Table of Contents
As the Generative AI domain surges forward, enterprises grapple with a pivotal challenge: deciphering and steering the expenses tied to large language models (LLMs). In an audacious move, Dataiku unveils its LLM Cost Guard, a novel facet of the Dataiku LLM Mesh, crafted to navigate this fiscal labyrinth.
The Advent of the LLM Cost Guard
LLM Cost Guard emerges as a beacon for enterprises, illuminating the path to prudent financial stewardship of Generative AI ventures. It meticulously tracks and scrutinizes LLM utilization, shedding light on the expenditures linked to distinct applications, purveyors, and users. This granular insight empowers businesses to discern the economic footprint of their LLM endeavors.
A Portal to Cost Clarity
Nestled within the Dataiku LLM Mesh, the LLM Cost Guard stands as a sentinel, offering a secure portal to a realm where LLM provider allegiance is rendered obsolete. It seamlessly amalgamates LLMs from industry titans such as OpenAI, Microsoft Azure, and others, fostering a provider-agnostic environment.
“AI is the linchpin of innovation and expansion for modern enterprises,” proclaims Florian Douetteau, Dataiku’s visionary co-founder and CEO. “LLM Cost Guard is our answer to the enigma of Generative AI costs, granting IT mavens the luxury of real-time fiscal oversight, liberating them to cultivate and pioneer.”
Demystifying Generative AI Expenditures
Generative AI’s potential to revolutionize industries has propelled its swift adoption. A staggering majority of organizations are poised to amplify their investments shortly. However, the art of cost containment remains elusive as IT vanguards zealously deploy LLMs. With projections placing the generative ai space at the helm of 55% of AI expenditures by 2030, the impetus for financially sound AI initiatives has never been greater
“Dataiku’s strides in cost monitoring are a watershed moment, addressing a dire market need,” asserts Ritu Jyoti, Group VP at IDC. “As Generative AI becomes intertwined with corporate operations, the demand for a tool that not only elucidates costs but also weaves governance into its fabric is paramount.”
The Dataiku LLM Mesh: A Conduit for Cost Transparency
The Dataiku LLM Mesh is a centralized nexus, boasting an extensive array of connections to the most sought-after LLM providers. Enterprises, with their penchant for diverse LLM vendors, find solace in LLM Cost Guard’s ability to monitor and relay insights, ensuring a panoramic view of their projects.
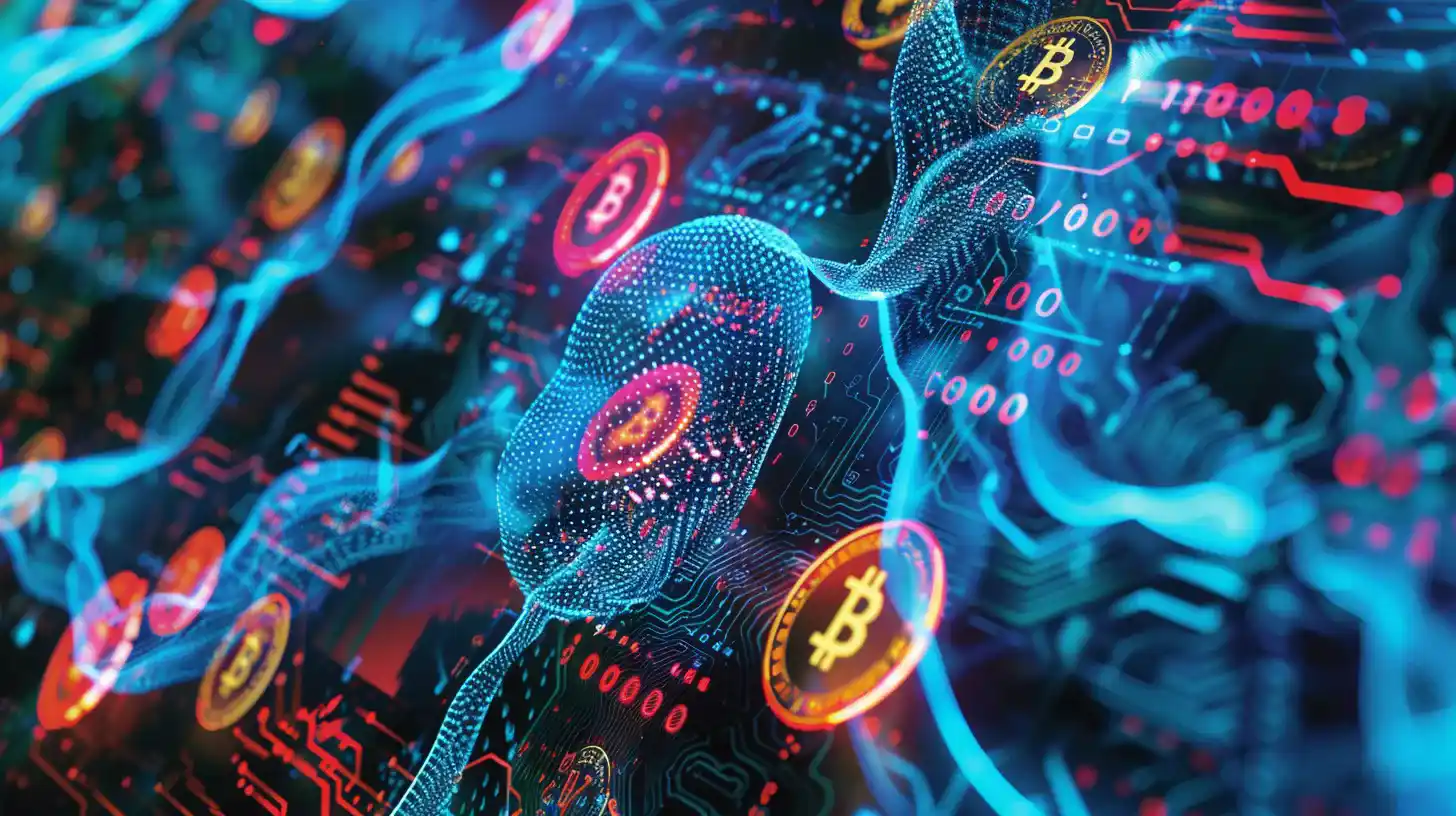
LLM Cost Guard empowers enterprises to:
- Catalog and Monitor LLM Outlays: Attribute costs to specific ventures, enhancing financial lucidity and responsibility.
- Dissect Costs: Differentiate between operational and developmental outlays, bolstering strategic fiscal planning.
- Forge Early Alerts: Detect fiscal excesses swiftly, averting monetary perils, including governance lapses.
- Harvest In-Depth Insights: Utilize a comprehensive dashboard to steer LLM investments judiciously.
How does LLM Cost Guard work?
LLM Cost Guard serves as a complete monitoring mechanism for Dataiku’s LLM Mesh. It’s intended to provide real-time visibility into Generative AI expenditures, with a thorough breakdown of charges by application, service, and user. Here’s how it works.
- Performance Dashboard: It contains a pre-built dashboard that monitors consumption and expenses across various LLM services and providers, assisting teams in diagnosing problems and selecting the best service for their requirements.
- Cost Tracking: The system enables effective tracking of LLM usage, with a fully auditable log that shows who is using which LLM service and for what reason. This simplifies expense tracking and internal rebilling.
- Provider Comparison: LLM Cost Guard provides strong monitoring across several models and providers, providing a holistic picture of costs and allowing proactive financial management for all corporate use cases.
- LLM Operations Strategy: It is part of the bigger Dataiku LLM Guard Services, which offer more cost-effective monitoring solutions. These services contribute to continuous development and a feedback loop by monitoring resource utilization, recognizing and managing PII, detecting toxicity, and blocking banned phrases.
- Cost savings: The system also allows you to cache results for typical requests, which saves money and improves speed. Teams that self-host can save money on infrastructure by caching local Hugging Face models.