Table of Contents
Recent advancements due to “Nuclear Reactor Physics” artificial intelligence (AI) and machine learning (ML) have sparked significant interest among nuclear engineers. However, the lack of specific benchmark exercises for applying AI and ML techniques in nuclear engineering analyses hinders their practicality and widespread adoption.
Advancing Nuclear Reactor Physics through AI and ML Initiatives
To address nuclear reactor physics issue and contribute to the development of future-generation nuclear systems and innovative solutions, the Task Force on Artificial Intelligence and Machine Learning for Scientific Computing in Nuclear Engineering was formed within the Expert Group on Reactor Systems Multi-Physics (EGMUP) of the Nuclear Science Committee’s Working Party on Scientific Issues and Uncertainty Analysis of Reactor Systems (WPRS).
This initiative aligns with the NEA’s strategic objective of establishing a robust scientific and technical foundation for the advancement of nuclear reactor physics technologies.
The main objective of the Task Force is to create benchmark exercises that will concentrate on significant AI and ML tasks, encompassing diverse computational domains of interest, ranging from individual physics to multi-scale and multi-physics.
ML techniques, particularly AI, have gained significant importance in various sub-disciplines of nuclear science and engineering. These include in-core fuel management, reactor safety, flow regime prediction, and radiation protection. The utilization of ML methods has enabled the creation of optimal core loading maps, enhanced safety measures in nuclear reactors, and facilitated the rapid comprehension of flow data.
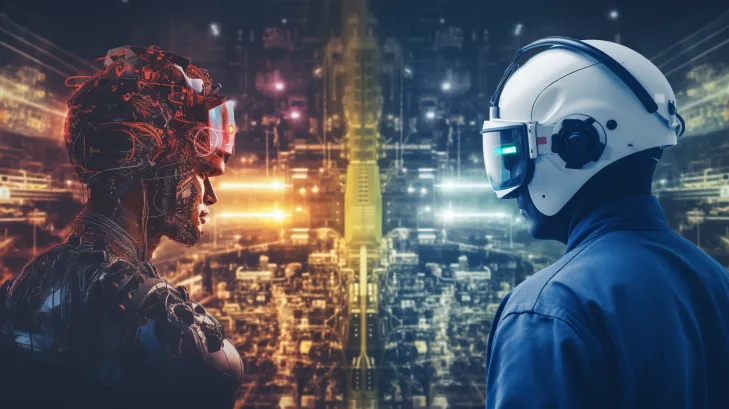
Advancements in Predicting Critical Heat Flux through AI and ML
The successful launch of a comprehensive benchmark for nuclear reactor physics on AI and ML to predict Critical Heat Flux (CHF) marks a significant milestone. CHF is a crucial factor in boiling systems, as it represents the limit beyond which wall heat transfer decreases significantly. This is commonly referred to as critical boiling transition, boiling crisis, departure from nucleate boiling (DNB), or drying out, depending on the operating conditions.
In heat transfer-controlled systems, such as nuclear reactor cores, CHF can lead to a significant increase in wall temperature, which can accelerate wall oxidation and potentially cause fuel rod failure. Despite being an important design limit criterion for safe nuclear reactor physics operation, accurately predicting CHF is challenging due to the complexities of local fluid flow and heat exchange dynamics.
The IAEA organized a Technical Meeting on Artificial Intelligence for Nuclear Technology and Applications in 2021. The meeting aimed to create a global platform for discussing and promoting collaboration in nuclear reactor physics science, technology, and applications, as well as radiation protection, nuclear security, and safeguards verification.
Exploring AI and ML Integration for Enhanced Nuclear Reactor Safety
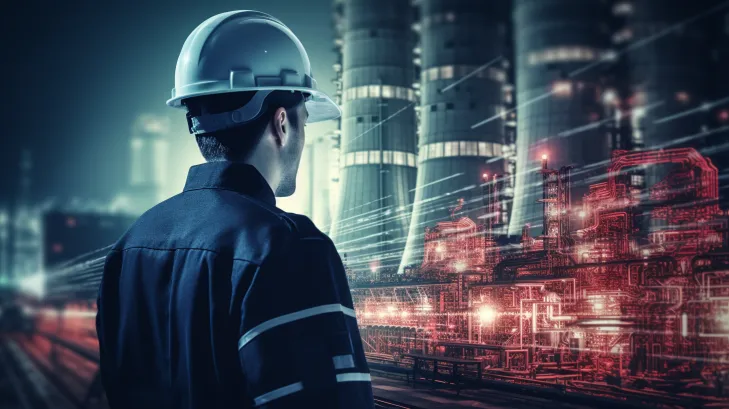
The primary goal was to determine the priorities of nuclear reactor physics for future activities in these areas and how the IAEA can assist in their implementation. Additionally, the meeting was a chance to examine the ethical implications of the intersection of artificial intelligence and nuclear technologies. This was the first meeting of its kind.
The existing CHF models rely heavily on empirical correlations that have been developed and validated for a particular application case domain. To enhance the CHF modeling, AI and ML techniques are being employed in this benchmarking exercise, which utilizes a comprehensive experimental database provided by the US Nuclear Regulatory Commission (NRC).
This exercise aims to improve the modeling and enhance our understanding of safety margins, which could lead to new opportunities for design or operational optimizations.
The commencement of phase 1 for the CHF benchmark took place during the kick-off meeting on 30 October 2023. A total of 78 participants from 48 institutions across 16 countries attended this significant event. The strong participation highlights the deep interest and dedication of the global scientific community in incorporating AI and ML technologies into the field of nuclear engineering.
The Task Force’s ultimate objective is to utilize the knowledge gained from the benchmarks and extract valuable lessons that can be used to establish guidelines for the future implementation of AI and ML in scientific computing within the realm of nuclear engineering.