Table of Contents
Artificial Intelligence (AI) is dramatically transforming radiology, enhancing diagnostic precision and patient management. This technology enables healthcare providers to improve diagnostic accuracy, streamline operations, and innovate within healthcare practices. This article delves into AI’s profound impact on AI in radiology, exploring its advantages, practical applications, real-world success stories, challenges, and future outlook.
Introduction to AI in Radiology
AI’s integration into radiology is revolutionizing healthcare technology, facilitating innovative treatment plans and personalized patient care. The global market for AI in medical imaging, estimated at $0.98 billion in 2023, is projected to grow to approximately $11.76 billion by 2033, reflecting a robust CAGR of 28.19%. This significant growth underscores the potential for both startups and established companies to develop AI-driven solutions, fundamentally transforming patient care and diagnostic processes in radiology.
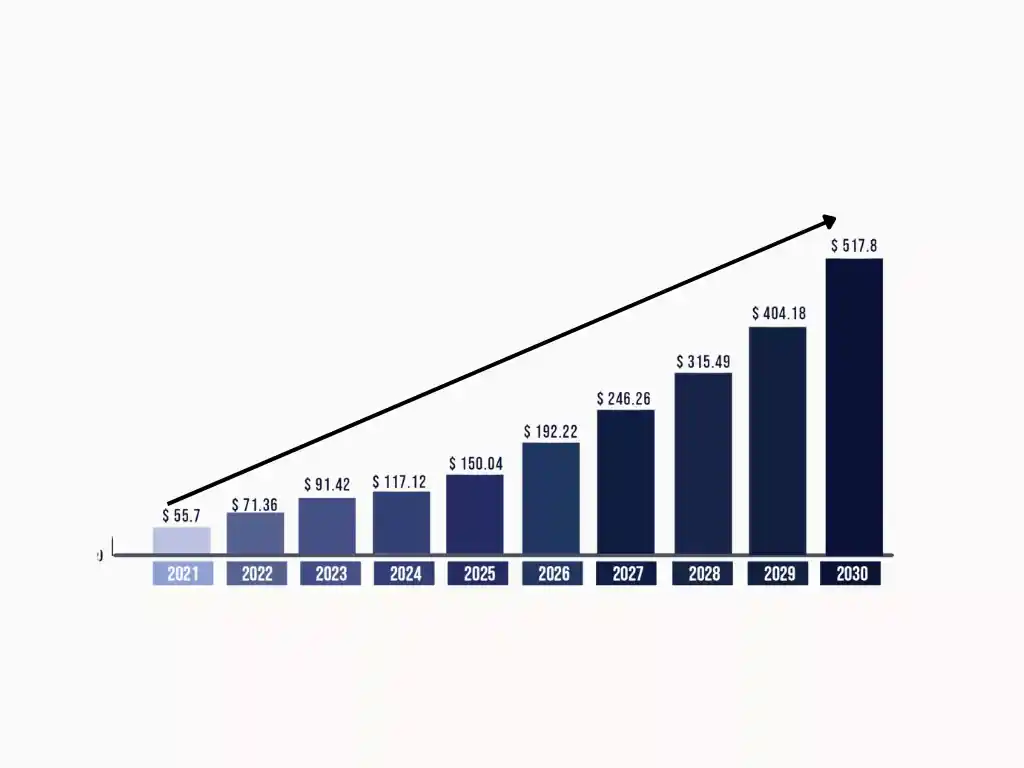
The Rise of AI in Radiology: Pioneering Precision and Efficiency
Enhancing Diagnostic Accuracy
AI in radiology is advancing medical diagnostics by improving accuracy, reducing diagnostic times, and enhancing patient outcomes. Radiologists now have tools that can rapidly analyze large volumes of imaging data, identifying patterns that humans might miss. Even so, developing these AI-powered tools particularly benefits rural areas where travel CT techs tend to be deployed to cover radiologist shortages and do not often have access to advanced equipment. This enhances diagnostic accuracy, reduces the likelihood of errors, and expands access to healthcare, contributing to a more reliable and efficient healthcare system.
Improving Operational Efficiency
AI significantly aids radiologists by automating tasks, improving diagnostic precision, and enhancing patient care, as reported by Johns Hopkins Medicine. This aligns with global trends where AI is becoming an integral part of medical imaging, optimizing workflows, and augmenting healthcare providers’ capabilities.
Case Study: Diagnostikum’s AI Utilization
Diagnostikum, an Austrian AI in radiology provider, showcases AI’s transformative impact. Using the AI-Rad Companion Chest CT, Diagnostikum addresses complex cases, heavy workloads, and staff shortages. This AI solution automates numerous radiological tasks, enhancing the diagnostic process and integrating seamlessly with existing digital systems.
Understanding the Benefits of AI in Radiology
AI is revolutionizing diagnostic processes and significantly boosting efficiency in radiology. Here’s a detailed look at its key advantages:
Increased Accuracy and Precision
AI enhances the accuracy of medical assessments by reducing human error, leading to more effective treatments. It streamlines the interpretation of complex images, ensuring reliable results, which is crucial for successful patient outcomes.
Enhanced Efficiency and Speed
AI integration in radiology reduces the time required for diagnostic processes, boosting overall efficiency. Faster image processing and analysis lighten radiologists’ workloads, ensuring quick, precise results and improving patient care.
Cost Reduction
AI streamlines radiological operations, leading to significant cost savings. By automating repetitive tasks, AI reduces the need for repeat scans, lowers operational costs, and enhances resource allocation, benefiting hospitals and clinics by reducing financial burdens and improving healthcare access.
Improved Patient Outcomes
AI improves diagnostic precision, directly enhancing patient health outcomes. It facilitates earlier and more accurate diagnoses, leading to more effective treatment planning, faster recovery times, fewer complications, and better patient care and satisfaction.
Advanced Research Capabilities
AI opens new avenues in radiological research, enhancing the development of novel diagnostic techniques. Researchers gain faster access to precise data, accelerating the innovation of new diagnostic methods and tools, advancing the understanding of complex medical conditions.
Use Cases of AI in Radiology
AI is transforming radiologists’ approach to imaging and diagnostics, enabling more precise and rapid identification of medical conditions. Here are some prominent use cases of AI in radiology:
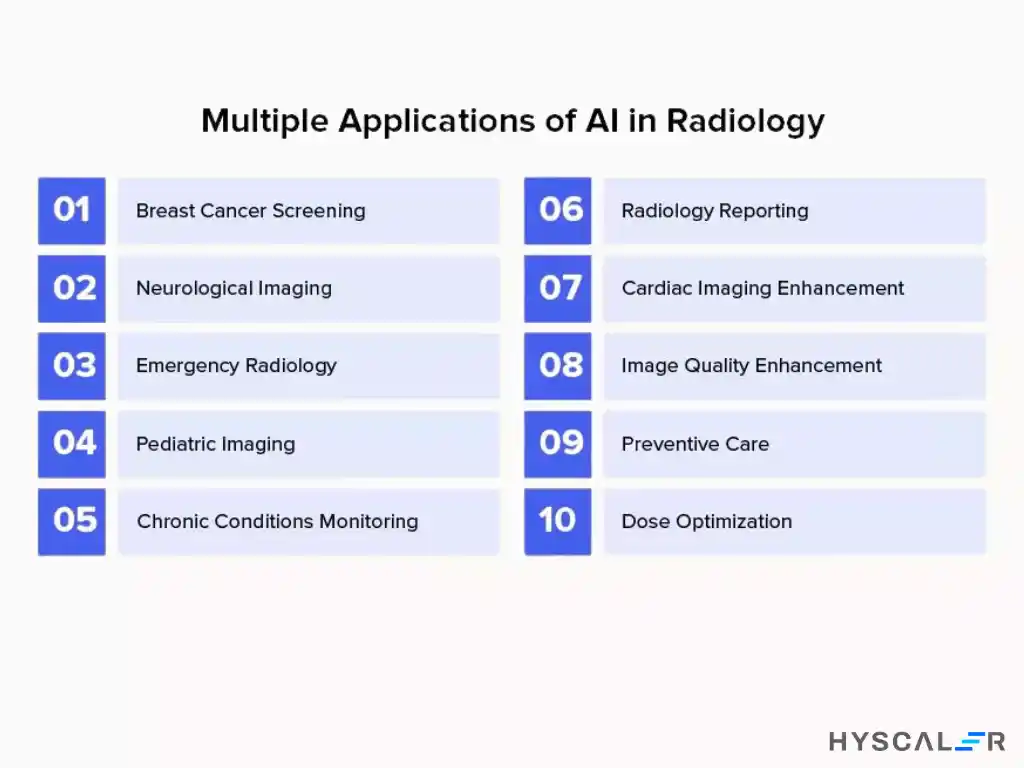
Breast Cancer Screening
AI improves breast cancer screening by analyzing mammograms more accurately than traditional methods, detecting early signs of cancer. This ensures timely interventions, potentially saving lives by identifying tumors before they spread and reducing the rate of false positives and negatives, lowering patient anxiety and unnecessary procedures.
Neurological Imaging
AI in neurological imaging enhances the detection of abnormalities such as tumors or strokes by analyzing brain scans more efficiently. This technology not only improves diagnostic accuracy but also speeds up interpretation, allowing quicker patient management and identifying subtle changes in brain structure indicative of early-stage diseases.
Emergency Radiology
In emergency radiology, AI rapidly diagnoses life-threatening conditions such as strokes, trauma, and internal bleeding. By analyzing images automatically and flagging critical cases, AI ensures that urgent cases receive immediate attention, significantly reducing response times, crucial for patient survival.
Pediatric Imaging
AI enhances the accuracy and safety of imaging procedures for children by reducing the radiation dose required during scans, which is vital for developing bodies. AI quickly identifies congenital and developmental abnormalities, supporting early and effective treatment plans.
Chronic Conditions Monitoring
AI improves the tracking and management of chronic conditions such as osteoporosis, arthritis, and cardiovascular diseases by analyzing imaging data over time. This continuous analysis detects subtle changes in the patient’s condition, enabling timely interventions and optimizing treatment plans.
Radiology Reporting
AI tools automatically generate preliminary reports by analyzing imaging data, enhancing diagnostic speed and accuracy. These technologies assist radiologists by highlighting areas of concern, ensuring no detail is overlooked, improving the consistency and quality of radiology reports, and reducing human error and workload.
Cardiac Imaging Enhancement
AI in cardiac imaging enhances the detection and analysis of heart-related anomalies. Advanced AI technologies identify subtle changes in cardiac structures, allowing early intervention and management of cardiac conditions. AI assists in detailed visualization of blood flow and heart function, offering invaluable insights for treatment planning.
Image Quality Enhancement
AI enhances the clarity and resolution of radiological images, aiding radiologists in making more accurate diagnoses. Improved image quality reduces the need for repeat scans, increasing efficiency and patient comfort. AI optimizes contrast and lighting, making subtle abnormalities more detectable.
Preventive Care
AI improves preventive care by analyzing imaging data to identify early signs of diseases such as cancer or vascular issues. Early detection allows treatments to prevent disease progression and improve patient outcomes. AI algorithms can predict potential health risks, enabling healthcare providers to recommend tailored preventive measures.
Dose Optimization
AI tailors the radiation dose to the minimum required to achieve clear images, reducing patient exposure while maintaining image quality. This precise calibration helps mitigate long-term risks associated with radiation, optimizing dosing protocols for various types of scans and patient demographics.
Real-Life Examples of AI in Radiology
IBM Watson Health
IBM Watson Health utilizes extensive datasets from radiology studies to enhance diagnostic accuracy by analyzing medical images to detect diseases such as lung cancer. Watson’s AI technology provides comprehensive patient profiles, including previous imaging studies and relevant medical history, improving diagnostic precision and patient treatment plans.
Google DeepMind
Google DeepMind’s partnership with UK hospitals focuses on improving the speed and accuracy of breast cancer diagnostics using AI. DeepMind’s AI analyzes mammograms, reducing false positives and negatives, enhancing diagnostic reliability, and potentially saving lives by identifying abnormalities more effectively.
Zebra Medical Vision
Zebra Medical Vision uses AI to enhance the early detection of various medical conditions, including pneumonia. Their advanced AI algorithms analyze chest X-rays, identifying subtle signs of this potentially life-threatening condition with remarkable precision, aiding radiologists in making faster and more accurate assessments.
Aidoc
Aidoc provides real-time decision support to radiologists through advanced AI algorithms that quickly analyze medical imaging, identify urgent cases, and alert medical staff. This ensures quicker attention to critical cases and streamlines workflows within emergency departments.
Arterys
Arterys excels in precision medicine with its AI-powered medical imaging platform, handling tasks from identifying breast cancer to diagnosing brain tumors and detecting strokes. Arterys’s Cardio AI processes cardiac images and generates detailed reports, improving diagnostic precision and alleviating radiologists’ workload.
Challenges Associated with AI Integration in Radiology
Accuracy and Reliability
Ensuring the accuracy and reliability of AI algorithms is crucial. These systems must be trained on diverse datasets to effectively recognize and interpret complex medical images across varied patient demographics, raising concerns about data privacy and ethical use of medical records.
Adaptability
AI systems must manage diverse human pathologies without sacrificing accuracy. Ensuring adaptability to a wide variety of medical cases with unique characteristics is essential.
Technological and Budgetary Constraints
Many radiology departments face technological and budgetary constraints that hinder the seamless adoption of AI solutions. Integration into existing healthcare systems can be challenging.
Regulatory Compliance
Navigating complex regulatory landscapes, maintaining ethical standards in data usage, and obtaining necessary certifications for clinical use are significant hurdles businesses need to overcome.
Training and Adaptation
Ongoing training and adaptation among medical staff are crucial to effectively utilize AI tools without undermining the vital role of radiologists in the diagnostic process.
Future Prospects of AI in Radiology
Enhanced Diagnostics
Predictions indicate that AI will play a vital role in transforming radiological diagnostics by improving accuracy and reducing diagnostic times. The growing demand for precise and efficient medical imaging is driving this shift, with AI technologies uniquely positioned to meet these needs.
Generative AI
Generative AI in radiology will revolutionize how medical imaging data is processed and interpreted, enabling the creation of enhanced imaging models that can predict patient outcomes more accurately and tailor treatments accordingly.
Global Collaboration
AI technologies will facilitate collaborative diagnostics, connecting radiologists and medical professionals globally. This networked approach will enhance knowledge sharing and improve diagnostic consistency across different regions.
Conclusion
The integration of AI in radiology is reshaping diagnostic imaging and patient care. From enhancing accuracy and efficiency to offering advanced research capabilities and transforming business models, AI is poised to significantly impact the future of radiology. As the technology continues to evolve, businesses in the healthcare sector must embrace these advancements to stay competitive and improve patient care outcomes.