Deep Learning as a Beacon for Medical Diagnosis: The Top 3 AI Tools Transforming Healthcare
Table of Contents
Introduction
The evolution of medical diagnosis is marked by a series of innovations that have progressively enhanced the physician’s ability to detect and treat disease. In this continuum of advancement, the introduction of artificial intelligence (AI), and more specifically deep learning, stands out as a pivotal milestone.
Deep learning, a sophisticated branch of machine learning, draws its inspiration from the neural networks of the human brain, emulating its ability to learn from and interpret vast amounts of data. As we stand on the cusp of a new era in medical care, deep learning has become a beacon, guiding the way toward more effective, efficient, and personalized Healthcare.
This article aims to explore the transformative effects of the top three deep-learning AI tools on medical diagnosis, casting a light on how they are reshaping the landscape of healthcare.
The Impact of Deep Learning on Medical Diagnosis
With the revolutionary role of deep learning in medical diagnosis, one must first grasp its foundational impact on the field. Traditional diagnostic processes, heavily reliant on the acumen and experience of medical professionals, have been augmented by deep learning’s capacity to analyze and learn from data on a scale and at a speed that is beyond human capability.
Deep learning algorithms, through their sophisticated pattern recognition capabilities, are able to identify subtle nuances in medical images and patient data that may elude even the most trained eyes. This has profound implications for early detection of diseases, where the slightest indicator can make the difference between a treatable condition and a life-threatening one.
For instance, in oncology, deep learning tools can sift through thousands of radiology images to detect early signs of tumors that are imperceptible to the human observer. The integration of deep learning in medical diagnosis also promises to enhance the patient experience.
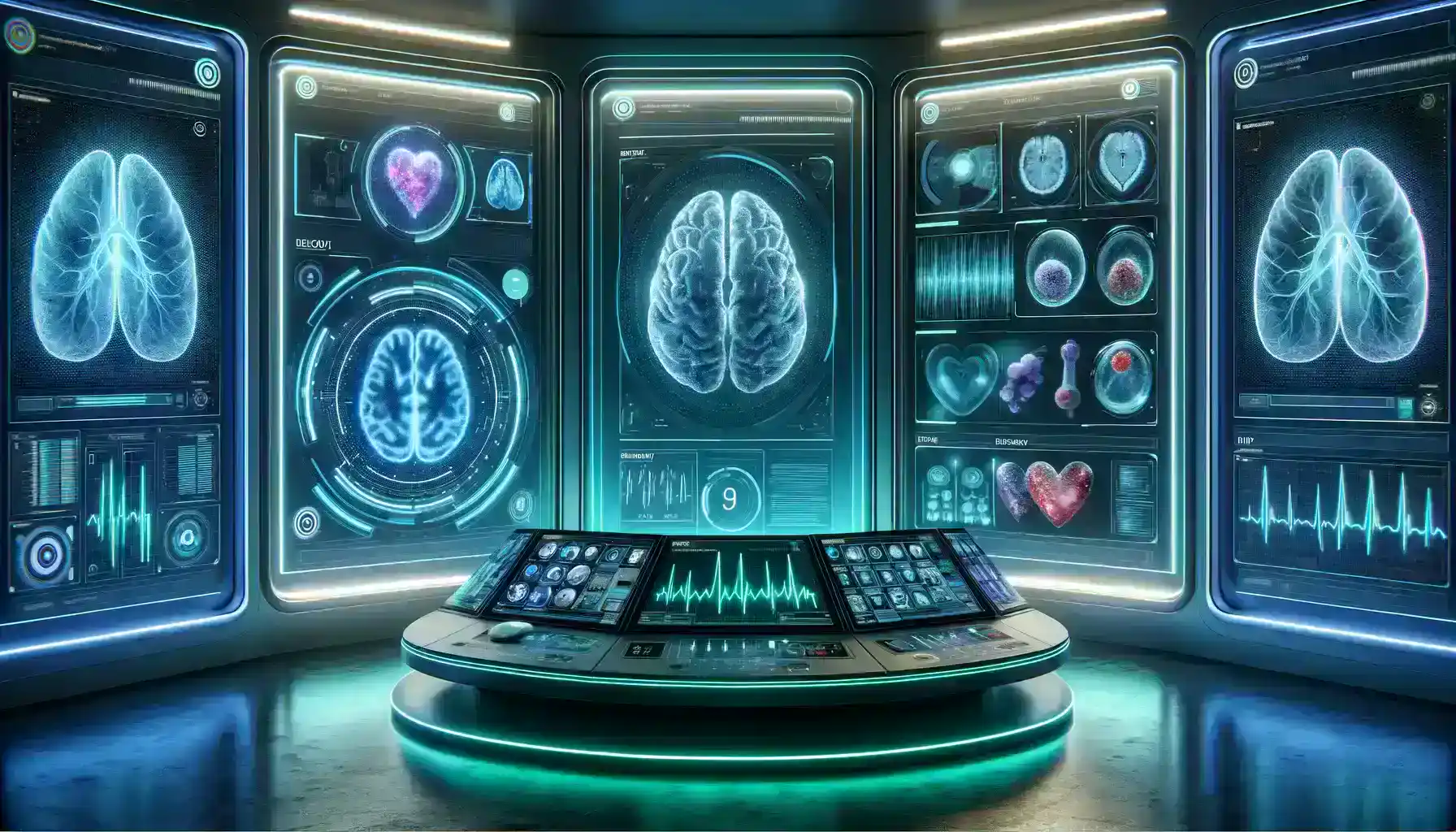
These AI tools continue to learn and improve over time, the hope is that they will contribute to a continuous increase in the quality of patient outcomes, making healthcare more responsive and effective.
This technology is not just another tool in the diagnostic arsenal; it represents a paradigm shift in how the data is processed, understood, and applied. As we examine the top three AI tools harnessing this technology, it becomes clear that the future of medical diagnosis is not just bright; it is intelligent.
1. Imaging Diagnostic Tools: Enhanced Radiology
In the field of medical diagnosis, deep learning-powered imaging diagnostic tools have significantly advanced the field of radiology. These tools, equipped with advanced algorithms, are proficient at interpreting images, such as X-rays, MRIs, and CT scans, with a level of accuracy that was previously unattainable.
Trained on extensive datasets, these AI systems excel at identifying and analyzing intricate patterns within the images, providing invaluable insights that could be overlooked by human eyes.
This level of precision in medical diagnosis is instrumental in planning the appropriate intervention strategies, especially in complex cases such as brain tumors where early detection can dramatically improve prognosis.
NeuroScan AI is a deep learning-powered tool designed to analyze neurological imaging. It’s engineered to discern the slightest variances in brain scans, aiding in the detection of conditions such as stroke, brain hemorrhages, and tumors.
NeuroScan AI’s deep learning model has been trained on a diverse collection of neurological scans, allowing it to recognize early signs of abnormalities. In a notable case, it detected an early-stage ischemic stroke in a patient by identifying the reduced blood flow in the brain, which appeared as subtle changes in the scan that were almost imperceptible.
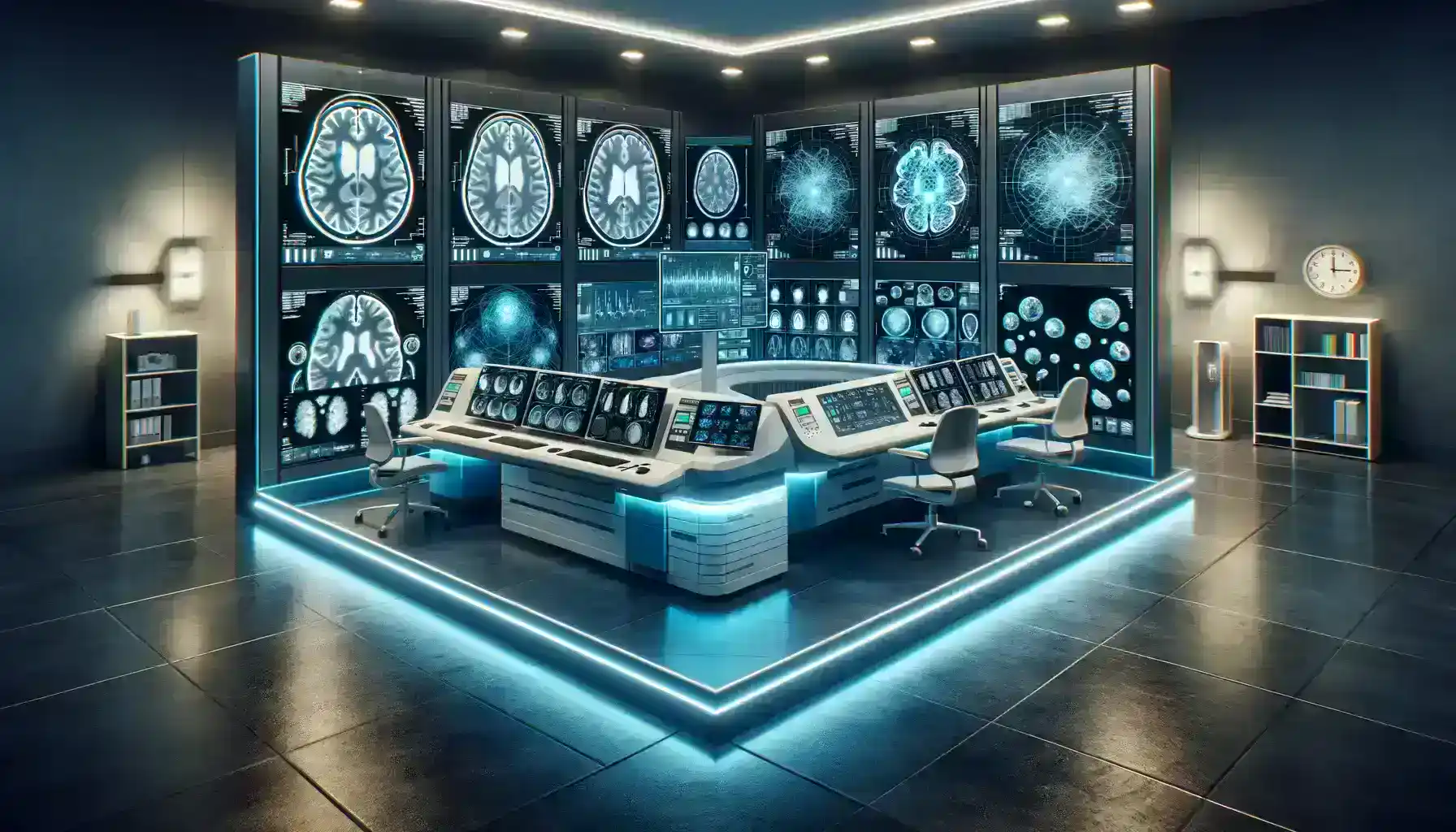
2. Pathology Diagnostic Tools: Precision Pathology
The meticulous examination of tissue samples is crucial in pathology, and deep learning tools like PathoPrecise AI bring a new level of precision to this field. These tools assist pathologists in diagnosing diseases by analyzing biopsy samples down to the cellular level, often providing crucial second opinions.
This transformation has made the field more accurate, more nuanced, and significantly more efficient. Deep learning, especially convolutional neural networks (CNNs), has been a game-changer for pathology. These networks are incredibly effective at processing visual data and have been instrumental in advancing the analysis of medical images, including pathology slides.
PathoPrecise AI stands out for its ability to analyze histopathological images with exceptional detail. It scrutinizes tissue samples at the cellular level, examining cell morphology, staining patterns, and the architecture of tissue sections. This AI tool is trained on extensive datasets of annotated pathology images, which include a range of conditions from benign to malignant lesions.
The implementation of PathoPrecise AI in clinical settings has dramatically improved the speed and accuracy of medical diagnosis. Pathologists can now rely on this tool to perform a preliminary analysis of samples, flagging potential areas of concern.
The potential for such tools to learn and improve over time promises even greater advances in the diagnosis and understanding of a wide range of diseases. It underscores the transformative impact that such tools have on the medical diagnosis process, offering a glimpse into the future where AI and human expertise work in concert for the benefit of patient care.
3. Predictive Diagnostic Tools: Forecasting Health Outcomes
Predictive diagnostic tools harness the predictive power of deep learning to forecast potential health events. PredictHealth AI epitomizes this category by utilizing personalized data to anticipate future medical conditions. These tools are capable of synthesizing vast amounts of personal health data to predict future medical events, effectively shifting the healthcare paradigm from reactive to proactive.
The role of predictive diagnostic tools is to utilize algorithms to analyze and interpret complex data. PredictHealth AI, a leading example in this field, leverages deep learning to provide personalized medical diagnosis and prognosis.
PredictHealth AI is a sophisticated deep-learning framework that can process and learn from an extensive array of data points. It examines a patient’s complete medical history, genetic markers, and lifestyle choices, and draws correlations between these factors and potential health risks. This analysis includes data from electronic health records (EHRs), genomic databases, and even wearable health monitoring devices.
The advantage of using tools like PredictHealth AI in medical diagnosis is manifold. By identifying risks early, they enable preventive care, which can be less invasive, more cost-effective, and can lead to better health outcomes. Additionally, they can help to personalize patient care, ensuring that interventions are tailored to the individual’s specific risk profile.
Case Study
Real-time Monitoring and Diagnosis in Cardiology with CardioTrack AI
Cardiovascular diseases (CVDs) are the number one cause of death globally, and early detection is key to improving patient outcomes. To address this, a leading tech company specializing in medical diagnosis devices partnered with the cardiology department of a renowned hospital. The partnership aimed to leverage advanced AI technology for better heart disease monitoring and management.
Challenge: The primary challenge in cardiology is the detection and diagnosis of conditions like arrhythmias, which are often sporadic and asymptomatic until they become severe. Traditional methods for monitoring such conditions require patients to be hooked to machines, limiting mobility, and typically only capturing a snapshot of heart activity rather than providing continuous surveillance.
Solution: The development of CardioTrack AI, a wearable device equipped with sensors and powered by deep learning algorithms. This device is capable of not only monitoring heart rhythms in real time but also of analyzing the data on the fly to identify anomalies that may indicate the onset of arrhythmias or other heart-related issues.
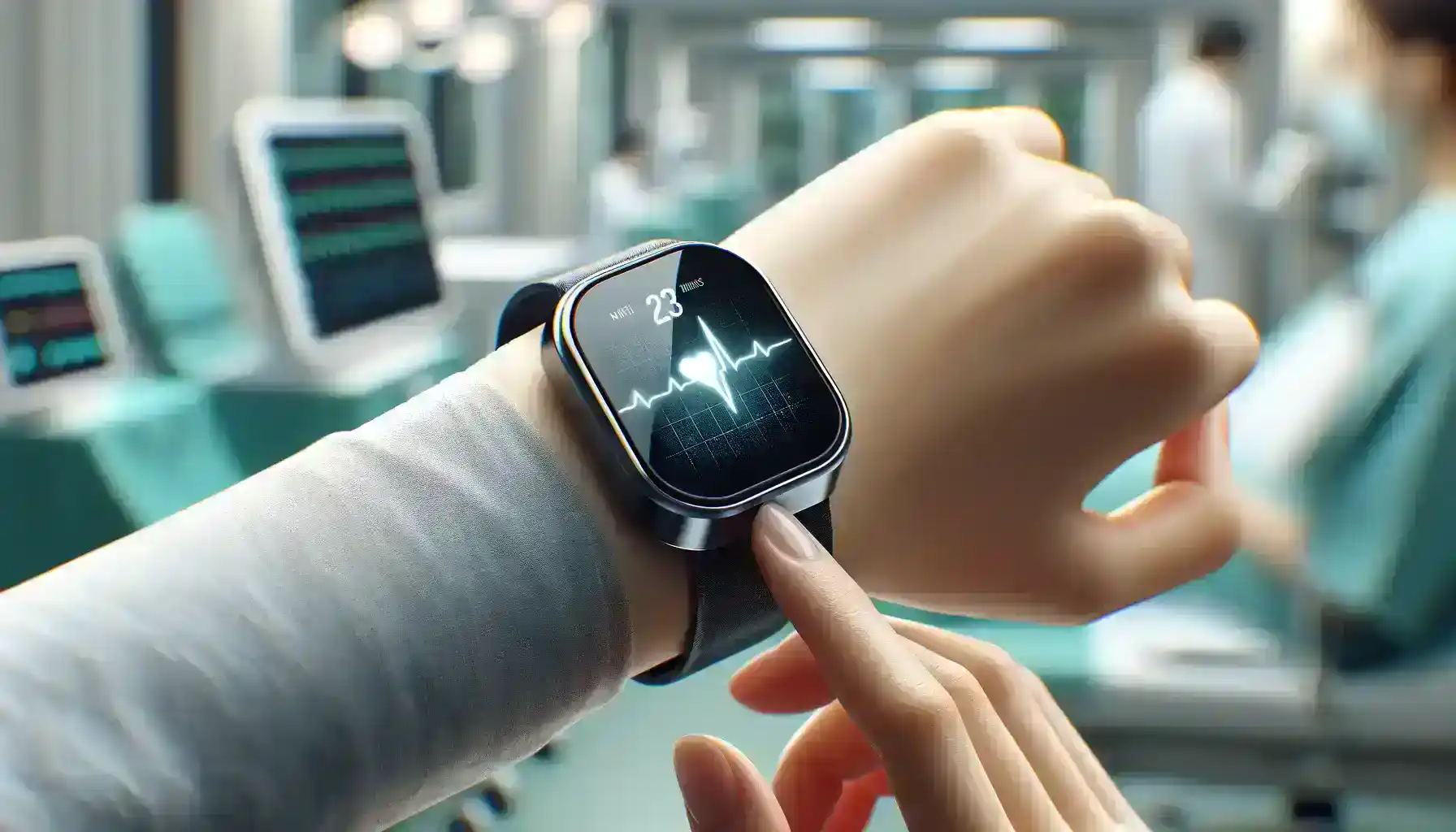
Technology Implementation: CardioTrack AI uses a combination of electrocardiogram (ECG) sensors and accelerometers to monitor the patient’s heart activity continuously. The device is discreet and comfortable, designed to be worn 24/7, and it transmits data wirelessly to a secure cloud platform.
Results: CardioTrack AI was provided to a group of high-risk patients who had a history of cardiac issues but no current diagnosis of arrhythmias. The device detected irregular heartbeats consistent with AFib in several patients. These detections were previously undiagnosed due to the intermittent nature of their symptoms.
CardioTrack AI demonstrated that real-time monitoring and AI-driven analysis could transform cardiac care by facilitating early detection and intervention. As a result, the tool not only saved lives but also reduced the burden on healthcare systems by preventing acute cardiac events. This case study serves as a testament to the potential of AI in medical diagnosis and continuous patient care, especially for chronic conditions like heart disease.
Conclusion
The integration of deep learning AI tools into the arena of medical diagnosis marks a significant leap forward in healthcare. The case study of CardioTrack AI exemplifies the transformative potential of these technologies. By enhancing radiology, pathology, and cardiology with AI-powered solutions, medical professionals can detect diseases earlier, make more accurate diagnoses, and offer personalized treatment plans.
NeuroScan AI’s ability to detect neurological abnormalities and CardioTrack AI’s real-time monitoring of cardiac health demonstrate how continuous and automated analysis can lead to lifesaving interventions. Similarly, PathoPrecise AI’s precision in identifying minute pathological details showcases the critical role of AI in diagnosing complex diseases like cancer with greater confidence.
These AI tools represent the pinnacle of current deep learning applications in healthcare, but they also pave the way for future innovations. As AI technology continues to advance, it promises even greater capabilities and potential applications.
However, while these advances are impressive, they also raise considerations about ethical implications, data privacy, and the need for robust training datasets to ensure AI systems can serve diverse populations effectively. Addressing these challenges is essential for the responsible deployment of AI in healthcare.
Deep learning stands as a beacon for medical diagnosis, shining a light on the possibilities of early detection, accurate diagnosis, and effective treatment. As healthcare systems continue to adopt and integrate these AI tools, the future of medical diagnosis looks bright, with the promise of improved patient outcomes and a new standard of care in the digital age.
Featured Insights
Integrating Real-Time Weather Data into Your Travel and Navigation App
The Future is Now: AI and ML Technologies Reshaping Healthcare
Introduction to Digital Twin Technology in Manufacturing with Unprecedented Efficiency and Innovation
Adobe’s Firefly AI: New Tools for Photoshop and Illustrator
Introducing Meta Imagine Me: Your Digital Doppelganger
AI in Gaming is Transforming the Industry