Table of Contents
The world of artificial intelligence is constantly evolving, with new hardware and software advancements pushing the boundaries of what’s possible. One recent development that has generated significant buzz is the Sohu chip, designed by the company Etched.
Sohu is a single-model AI chip, meaning it’s specifically designed to excel at running a particular type of AI model known as transformers. Transformers have become the dominant architecture for various AI tasks, including natural language processing, image recognition, and code generation.
This article delves into the Sohu chip, exploring its technical specifications, potential applications, and the challenges and limitations it faces. We’ll also discuss the future implications of this specialized chip and its potential impact on the AI hardware landscape.
Why a Single-Model AI Chip?
Traditionally, AI hardware like GPUs (Graphics Processing Units) has been designed for versatility. They can handle various types of AI models, offering flexibility for developers. However, this flexibility comes at a price.
Sohu takes a different approach. By specializing in transformers, Etched claims the chip can achieve significantly faster performance and lower power consumption compared to running transformers on a general-purpose GPU.
Here’s the reasoning behind Sohu’s design:
- Transformer-focused architecture: Sohu optimizes its internal workings specifically for the way transformers process data. This allows for a more efficient allocation of resources compared to a general-purpose GPU.
- Reduced overhead: By eliminating the need for supporting various types of AI models, Sohu minimizes unnecessary processing overhead. This translates to faster execution times.
- Lower power consumption: Tailoring the chip for transformers allows for a more energy-efficient design, leading to potential cost savings and reduced environmental impact.
Sohu Chip: Technical Specifications
While detailed technical specifications haven’t been publicly released yet, Etched has provided some insights:
- Focus on throughput: Sohu is built for speed, boasting a processing power measured in tokens per second, catering to the high-throughput nature of transformer models. Tokens are the basic units of text that transformers process and a higher throughput signifies the ability to handle massive amounts of text data efficiently.
- Custom architecture: The chip utilizes a custom architecture optimized for transformer operations, likely including specialized cores and memory configurations. These custom elements would be designed to handle the specific computations involved in transformer models, further accelerating performance.
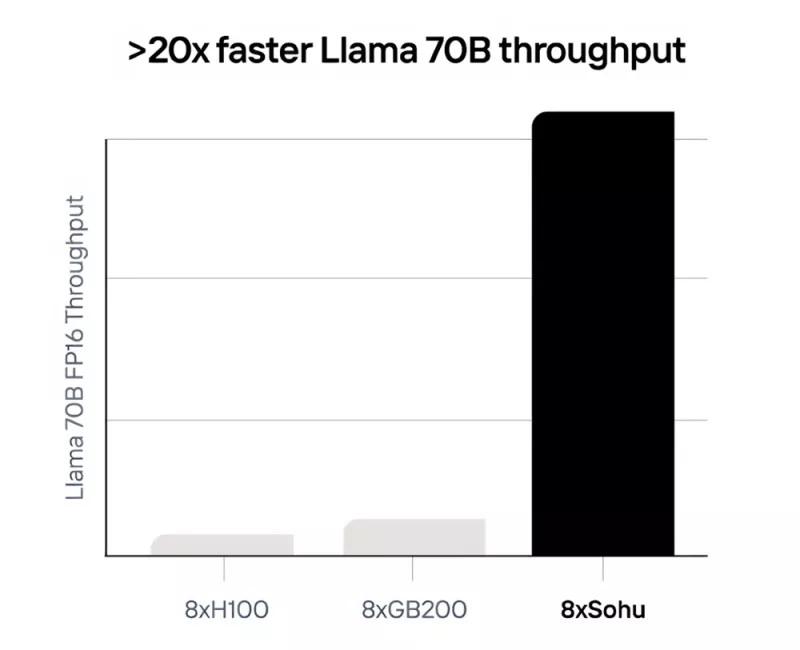
Potential Applications
Sohu’s capabilities align well with tasks that heavily rely on transformer models. Here are some potential applications:
- Natural Language Processing (NLP): Sohu can accelerate tasks like machine translation, text summarization, and sentiment analysis. In machine translation, Sohu could enable real-time translation of spoken language or large documents, significantly improving communication efficiency. For text summarization, Sohu could power the creation of concise and informative summaries of lengthy articles or research papers. Sentiment analysis, which involves gauging the emotional tone of text, could benefit from Sohu’s speed, allowing for faster analysis of social media trends or customer reviews.
- Large Language Models (LLMs): Training and running powerful LLMs, like ChatGPT, Gemini, etc would benefit significantly from Sohu’s speed and efficiency. LLMs are AI models that can create text, translate languages, produce various creative content, and provide informative answers to questions. Sohu’s ability to handle massive amounts of data and complex computations would be instrumental in training even more powerful LLMs in the future.
- Computer Vision: Transformer-based vision models used for object detection, image segmentation, and image generation could see a performance boost with Sohu. Object detection involves pinpointing and classifying objects within an image, while image segmentation separates different objects within an image into distinct categories. Image generation, on the other hand, refers to the creation of entirely new images based on specific prompts or instructions. Its processing power could accelerate these tasks, leading to faster image analysis and more realistic image generation.
- Generative AI: Applications like code generation, music composition, and creative text formats could leverage Sohu’s capabilities. Generative AI models can create new and original content, and their ability to handle complex data patterns could prove valuable in these areas. For instance, it could be used to generate different variations of creative text formats, like poems or code snippets, based on specific user inputs or parameters.
Challenges and Limitations
Despite its potential, Sohu faces some challenges:
- Limited applicability: As a single-model chip, it is restricted to running transformer models. This could be a drawback for developers who work with diverse AI models encompassing various architectures beyond transformers.
- Software ecosystem: Compatibility with existing software frameworks and libraries optimized for GPUs might require additional development. To fully harness its potential, developers would need tools and libraries specifically designed to work with Sohu.
- Learning curve: Developers accustomed to working with GPUs might need to adapt their workflow and learn new programming paradigms to leverage Sohu effectively. This learning curve could hinder the chip’s adoption rate in the short term.
- Maturity: As a new technology, it is still in its early stages. There might be unforeseen bugs or limitations that emerge as developers begin using the chip in real-world applications. Etched will need to address these issues through ongoing development and refinement.
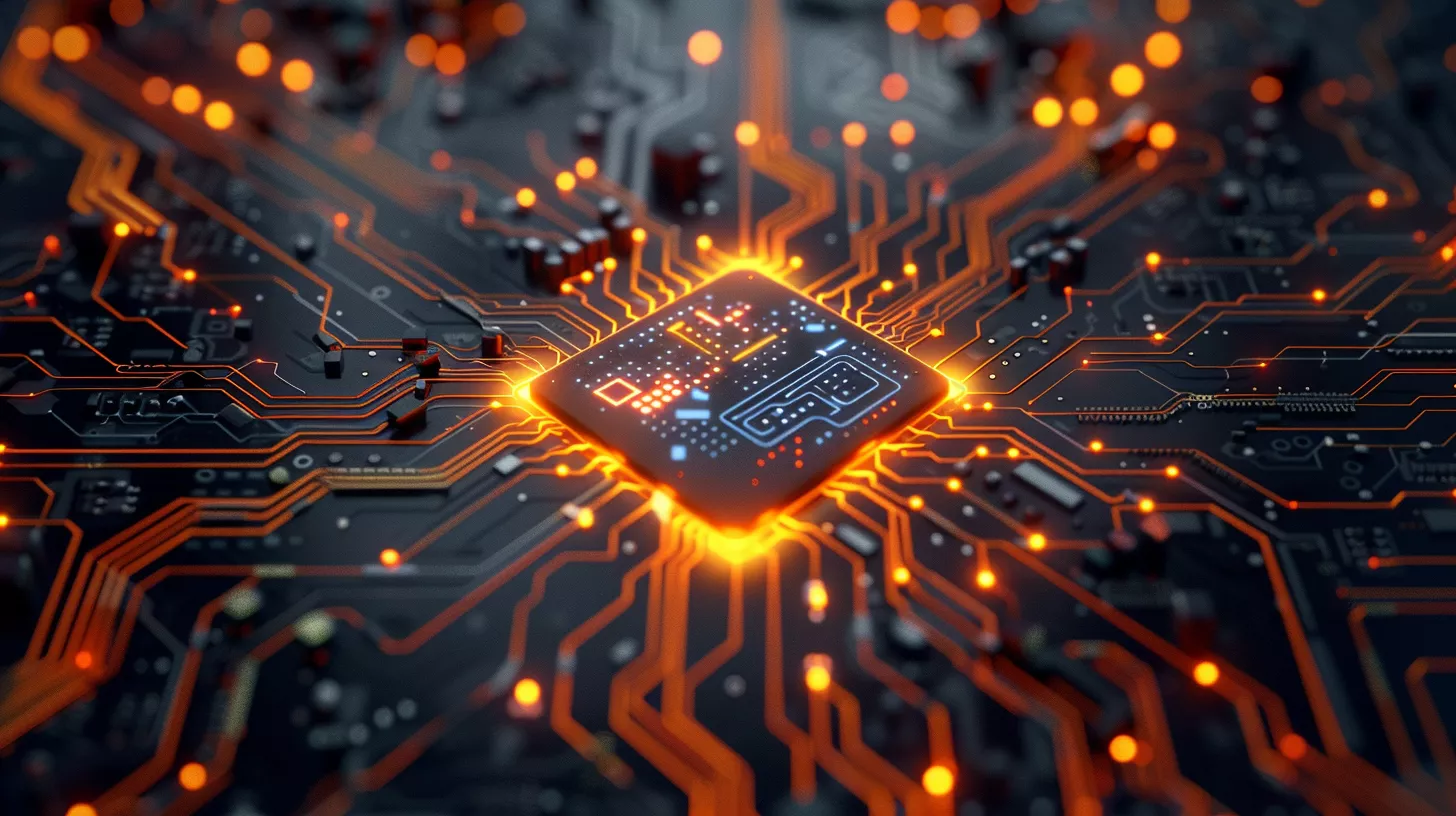
Future Implications
The Etched’s AI chip represents a significant step forward in specialized AI hardware. Its potential benefits include:
- Faster AI development: Sohu’s speed and efficiency could accelerate the training and deployment of transformer-based AI models, leading to faster innovation in various fields.
- Reduced operational costs: Lower power consumption could translate to significant cost savings for companies and organizations deploying large-scale AI models.
- More efficient AI applications: Sohu’s capabilities could pave the way for the development of more efficient and powerful AI applications across various industries.
However, the impact of Sohu will also depend on how effectively it addresses its limitations:
- Need for broader applicability: If Etched can expand its capabilities to support a wider range of AI models beyond transformers, it would gain wider appeal among developers.
- Software development: The development of a robust software ecosystem specifically designed for Sohu is crucial for its success. This would encourage developers to adopt the chip and unlock its full potential.
Overall, Etched’s AI chip has the potential to be a game-changer for transformer-based AI. Its focus on performance and efficiency aligns well with the growing demands of AI applications. How Etched addresses the challenges and limitations will determine the chip’s ultimate impact on the future of AI hardware.