Table of Contents
AI Training Datasets have been the backbone of machine learning and artificial intelligence development. However, the field constantly evolves, and the need for high-quality and ethically sourced data has never been greater. In this article, we will explore the latest advancements in AI Training Datasets, focusing on Pile v2 and how it is revolutionizing the industry.
AI Training Datasets: Fueling Machine Learning Advancements
AI Training Datasets are collections of labeled information used to teach machine learning algorithms how to perform specific tasks. These datasets consist of input data paired with corresponding desired outputs, serving as a foundation for training AI models.
Crucial Role in Machine Learning
They are pivotal in machine learning, enabling algorithms to learn patterns, make predictions, and perform tasks accurately. The quality and diversity of the data directly influence an AI system’s performance. High-quality datasets result in more robust and reliable models.
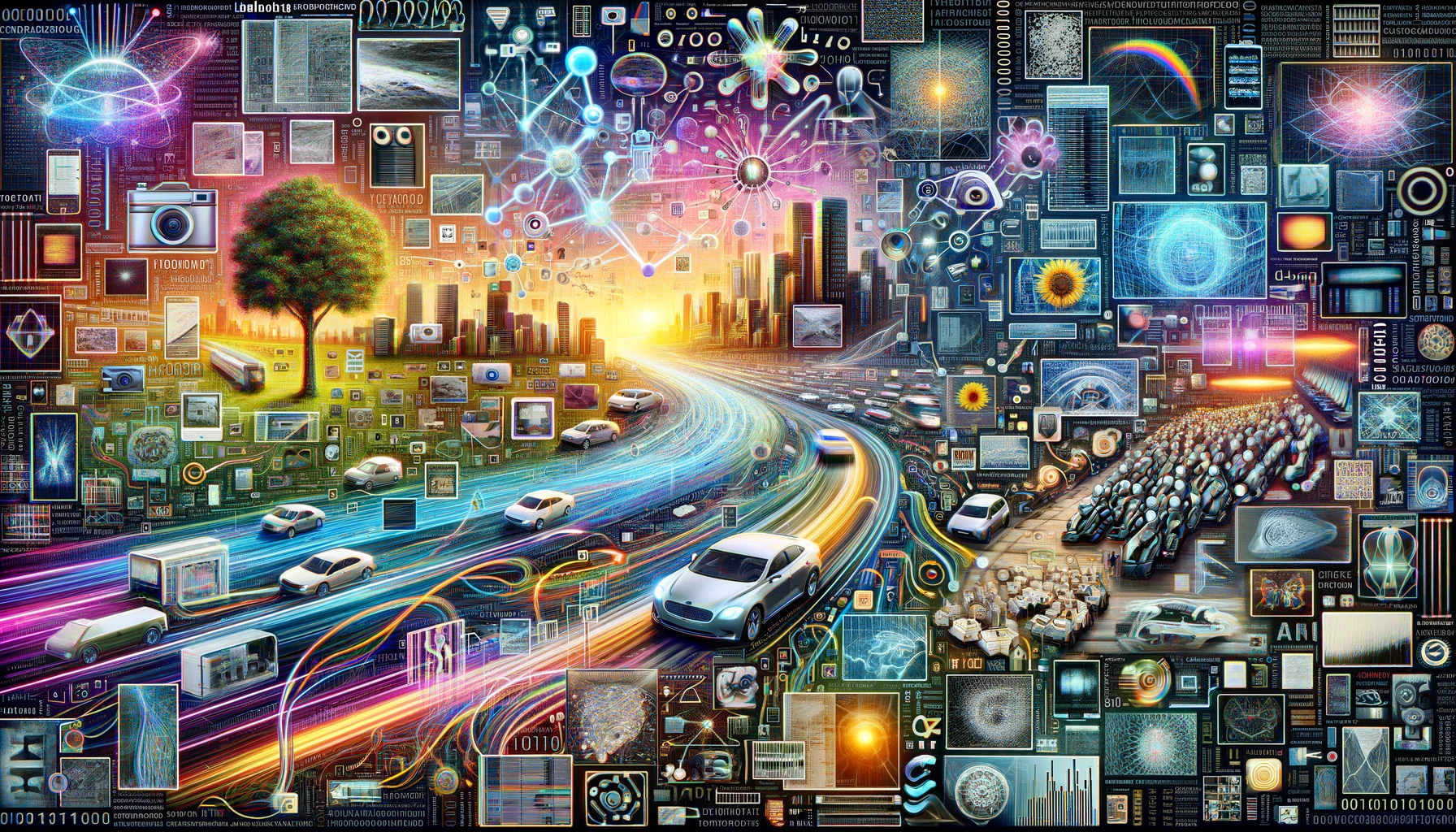
Significance of Data Quality and Ethics
The quality of AI Training Datasets is paramount. Biased, incomplete, or inaccurate data can lead to biased and unreliable AI models, which may perpetuate unfairness and discrimination. Ethical considerations in dataset creation, such as privacy preservation and fairness, are essential to ensure AI systems benefit society without harm.
AI Projects Relying on Training Datasets
Image Recognition: AI systems used in image recognition heavily rely on datasets containing millions of labeled images. For instance, the ImageNet dataset with over 14 million images has been instrumental in advancing computer vision.
Natural Language Processing (NLP): NLP models like GPT-3 are trained on extensive text datasets, allowing them to understand and generate human-like text. The Common Crawl dataset and Wikipedia dumps are fundamental resources for NLP.
Self-Driving Cars: Autonomous vehicles use training datasets comprising sensor data and annotated images to learn how to navigate safely and make real-time decisions on the road.
Medical Diagnostics: AI models for medical image analysis, like detecting diseases from X-rays or MRIs, depend on datasets of medical images and corresponding diagnoses.
The Evolution of AI Training Datasets
Challenges and Limitations:
Traditional AI training datasets have faced several challenges and limitations. They often suffer from biases, lack diversity, and struggle to keep up with the ever-evolving data needs of modern AI models. For instance, datasets used for natural language processing may have biases based on the sources they were collected from, leading to biased AI outputs.
Introduction to Pile v2 and Its Key Features
Pile v2 represents a significant leap in addressing these limitations. It is a massive, diverse, and constantly updated dataset that encompasses a wide range of text from the internet. One of its key features is its size, as it contains an unprecedented amount of data, enabling AI models to learn from a broader context. Additionally, Pile v2 is designed to be continuously updated, ensuring that AI models stay relevant and up-to-date.
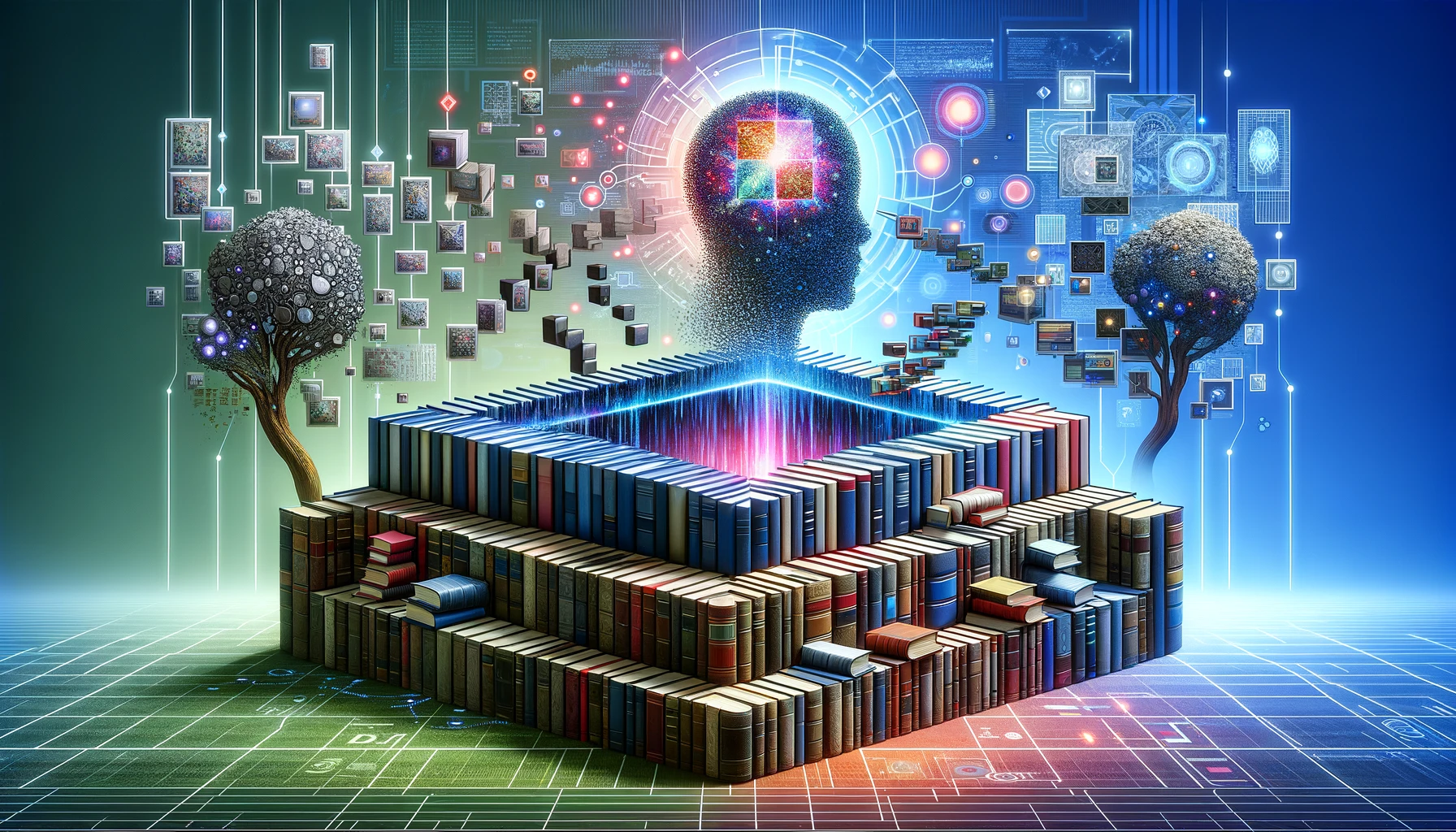
How Pile v2 Addresses Shortcomings
Pile v2 tackles the shortcomings of previous datasets through its diversity and scale. By including text from various sources, it reduces biases and improves the robustness of AI models. It also helps AI systems generalize better by exposing them to a broader spectrum of language and topics, mitigating issues like overfitting.
Applications and Success Stories:
The impact of Pile v2 can be seen in various real-world applications. For instance, AI-powered chatbots using Pile v2 can provide more nuanced and context-aware responses, improving customer service. In healthcare, Pile v2 aids in better understanding medical literature and assisting in diagnosis. Moreover, Pile v2 has enhanced the performance of AI models in language translation, making them more accurate and adaptable to different dialects.
Boosting Data Quality with Pile v2: A Game-Changer for AI Training Datasets
Pile v2:
An Overview Pile v2, the latest iteration of the renowned AI training dataset, promises substantial advancements in data quality. It addresses critical issues like noise, bias, and irrelevance, making it a cornerstone for AI development. Pile v2 leverages a refined curation process to ensure the highest standards of data accuracy and relevance.
Ensuring Data Accuracy and Relevance:
Pile v2 employs a multi-layered approach to guarantee data quality. First, it incorporates extensive human review, where experts meticulously assess and validate data entries. This step reduces the likelihood of erroneous information entering the dataset. Second, machine learning algorithms identify and flag low-quality or irrelevant data points. This combination of human expertise and AI-powered filtering results in a robust dataset.
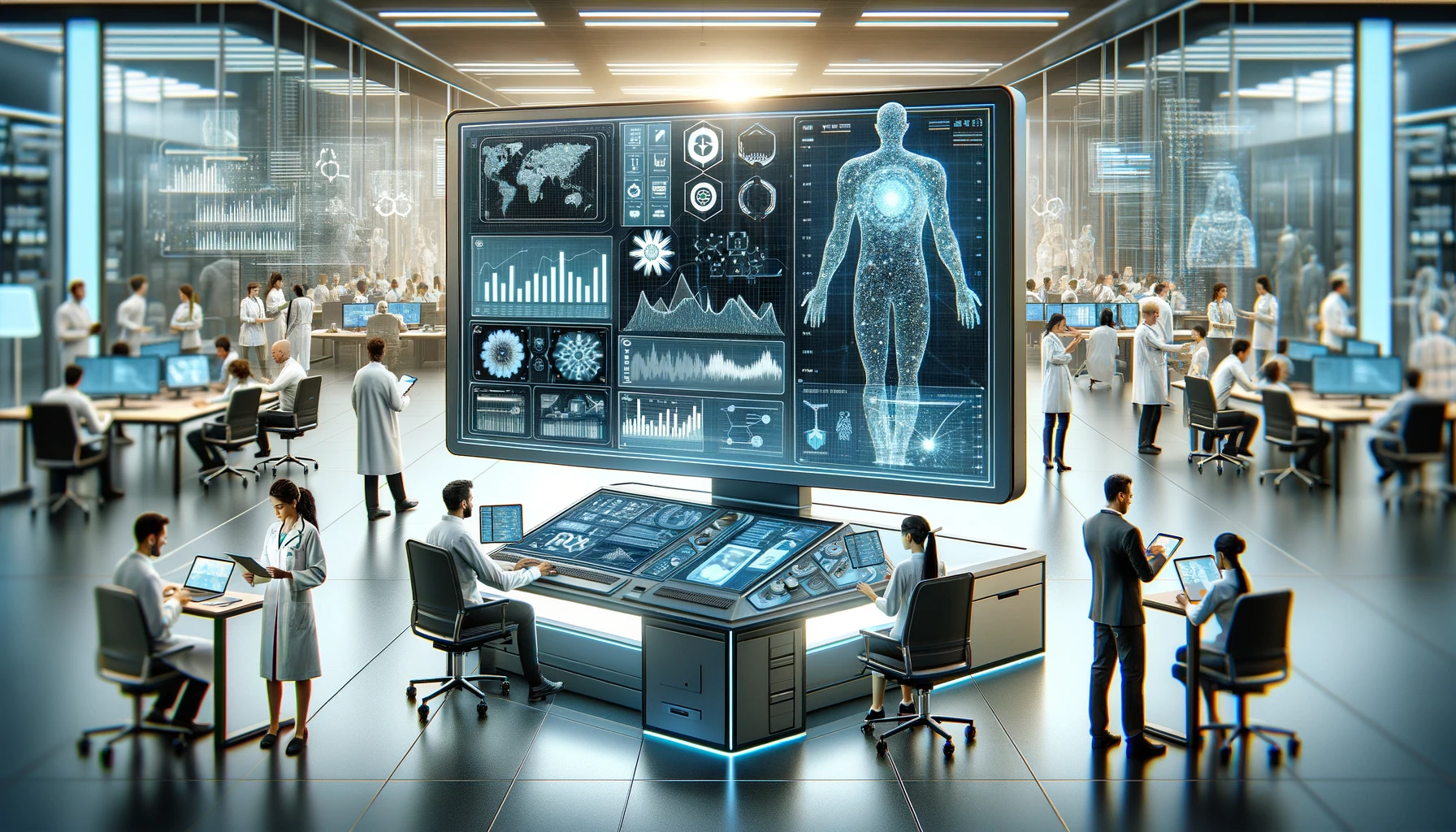
Use Cases and Industries Benefiting from Pile v2:
Pile v2’s enhanced data quality finds applications across various industries. In healthcare, it aids in developing more accurate diagnostic AI models. In finance, it improves fraud detection algorithms by providing cleaner transaction data. Autonomous vehicles benefit from precise mapping data, and natural language processing models become more reliable when trained on Pile v2.
Comparative Analysis with Previous AI Training Datasets:
When compared to earlier AI training datasets, Pile v2 stands out. Previous datasets often suffered from inconsistencies, outdated information, and inherent biases. In contrast, Pile v2’s meticulous curation and rigorous quality control processes result in a higher signal-to-noise ratio, reducing the risk of models learning from spurious correlations.
For instance, while older datasets may have contained outdated facts or biased perspectives on certain topics, Pile v2 aims to provide the most up-to-date, balanced, and relevant data, ensuring that AI models are more accurate and ethical in their decision-making.
Ethical Considerations in AI Training Data
It plays a pivotal role in shaping the performance and behavior of artificial intelligence systems. However, they also raise significant ethical challenges. One of the key concerns is bias, where AI models can inherit and perpetuate existing prejudices present in the training data. For instance, if an AI system is trained on historical data that contains gender or racial biases, it may inadvertently produce discriminatory outputs in real-world applications.
Pile v2’s Approach to Ethical Data Sourcing and Curation:
To address these ethical challenges, Pile v2, a cutting-edge AI dataset, takes a proactive approach to ethical data sourcing and curation. It employs rigorous processes to identify and mitigate biases in its training data, ensuring that the data used to train AI models is as fair and unbiased as possible. Pile v2 also emphasizes transparency in its data collection methods, allowing users to understand the origins and potential biases of the data.
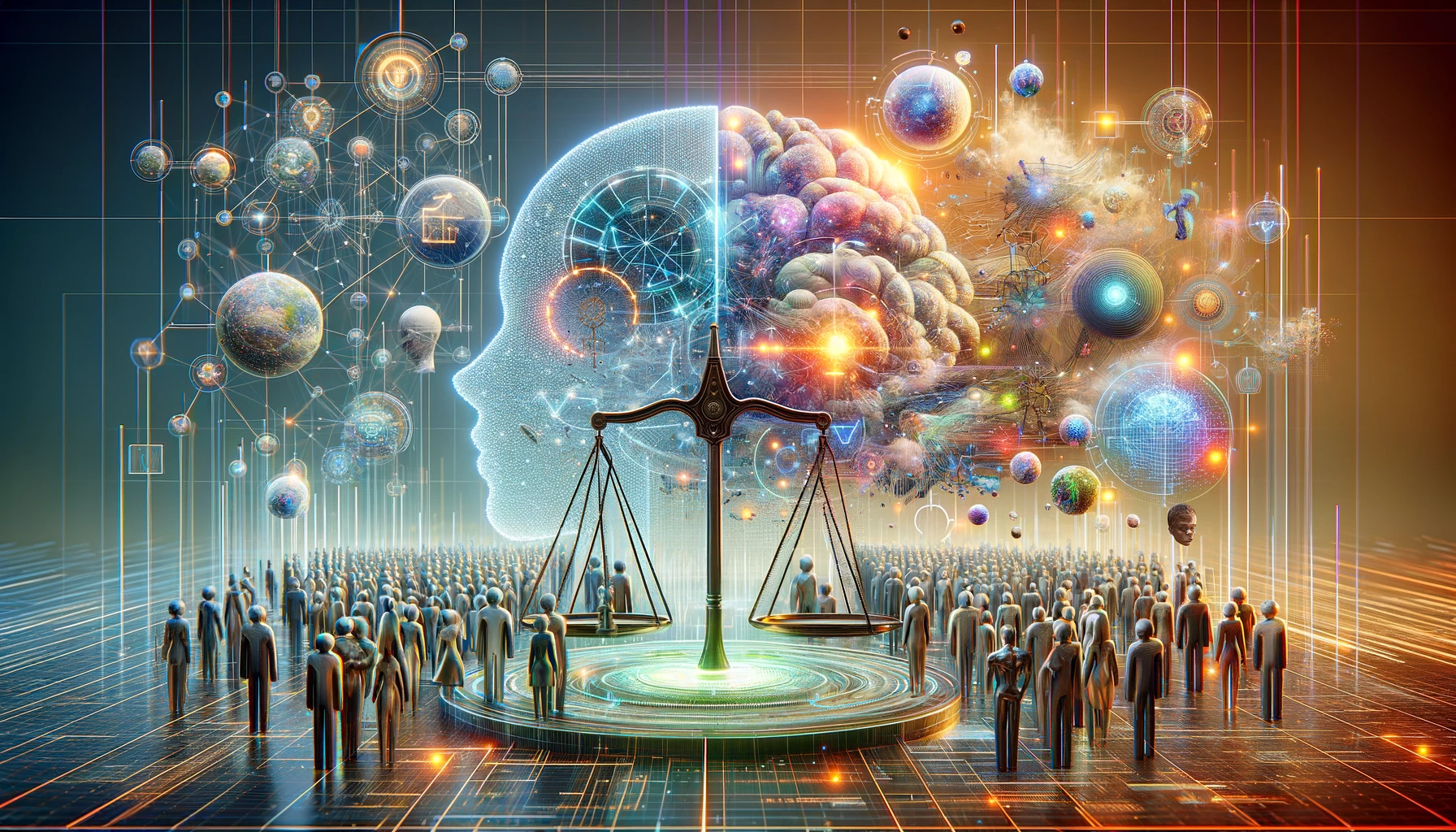
Ensuring Fairness, Diversity, and Inclusivity in AI Datasets:
To ensure fairness, diversity, and inclusivity in AI datasets, it is essential to actively monitor and curate the data. For instance, when creating datasets for natural language processing tasks, care should be taken to include diverse language variants, dialects, and cultural perspectives. This prevents AI systems from favoring one demographic over others and promotes a more inclusive and equitable use of technology.
Case Studies Highlighting the Importance of Ethical AI Data:
In a case study involving AI-driven hiring platforms, biased training data led to the system unfairly favoring male candidates over female ones, perpetuating gender disparities. Similarly, in the healthcare sector, an AI diagnostic tool trained on predominantly Caucasian patient data struggled to provide accurate diagnoses for patients from other racial backgrounds. These instances underscore the critical importance of ethical AI data to avoid discrimination and ensure equitable outcomes.
AI Training Datasets Transforming Industries
Healthcare: AI Training Datasets Revolutionize Medical Diagnosis:
It has ushered in a transformative era in healthcare by significantly enhancing medical diagnosis. These datasets include vast collections of patient records, medical images, and genomic data, enabling machine-learning models to identify diseases more accurately and quickly than ever before. For instance, in radiology, datasets with annotated images have enabled AI algorithms to assist radiologists in detecting abnormalities such as tumors, improving diagnostic accuracy and patient outcomes. Furthermore, genomic datasets have empowered AI to predict an individual’s susceptibility to certain diseases, allowing for personalized treatment plans.
Autonomous Vehicles: High-Quality Datasets Drive Self-Driving Cars:
The development of self-driving cars relies heavily on AI training datasets that simulate real-world driving scenarios. These datasets consist of millions of miles of sensor data, including images, lidar scans, and GPS information. By training on diverse and high-quality datasets, autonomous vehicle algorithms can learn to navigate complex environments safely. For instance, companies like Waymo have collected extensive datasets to train their self-driving vehicles, making them safer and more capable of handling various road conditions.
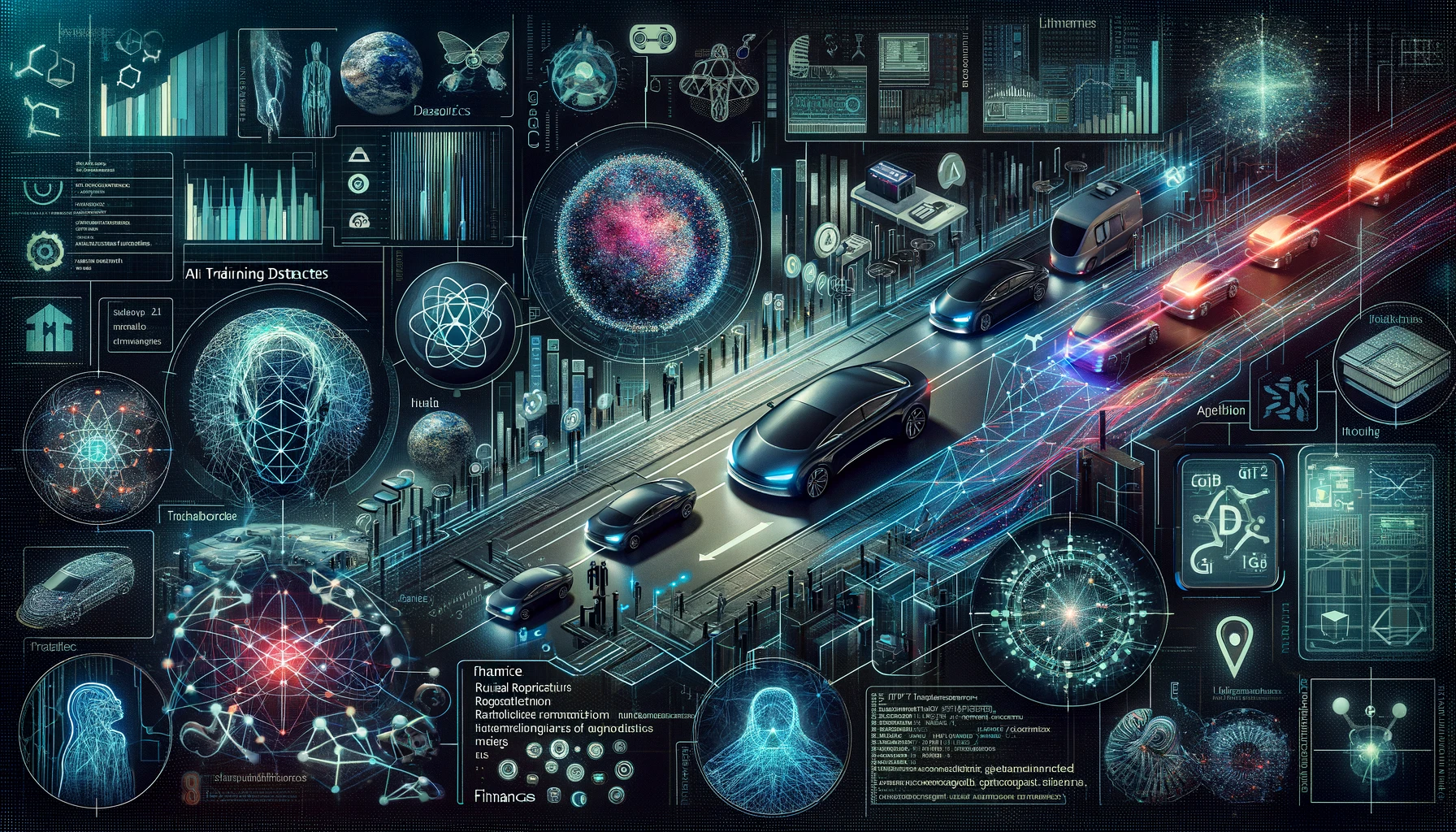
Natural Language Processing: Advancements in Language Understanding:
It has been instrumental in advancing natural language processing (NLP). Massive text corpora, like Wikipedia or news articles, serve as training data for language models such as GPT-3. These datasets enable machines to understand and generate human-like text, improving applications like chatbots, language translation, and content generation. Through continuous fine-tuning on diverse datasets, NLP models have achieved remarkable progress in tasks such as sentiment analysis, text summarization, and question-answering systems.
Finance: Ethical AI Data in Fraud Detection:
The finance industry relies on AI training datasets to bolster fraud detection mechanisms. Ethical AI datasets include transaction histories, customer profiles, and fraud case records. By analyzing these datasets, AI systems can detect fraudulent activities, such as unauthorized transactions or identity theft, in real time. For example, credit card companies utilize AI-powered fraud detection models that continuously learn from transaction data to identify suspicious patterns and protect customers from financial fraud. Ethical and comprehensive datasets are crucial to staying ahead of evolving fraud tactics in the financial sector.
The Future of AI Training Datasets
It is poised for significant advancements in the coming years. With the emergence of Pile v2, a vast, diverse, and continually updated dataset, we can expect several trends to shape the landscape. Firstly, AI Training Datasets will become even more extensive, encompassing broader domains and languages. This will enhance the capabilities of AI models across various applications, from healthcare to natural language processing.
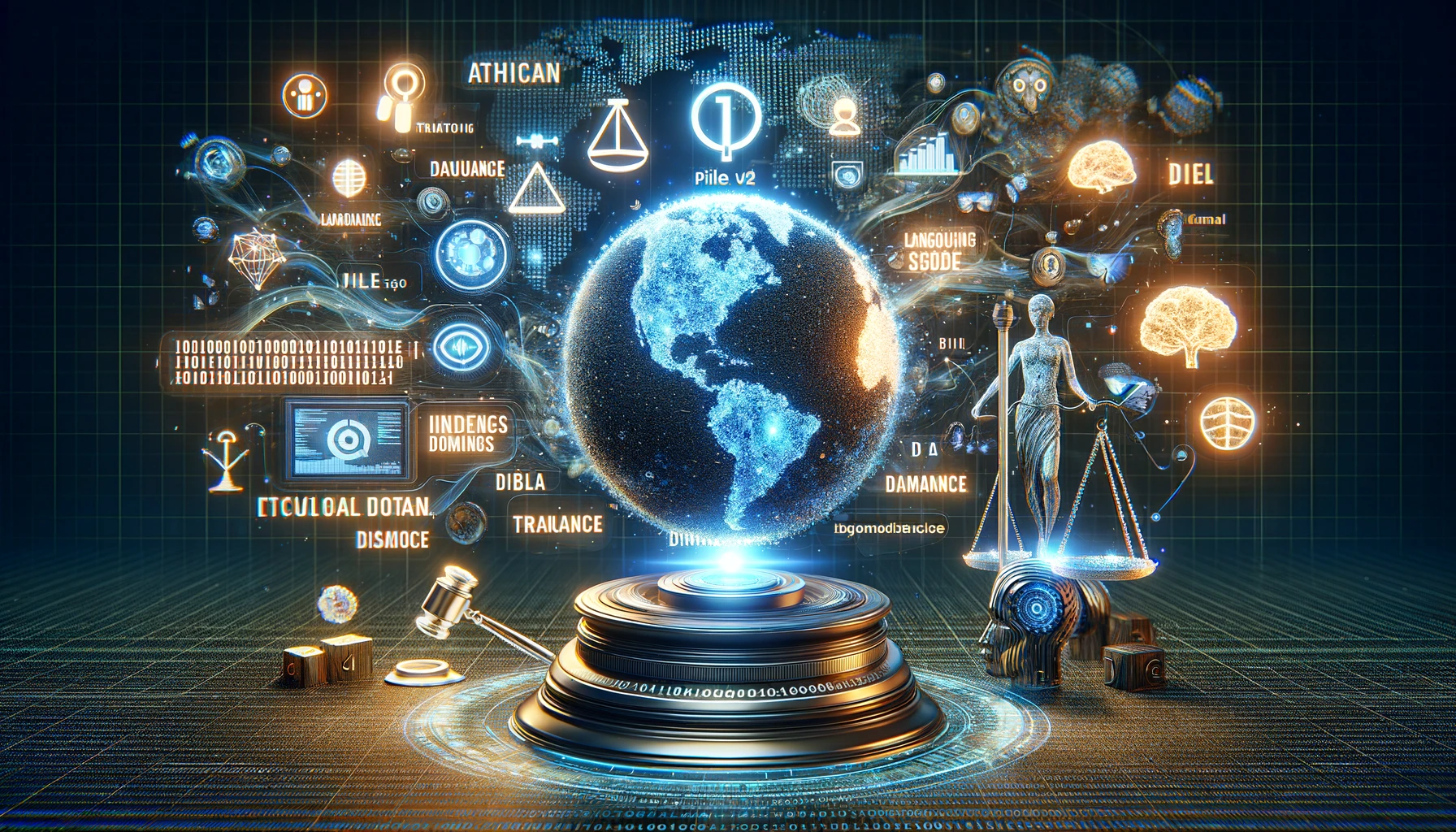
Moreover, ethical considerations and bias mitigation will gain prominence. Efforts to ensure fairness and transparency in AI Training Datasets will reduce algorithmic biases and improve AI-driven technologies’ ethical implications.
Furthermore, it will increasingly incorporate real-time data, enabling AI models to adapt swiftly to evolving environments. This will prove crucial in fields like autonomous vehicles and cybersecurity.
Advancements in AI Training Datasets and the Ethical Imperative
In the realm of AI Training Datasets, significant strides have been made, with Pile v2 marking a noteworthy milestone in enhancing both data quality and ethical considerations. The AI industry’s trajectory underscores the critical importance of prioritizing these facets to ensure the responsible and effective development of AI technologies. Delving into the innovations within AI Training Datasets and the emergence of Pile v2, we gain valuable insights into the future direction of AI and its potential to revolutionize diverse industries, all while maintaining rigorous ethical standards. This imperative ensures that AI continues to evolve as a force for good, benefiting society at large.