Table of Contents
Image recognition applications have been pivotal in the transformation of deep learning. Convolutional Neural Networks (CNNs) lie at the core of this revolution, excelling in capturing intricate details within images. However, CNNs demand substantial training datasets and grapple with issues like overfitting and adversarial attacks.
Ongoing research in the field is dedicated to addressing these challenges. Image recognition applications are at the forefront of innovation in the dynamic field of deep learning. Their impact extends across various domains, transforming how we interact with technology and the world around us.
From healthcare and automotive industries to surveillance and agriculture, image recognition is revolutionizing processes, improving efficiency, and enhancing decision-making.
As technology continues to advance, the potential for further expansion and refinement of image recognition applications is boundless. Researchers and developers are continuously pushing the boundaries of what is possible, unlocking new opportunities and shaping the future of this transformative technology.
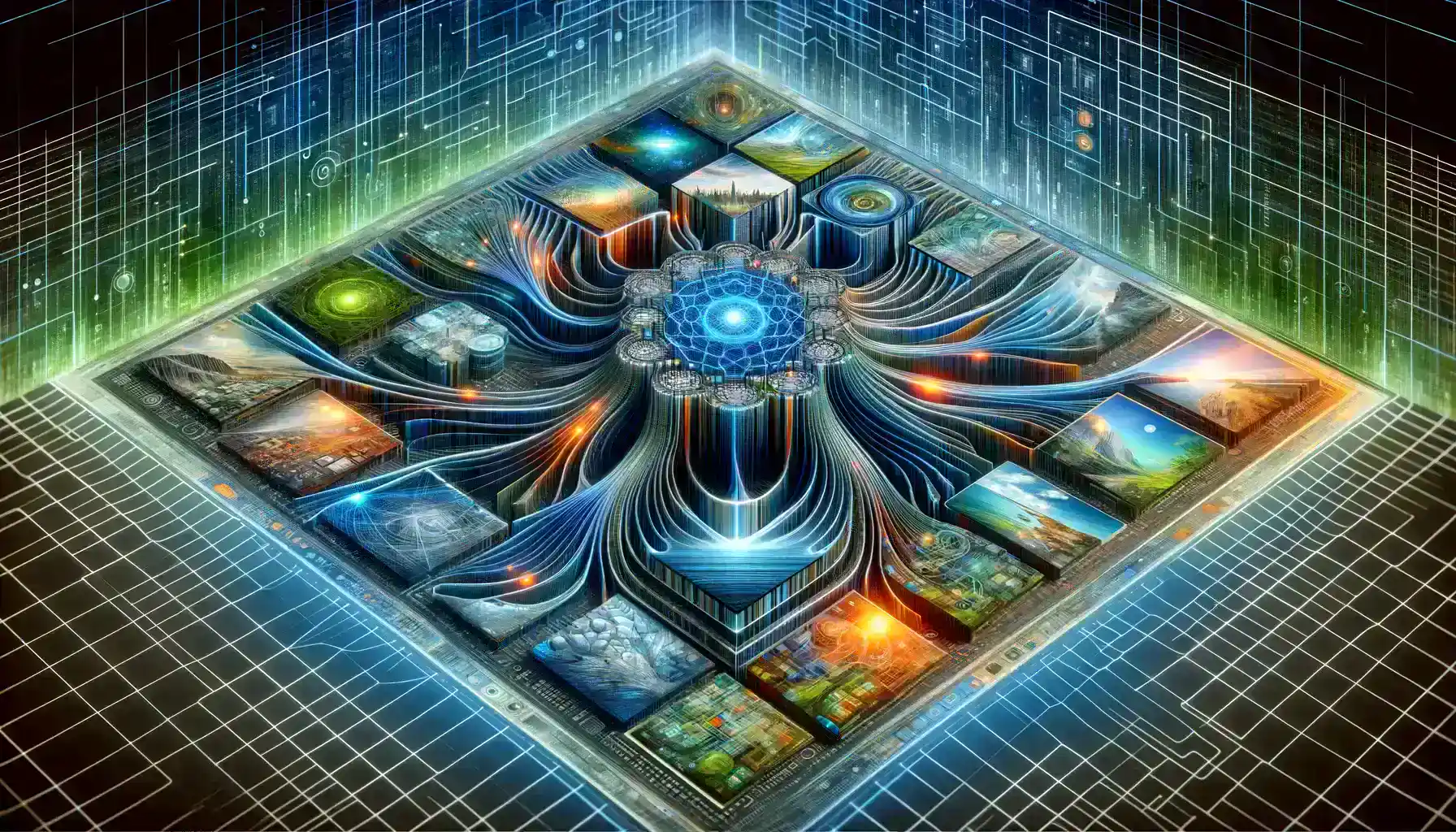
Image Recognition Applications: Facial Recognition’s Evolution and Diverse Applications:
Facial recognition technology, tracing its roots back to the 1960s, has undergone a remarkable evolution, driven by advancements in AI, IoT, and ML. Today, it finds widespread application across diverse sectors, revolutionizing various processes. Image recognition applications have played a significant role in this evolution, enhancing the capabilities and impact of facial recognition technology in fields such as security, healthcare, and more.
In the travel industry, airports use facial recognition for seamless passenger identification during departures, expediting security procedures, and enhancing travel experiences.
In healthcare, applications like Face2Gene leverage facial recognition to diagnose genetic disorders by analyzing facial features, improving rare condition identification. Social media platforms use facial recognition to enhance user experience, automatically tagging individuals in photos for engagement and personalization. Leading this advancement is the DeepFace framework, integrating models like VGGFace and FaceNet, achieving impressive accuracy, with some models nearing 99% on benchmark datasets like LFW.
Facial recognition’s future promises innovation, transforming technology interaction and social connections. Image recognition applications have been instrumental in enhancing facial recognition capabilities in these domains.
Empowering AI with Object Detection:
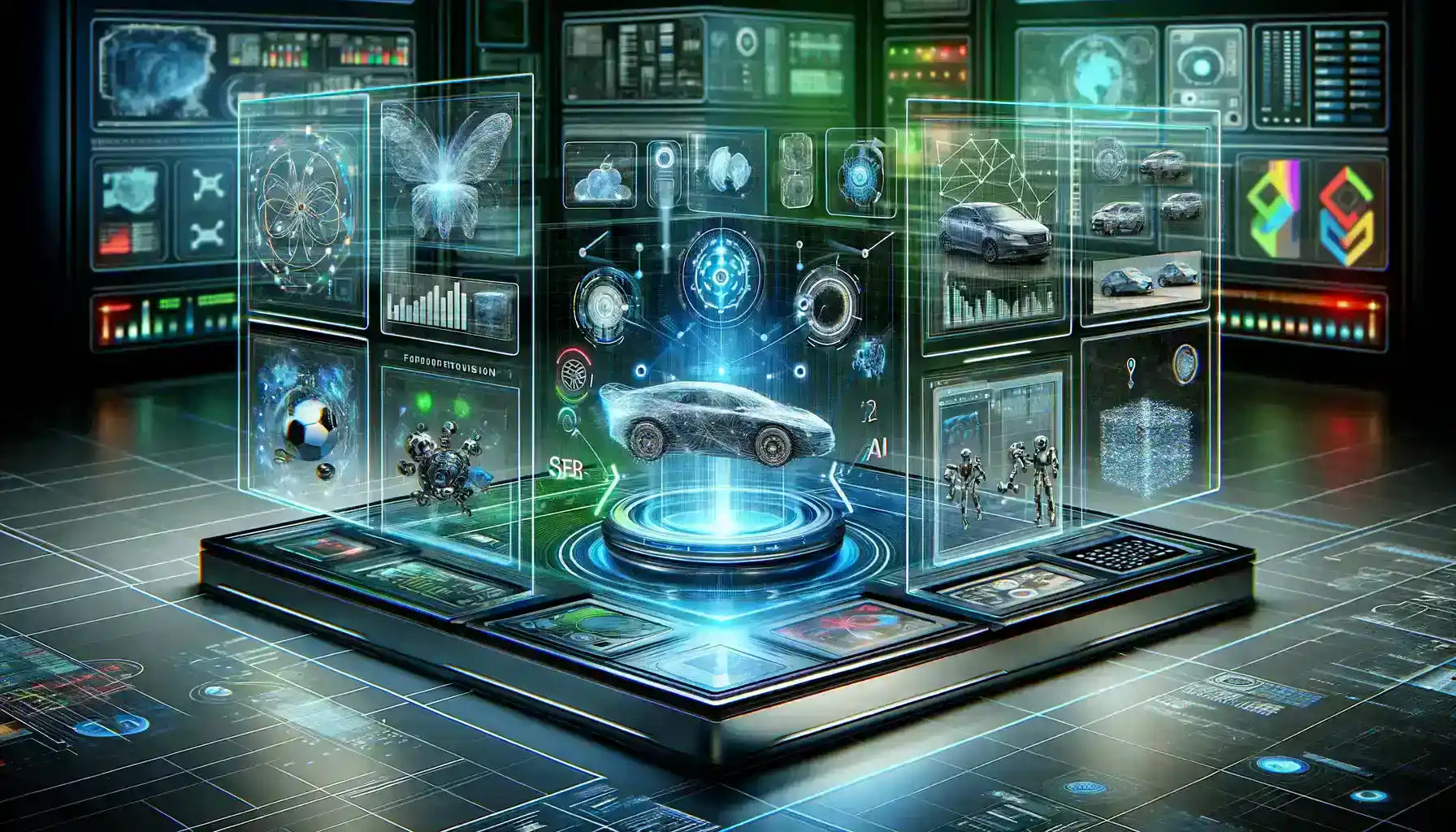
Object detection is a vital aspect of artificial intelligence, used to identify and locate objects in images with bounding boxes and categories. Its applications are diverse, from enabling self-driving cars to navigate complex environments to enhancing security through smart surveillance systems. Image recognition applications have played a significant role in advancing object detection, making it a critical technology in various domains.
Models used for object detection undergo rigorous training on extensive datasets such as COCO (Common Objects in Context), enabling them to achieve high accuracy. These models are categorized into one-stage and two-stage detectors. The RCNN family, which includes innovations like Fast-RCNN and Faster-RCNN, has been instrumental in the progress of object detection, leading to more efficient and accurate systems.
One-stage detectors like YOLO (You Only Look Once) and SSD (Single Shot Multibox Detector) are specifically designed for real-time applications, emphasizing speed and efficiency. Additionally, RetinaNet, while categorized as a one-stage detector, introduced the “Focal Loss” technique, which significantly improves accuracy when dealing with class imbalances. As object detection technology advances, it continues to drive innovations in AI, shaping the future of numerous industries.
Transformers Revolutionizing Object Detection:
Transformers, originally designed for natural language processing (NLP) tasks, have transcended their initial domain to revolutionize object detection in computer vision. Unlike Convolutional Neural Networks (CNNs), which focus on local pixel data, Transformers excel at capturing long-range dependencies and intricate patterns through self-attention mechanisms.
DETR (Detection Transformer) represents a groundbreaking model in object detection. It transforms object detection into a direct set prediction problem, eliminating the need for handcrafted components like anchor boxes. This approach streamlines the process, offering the potential for improved accuracy and efficiency in computer vision applications. Transformers, as they bridge the gap between NLP and computer vision, hold promise for the future of object detection, with image recognition applications playing a crucial role in their development.
Real-world Examples:
Surveillance: Object detection in video and real-time footage is used for theft detection, monitoring traffic violations, and identifying suspicious activities. Certainly, in the realm of surveillance, object detection plays a pivotal role in safeguarding various domains. Beyond theft detection, it assists law enforcement and security agencies in monitoring traffic violations and enhancing road safety by identifying reckless driving behaviors.
Furthermore, it acts as a vigilant sentinel in identifying suspicious activities in public spaces, contributing to the overall security and safety of communities. The real-time capabilities of object detection in surveillance continue to evolve, providing valuable insights and proactive measures for addressing potential threats and maintaining public order.
Image recognition applications are integral to the field of object detection in surveillance. They enable the system to identify and classify objects and people in real-time footage, facilitating theft detection, traffic violation monitoring, and the identification of suspicious activities. By combining image recognition with object detection, surveillance systems become more efficient and effective, contributing to enhanced security and public safety across various domains.
Automobiles: Autonomous driving systems rely on object detection to navigate and make decisions about accelerating, braking, or turning. In the automotive industry, the role of object detection in autonomous driving systems cannot be overstated. These systems heavily rely on object detection to perceive and interpret their surroundings, making critical decisions about acceleration, braking, steering, and navigation.
Image recognition applications integral to autonomous driving systems such as LiDAR, radar, and cameras, work in tandem to identify and track vehicles, pedestrians, cyclists, and road signs, ensuring safe and efficient autonomous driving. This technology represents a significant step towards the realization of self-driving cars, promising safer and more convenient transportation for the future.
Retail: In the retail sector, image recognition applications have revolutionized inventory management by enabling smart, real-time tracking of products on shelves. This not only ensures efficient restocking and reduces out-of-stock situations but also enhances the shopping experience for customers. Additionally, it facilitates cashier-less shopping experiences, where customers can simply pick up items and walk out of the store without going through a traditional checkout process. Overall, deep learning’s impact on image recognition has been revolutionary, but challenges like dataset size and adversarial attacks remain, necessitating ongoing research and development in the field.
Manufacturing: Image recognition applications play a vital role in manufacturing by enhancing object detection is used in manufacturing for quality control, ensuring that products meet specific standards. It identifies defects, irregularities, or missing components in real-time, reducing production errors. In the manufacturing industry, object detection technology is deployed for stringent quality control processes.
It operates in real-time to meticulously inspect products, ensuring they meet precise quality standards. By identifying defects, irregularities, or missing components promptly, object detection significantly reduces production errors and enhances the overall quality and reliability of manufactured goods. This not only minimizes waste but also leads to cost savings and improved customer satisfaction, making it an indispensable tool in modern manufacturing
Agriculture: Image recognition applications are a valuable asset in agriculture, aiding farmers in monitoring crop health, detecting pests, and optimizing irrigation. Drones equipped with object detection cameras efficiently survey large fields, providing valuable insights into crop conditions for precision agriculture. This data-driven approach allows farmers to make informed decisions, conserve resources, and improve crop yields, contributing to sustainable and efficient agricultural practices.
Healthcare: object detection technology extends its impact in healthcare by assisting in the analysis of various medical images, including CT scans, MRIs, and pathology slides. It plays a pivotal role in early disease detection, enabling healthcare professionals to identify anomalies, tumors, or abnormalities with high precision. This not only facilitates timely intervention but also aids in treatment planning, improving patient outcomes. Object detection applications in healthcare continue to advance, offering invaluable support in diagnostics and medical research.
Environmental Conservation: Image recognition applications are instrumental in environmental conservation efforts, particularly in tracking and protecting endangered species. Cameras equipped with object detection capabilities are strategically deployed to track and safeguard endangered species. These systems can identify and document wildlife activities, helping conservationists gather critical data for research and protection initiatives. The use of object detection in environmental conservation contributes to the preservation of biodiversity and the long-term sustainability of ecosystems.
Textile Industry: Image recognition applications are integral to quality control in fabric manufacturing. It plays a crucial role in inspecting fabrics for defects and irregularities, ensuring that the end products meet the highest quality standards. By identifying flaws such as tears, stains, or weaving errors, object detection technology helps minimize the production of substandard materials and reduces waste. This not only enhances the overall quality of textiles but also contributes to cost-efficiency and customer satisfaction in the textile manufacturing sector.
Construction: Image recognition applications enhance workplace safety through object detection technology they play a crucial role in enhancing workplace safety. It is utilized to detect and alert workers to potential hazards in real-time. This includes identifying the presence of moving machinery, vehicles, or obstacles in the construction site, helping prevent accidents and injuries. Object detection systems can provide immediate warnings to workers and equipment operators, contributing to a safer working environment. Additionally, they can be integrated with other safety measures to ensure comprehensive protection on construction sites, reducing the risk of accidents and improving overall safety standards.
Conclusion:
Deep learning, powered by Convolutional Neural Networks (CNNs), has ushered in a new era of image recognition. It has found applications in diverse sectors, from facial recognition to object detection, healthcare, and beyond. Image recognition applications have played a significant role in enhancing the capabilities and impact of deep learning in these domains. However, challenges like the need for substantial datasets and vulnerability to overfitting and adversarial attacks persist. Despite these obstacles, deep learning continues to drive innovation in image recognition, promising even greater advancements as technology evolves. The ongoing pursuit of solutions will unlock a wealth of possibilities in this dynamic field, transforming industries and shaping the future.
Related Article
Deep Learning as a Beacon for Medical Diagnosis: The Top 3 AI Tools Transforming Healthcare