Table of Contents
In the past, mathematicians used to record their formulas on paper of mathematics and use a pencil to find solutions that they considered pure and elegant. However, in the 1970s, they cautiously started utilizing computers to help them tackle certain problems. As time passed, computers have become frequently employed to solve the most challenging mathematical puzzles. Now, following a similar approach, a group of mathematicians are embracing machine learning tools to assist them in their numerical endeavors.
Mathematics and Machine Learning: Bridging Expertise for Future Innovation
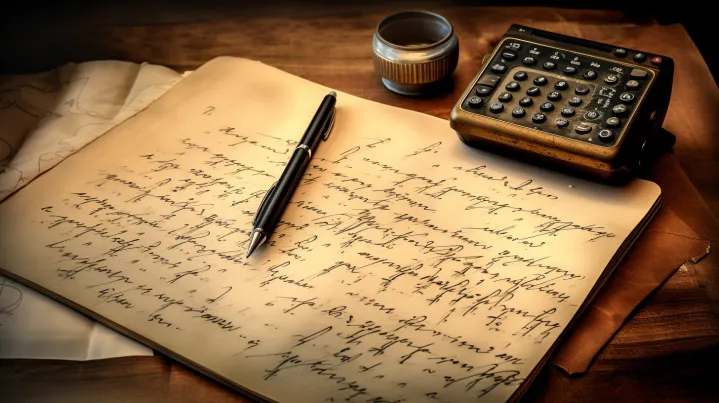
- Adopting Machine Learning in the Field of Mathematics
Sergei Gukov, the John D. MacArthur Professor of Theoretical Physics and Mathematics at Caltech, has organized the Mathematics and Machine Learning 2023 conference, scheduled to be held at Caltech from December 10th to 13th. According to Gukov, mathematicians are now starting to adopt machine learning techniques.
Gukov asserts that there exist certain mathematicians who might retain doubts regarding the utilization of these tools. He further states that although the tools may be mischievous and lack the purity of paper and pencil, they are nonetheless effective.
- Machine Learning: Transforming Math Problem Solving
Machine learning, a branch of artificial intelligence (AI), involves training computer programs on extensive datasets to identify novel patterns and make accurate predictions. The inaugural conference organized by the recently established Richard N. Merkin Center for Pure and Applied Mathematics aims to foster collaboration between data scientists, who develop machine learning tools, and mathematicians. The primary objective is to explore synergies between these two domains and facilitate mutual enrichment.
- Mathematics and Machine Learning: A Mutual Relationship
Gukov, the director of the recently established Merkin Center by Caltech Trustee Richard Merkin, emphasizes the reciprocal nature of the relationship. According to him, mathematicians can contribute by devising innovative algorithms for machine learning tools such as those employed in generative AI programs like ChatGPT. Conversely, machine learning can assist in solving intricate mathematical problems.
Yi Ni, a mathematics professor at Caltech, intends to participate in the conference, despite not incorporating machine learning into his own research. His area of expertise lies in topology, particularly the examination of mathematical knots in lower dimensions. According to Ni, there exists a disparity among mathematicians in their familiarity with advanced tools, such as machine learning. He emphasizes the importance of having access to an expert in machine learning who is willing to assist. Ultimately, Ni believes that artificial intelligence for mathematics will evolve into a distinct subfield within the realm of mathematics.
- The Riemann Hypothesis and the Application of Machine Learning
The Riemann hypothesis, a challenging problem that could potentially be solved with the assistance of machine learning, has been identified by Gukov. This problem, named after the renowned mathematician Bernhard Riemann from the 19th century, is among the seven Millennium Problems chosen by the Clay Mathematics Institute. Each of these problems carries a prize of $1 million for its successful resolution.
The Riemann hypothesis revolves around the Riemann zeta function, a formula that encapsulates information about prime numbers. If confirmed, this hypothesis would offer a fresh perspective on the distribution of prime numbers. The utilization of machine learning tools could aid in unraveling this problem by offering an alternative approach to exploring numerous iterations of the hypothesis.
Gukov emphasizes the effectiveness of machine learning tools in pattern recognition and analysis of intricate problems. On the other hand, Ni acknowledges that while machine learning solutions may lack aesthetic appeal, they excel in discovering novel connections. However, Ni also highlights the importance of mathematicians in transforming questions into computationally solvable forms.
Machine Learning and Knot Theory
Gukov has personally employed machine learning to unravel complexities in knot theory. Knot theory involves the examination of theoretical knots, resembling the knots commonly found in shoestrings, but with closed loops at their ends. These mathematical knots can be intertwined in different manners, and mathematicians like Gukov strive to comprehend their structures and interconnections. This research also intersects with other branches of mathematics, including representation theory and quantum algebra, and even extends to the realm of quantum physics.
Gukov and his colleagues are currently focused on resolving the smooth Poincaré conjecture in four dimensions. This conjecture, which is also classified as a Millennium Problem, was initially proposed by mathematician Henri Poincaré in the early 20th century. Notably, it was eventually solved between 2002 and 2003 by Grigori Perelman, who famously declined the accompanying $1 million prize.
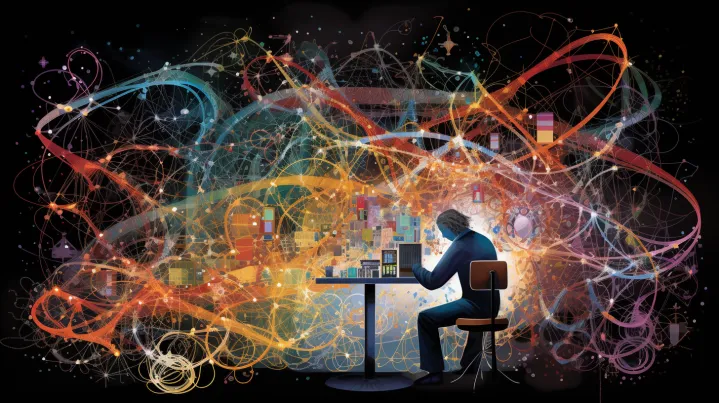
The crux of the problem involves comparing spheres to specific types of manifolds that exhibit a resemblance to spheres. Manifolds, in this context, refer to shapes that result from projecting higher-dimensional objects onto lower dimensions. Gukov likens the essence of the problem to questioning the true nature of objects that bear a resemblance to spheres.
Gukov and his team have devised a machine-learning approach to assess ribbon knots in their endeavor to solve the four-dimensional smooth Poincaré conjecture. This conjecture asserts that in four dimensions, any manifold resembling a sphere is, in fact, a sphere.
According to Gukov, our brain is incapable of comprehending four dimensions, thus we tend to simplify shapes by forming knots. A knot can be visualized as a ribbon where the string passes through a different section of the string in three dimensions but does not intersect with anything in four dimensions. By utilizing machine learning, we are able to examine the quality of “ribboness” in knots, which is a binary characteristic of knots and holds significance in the study of the smooth Poincaré conjecture.
In their preprint paper titled “Searching for Ribbons with Machine Learning,” Gukov and his team emphasize the role of machine learning in solving problems. They highlight its capability to efficiently explore numerous potential solutions and enhance the search process based on successful outcomes. The term “games” is used to describe this approach, as similar algorithms and structures can be utilized in playing intricate board games like Go or chess, where the objectives and winning strategies resemble those in mathematical problems.
Mathematics and Machine Learning Interplay
Mathematics can play a crucial role in the advancement of machine learning algorithms, as stated by Gukov. According to him, having a mathematical mindset can introduce innovative concepts to the creation of AI tools’ algorithms. Gukov highlights Peter Shor as an exemplary mathematician who contributed valuable insights to computer science challenges. Shor, who completed his undergraduate studies in mathematics at Caltech in 1981, is renowned for devising Shor’s algorithm. This algorithm consists of a series of principles that could potentially enable quantum computers in the future to factor integers at a faster rate than conventional computers, consequently rendering digital encryption codes vulnerable.
Machine learning algorithms in today’s world are trained using extensive datasets. These algorithms analyze vast amounts of data, including language and images, in order to identify patterns and establish new connections. However, the process by which these algorithms arrive at their conclusions is often unknown to data scientists. This lack of transparency is due to the algorithms being concealed within a “black box.” By adopting a mathematical approach to algorithm development, as suggested by Gukov, we can uncover the inner workings of these algorithms and gain a deeper comprehension of their functionality. This understanding will enable us to make enhancements and improvements to the algorithms.