Table of Contents
Artificial intelligence (AI) is rapidly transforming our world, quietly weaving itself into the fabric of our daily lives. From recommending movies to filtering emails, AI algorithms are making decisions that impact us in countless ways. However, a major concern has emerged – the lack of transparency in these complex systems. This is where Explainable AI (XAI) steps in, aiming to shed light on the inner workings of AI and build trust in its decision-making processes.
The Need for Explainable AI
Imagine a loan application being rejected by an AI system, but you have no idea why. This lack of explanation can be frustrating, unfair, and even discriminatory. Here’s why XAI is crucial:
- Transparency and Trust: When people understand how AI arrives at a decision, they’re more likely to trust and accept its outcome. XAI fosters transparency, allowing users to understand the rationale behind an AI system’s recommendations. For instance, if an AI recruiting tool rejects a candidate, XAI could explain which aspects of the resume didn’t align with the job description. This transparency fosters trust and allows for potential corrections in the application process.
- Fairness and Bias Detection: AI algorithms can inherit biases from the data they’re trained on. Imagine a facial recognition system trained on a biased dataset – it might struggle to accurately recognize faces of certain ethnicities. XAI helps identify and mitigate bias, ensuring fair and equitable treatment for everyone. By analyzing the factors influencing an AI’s decision on loan applications, for example, XAI can expose potential biases based on race or gender in the lending process.
- Human Oversight and Accountability: Complex AI models can become intricate black boxes. Without understanding how they arrive at decisions, it’s difficult to hold them accountable. XAI provides human experts with the ability to understand the model’s reasoning, enabling better oversight and accountability. In healthcare, XAI can explain diagnoses made by AI systems, allowing doctors to understand the reasoning behind the diagnosis and intervene if necessary. This ensures that the ultimate decision-making authority remains with humans.
Benefits of Explainable AI
XAI offers a multitude of advantages that extend beyond user trust:
- Improved Decision-Making: Users can make more informed choices by understanding the factors influencing an AI’s decision. Imagine an AI risk assessment tool flagging a potential security threat. XAI can explain the rationale behind the assessment, allowing security personnel to prioritize threats and allocate resources effectively.
- Enhanced Debugging and Model Improvement: XAI techniques can pinpoint weaknesses and biases within an AI model. Let’s say an AI customer service chatbot consistently steers conversations toward a specific product. XAI can identify patterns in the training data that might be causing this bias, allowing developers to refine the training data and improve the chatbot’s responses.
- Responsible AI Development: XAI empowers developers to build AI systems aligned with ethical principles. Transparency fosters responsible development, ensuring AI is used for good. Consider an AI system designed to automate hiring decisions. XAI can ensure the system is evaluating candidates based on relevant skills and experience, promoting fair hiring practices.
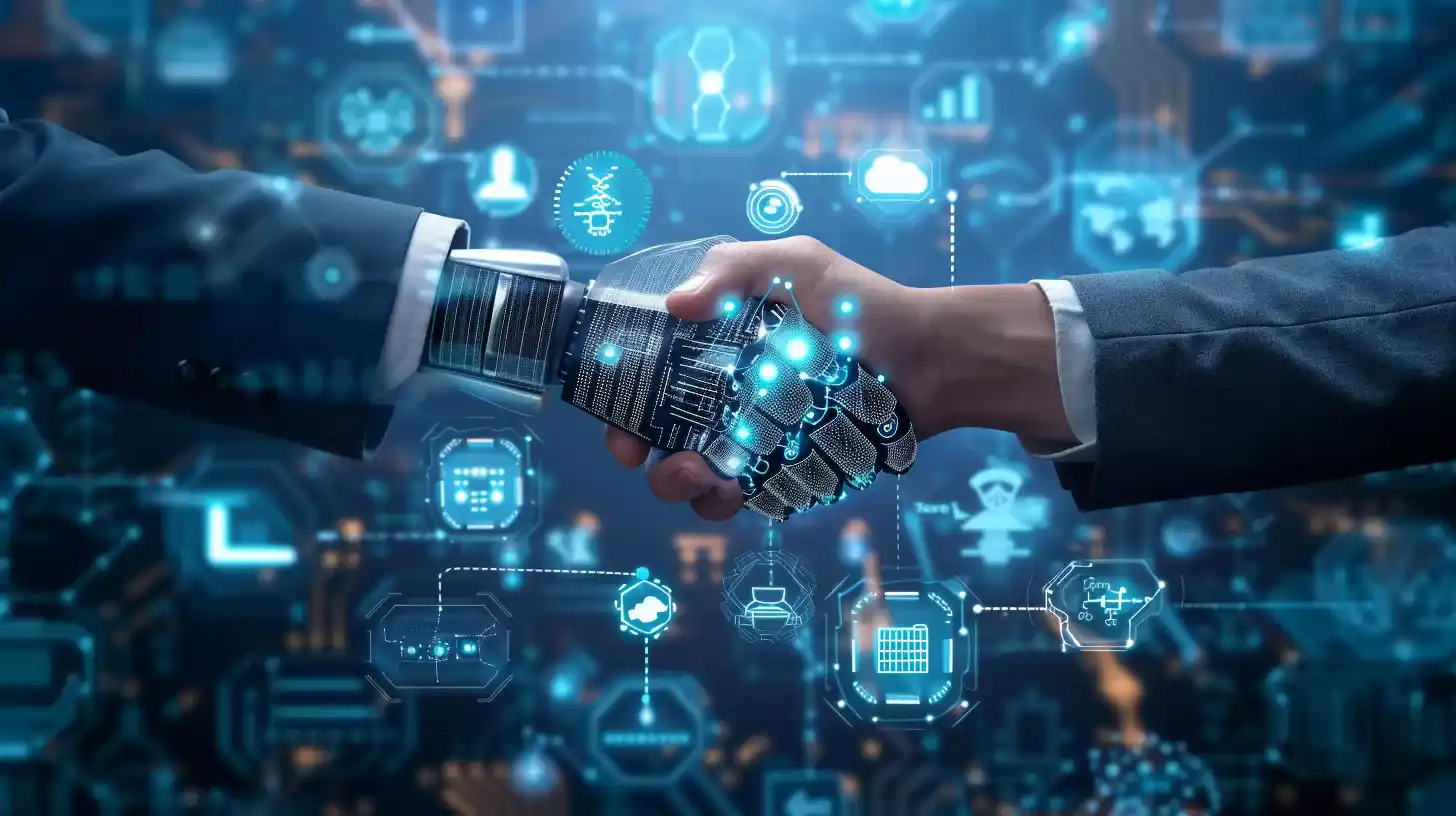
Approaches to Achieving Explainable AI
There’s no one-size-fits-all approach to Explainable AI (XAI). Different techniques work better for different types of AI models. Here are some common approaches:
- Model-Agnostic Methods: These techniques work across various models, explaining their predictions through feature attribution. They highlight the data points that most significantly influenced the model’s decision. For instance, an XAI method might reveal that a particular applicant’s credit score was the most significant factor in an AI loan rejection decision.
- Model-Specific Methods: These techniques are tailored to specific AI model architectures. For instance, with decision trees, XAI methods can visualize the decision-making process with tree structures, allowing users to see the sequence of questions the model asked to arrive at a decision.
- Counterfactual Explanations: These methods explore hypothetical scenarios – “What if this data point was different?” – to explain the impact of specific features on the model’s output. Imagine an AI system recommending a movie based on your viewing history. A counterfactual explanation might show how the recommendation would change if you hadn’t rated a particular genre highly.
Industry Applications of Explainable AI
Explainable AI (XAI) is finding applications in various sectors, making AI more reliable and trustworthy:
- Finance: XAI can explain loan approval decisions, helping lenders build trust with borrowers and ensure fair lending practices. By explaining the factors influencing loan approvals, XAI can also help lenders identify areas for improvement in their lending criteria.
- Healthcare: XAI can explain medical diagnoses made by AI systems, allowing doctors to understand the reasoning behind the diagnosis and make informed treatment decisions.
- Criminal Justice: XAI can explain risk assessments used in criminal justice, promoting transparency and preventing potential biases in sentencing decisions. For instance, by defining the factors influencing an AI’s risk assessment of a repeat offender, XAI can help judges make more informed decisions about parole or rehabilitation programs.
- Manufacturing: XAI can explain the reasoning behind predictive maintenance recommendations made by AI systems, allowing factory personnel to understand why a particular machine might need maintenance and prioritize accordingly. This can lead to improved production efficiency and reduced downtime.
The Future of Explainable AI
Explainable AI (XAI) is a rapidly evolving field with immense potential. Here’s a glimpse into the future:
- Evolving XAI Techniques: New and sophisticated XAI techniques are constantly being developed. We can expect advancements in model-agnostic methods, allowing for broader applicability across different AI models. Additionally, the development of domain-specific XAI solutions tailored to particular industries can be expected. For example, we might see the emergence of XAI techniques specifically designed for explaining complex medical diagnoses made by AI systems.
- Standardization and Regulations: As XAI becomes more prominent, standardization of XAI practices and regulations might emerge. This could involve establishing guidelines for developers to ensure a baseline level of explainability in their AI systems. Regulations might also be introduced to promote responsible AI development and mitigate potential risks associated with opaque AI systems.
- Human-AI Collaboration: The future lies in fostering human-AI collaboration, where humans leverage XAI to understand AI recommendations and make better joint decisions. Imagine an AI system recommending marketing strategies. With XAI explanations, marketing professionals can understand the rationale behind the recommendations and adapt them to their specific target audience, leading to more effective marketing campaigns.
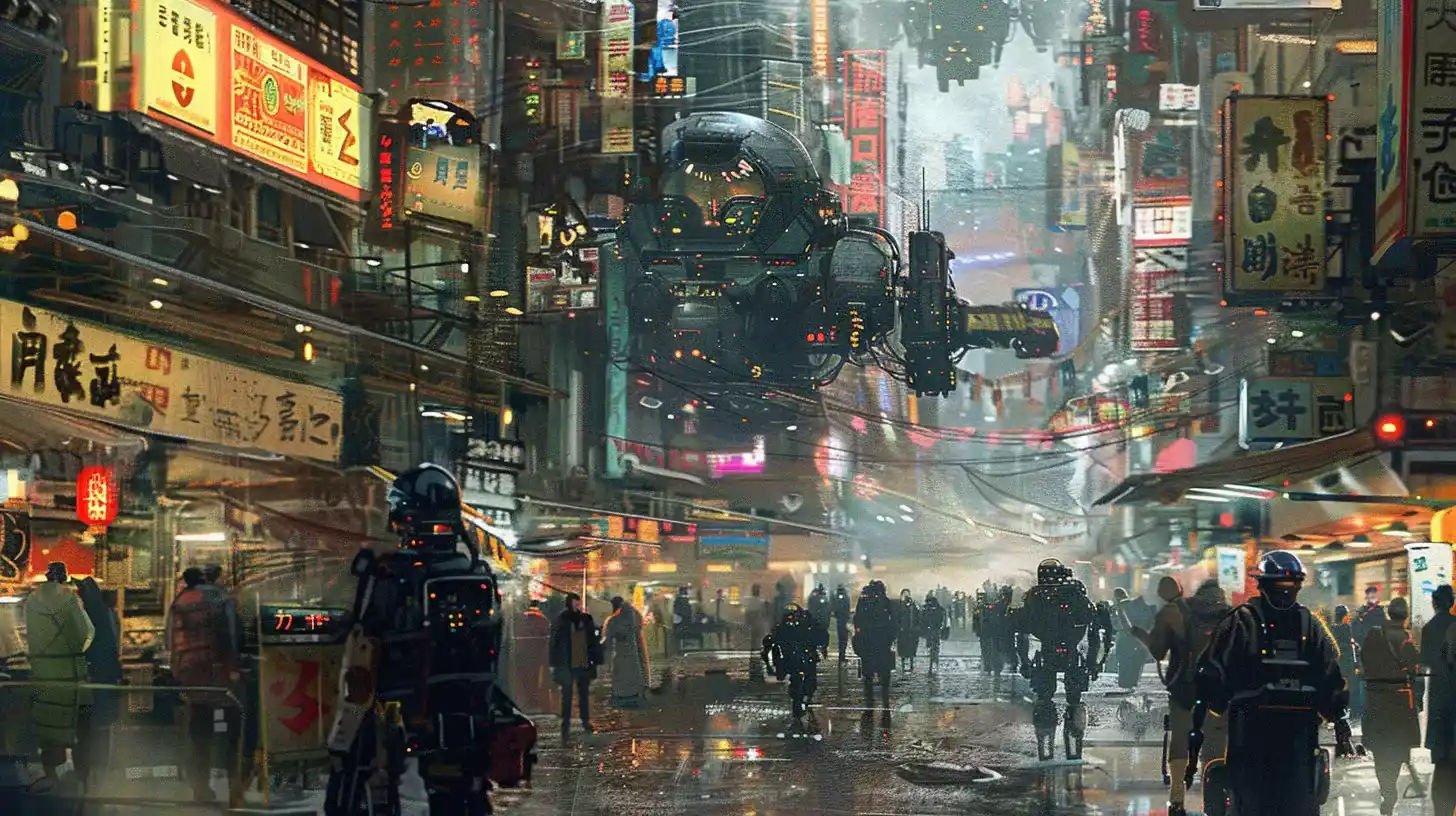
Conclusion
Explainable AI (XAI) goes beyond revealing the inner workings of AI systems; it focuses on establishing trust and promoting ethical AI advancement. With AI becoming more prevalent in various aspects of society, such as autonomous vehicles and personalized education tools, XAI is crucial for promoting responsible AI usage and shaping a future where AI benefits everyone. Through promoting transparency and comprehension in AI decision-making, XAI sets the stage for a future where humans and AI can work together harmoniously to address challenging issues and improve the world.
FAQs
Q. What is explainable AI (XAI)?
Explainable AI (XAI) refers to techniques and methods used to make AI models and their decision-making processes more transparent and understandable to humans.
Q. Why is explainable AI important?
Explainable AI (XAI) is important because it builds trust, ensures accountability, and promotes ethical AI use by making AI systems’ decision-making processes transparent and understandable.
Q. How does explainable AI improve model performance?
Explainable AI (XAI) improves model performance by helping developers identify weaknesses and biases in AI models, allowing for iterative refinement and improvement.
Q. What are some common techniques for achieving explainable AI?
Common techniques for achieving explainable AI include post-hoc explainability methods (e.g., feature importance, saliency maps, surrogate models) and intrinsically interpretable models (e.g., decision trees, linear regression).
Q. In which industries is explainable AI particularly useful?
Explainable AI (XAI) is particularly useful in industries such as healthcare, finance, law enforcement, and any other field where transparency and accountability in AI decision-making are crucial.
Q. What is the future of explainable AI?
The future of explainable AI includes advancements in techniques, increased regulatory and ethical considerations, and broader adoption across various industries to ensure trustworthy and ethical AI systems.