 17 milliseconds to a substantial 10 seconds and were then tasked with identifying the correct object from a set of 50 options.
The results were illuminating. Existing test sets, including ObjectNet, seemed biased toward easier and shorter MVT images, with most benchmark performance derived from images that are relatively easy for humans to recognize.
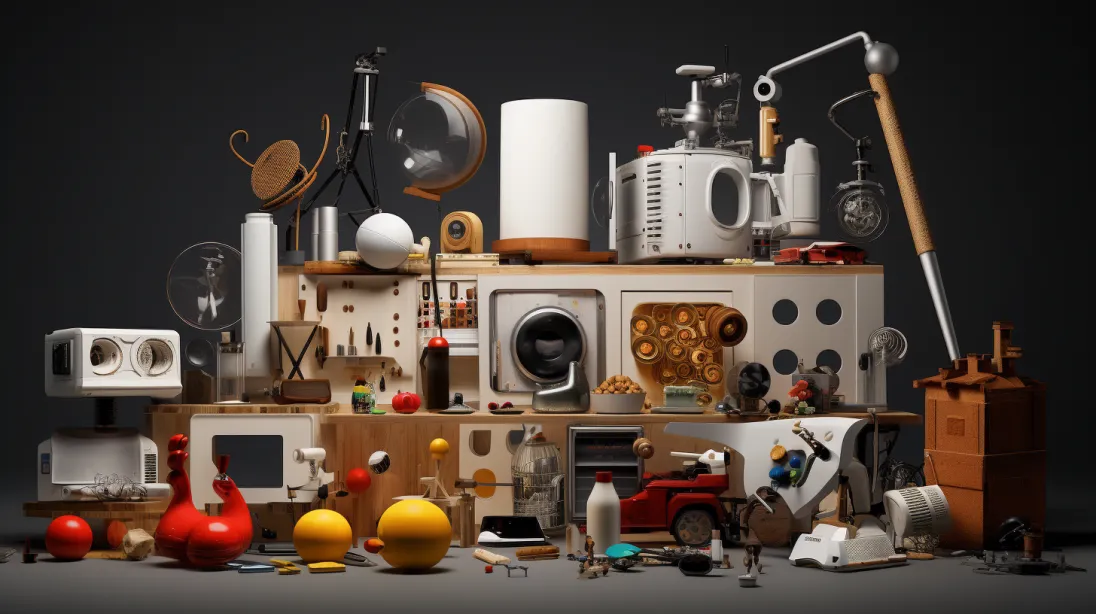
Scaling Challenges and Model Performance
One intriguing observation was the correlation between model performance and Image Recognition difficulty. Larger AI models demonstrated significant improvements in recognizing simpler images but struggled with more complex ones. Notably, the CLIP models, which combine language and vision, displayed a tendency toward more human-like image recognition.
David Mayo highlights, “Object recognition datasets have traditionally favored less-complex images. This practice has led to inflated model performance metrics that do not accurately reflect a model’s robustness or its ability to tackle complex visual tasks. Our research reveals that harder images pose a more acute challenge, causing a distribution shift that is often not accounted for in standard evaluations.”
From ObjectNet to MVT: A Journey of Progress
The research journey that began with ObjectNet has evolved into a broader exploration of Image Recognition difficulty. Unlike conventional approaches that focus solely on absolute performance, the MVT metric assesses how models perform by contrasting their responses to the easiest and most challenging images. This approach offers a new dimension for evaluating models and understanding the complexities of human perception.
The study delved into various metrics, including c-score, prediction depth, and adversarial robustness, to investigate how networks process more challenging images differently. While patterns emerged, a comprehensive semantic explanation of Image Recognition difficulty remains a subject of ongoing research.
Implications in Healthcare and Beyond
The relevance of understanding visual complexity extends to healthcare, where AI models interpret medical images such as X-rays. The diversity and difficult distribution of these images directly impacts the effectiveness of AI systems. The researchers advocate for meticulous analysis of difficulty distribution tailored for professionals to ensure AI systems meet expert standards.
Mayo and his team are also exploring the neurological aspects of visual recognition. They aim to uncover whether the brain exhibits distinct activity when processing easy versus challenging images, potentially shedding light on how our brains decode the visual world efficiently.
Toward Human-Level Performance
The journey ahead involves enhancing AI’s predictive capabilities regarding Image Recognition difficulty and identifying correlations with viewing-time difficulty to create harder or easier versions of images. While the study has made significant progress, it acknowledges limitations, particularly in separating object recognition from visual search tasks.
In conclusion, this comprehensive approach addresses the longstanding challenge of objectively assessing progress toward human-level performance in object recognition. The introduction of the Minimum Viewing Time difficulty metric opens new avenues for understanding and advancing the field of AI. With the potential to adapt this metric to various visual tasks, it paves the way for more robust, human-like performance in object recognition. This work ensures that AI models are truly tested and prepared for the complexities of real-world Image Recognition and understanding.
This study, presented at the 2023 Conference on Neural Information Processing Systems (NeurIPS), sheds light on the importance of considering image difficulty in AI benchmarking, ultimately leading to fairer comparisons between AI and human perception.