Table of Contents
In the dynamic realm of the financial sector, banks are confronted with the imperative task of securing their future against an array of evolving risks. This article ventures into the heart of this challenge, introducing the concept of “Secure Fin Futures.” Within this framework, we embark on an exploration of ten groundbreaking machine learning (ML) solutions meticulously crafted to address and mitigate risks inherent to the banking industry proactively.
These cutting-edge ML solutions form a strategic arsenal for financial institutions, equipping them with the tools to navigate the complexities of modern finance. From predictive analytics that anticipate market trends to robust fraud detection systems and algorithmic trading strategies optimizing investment portfolios, each solution plays a pivotal role in fortifying the resilience of banks.
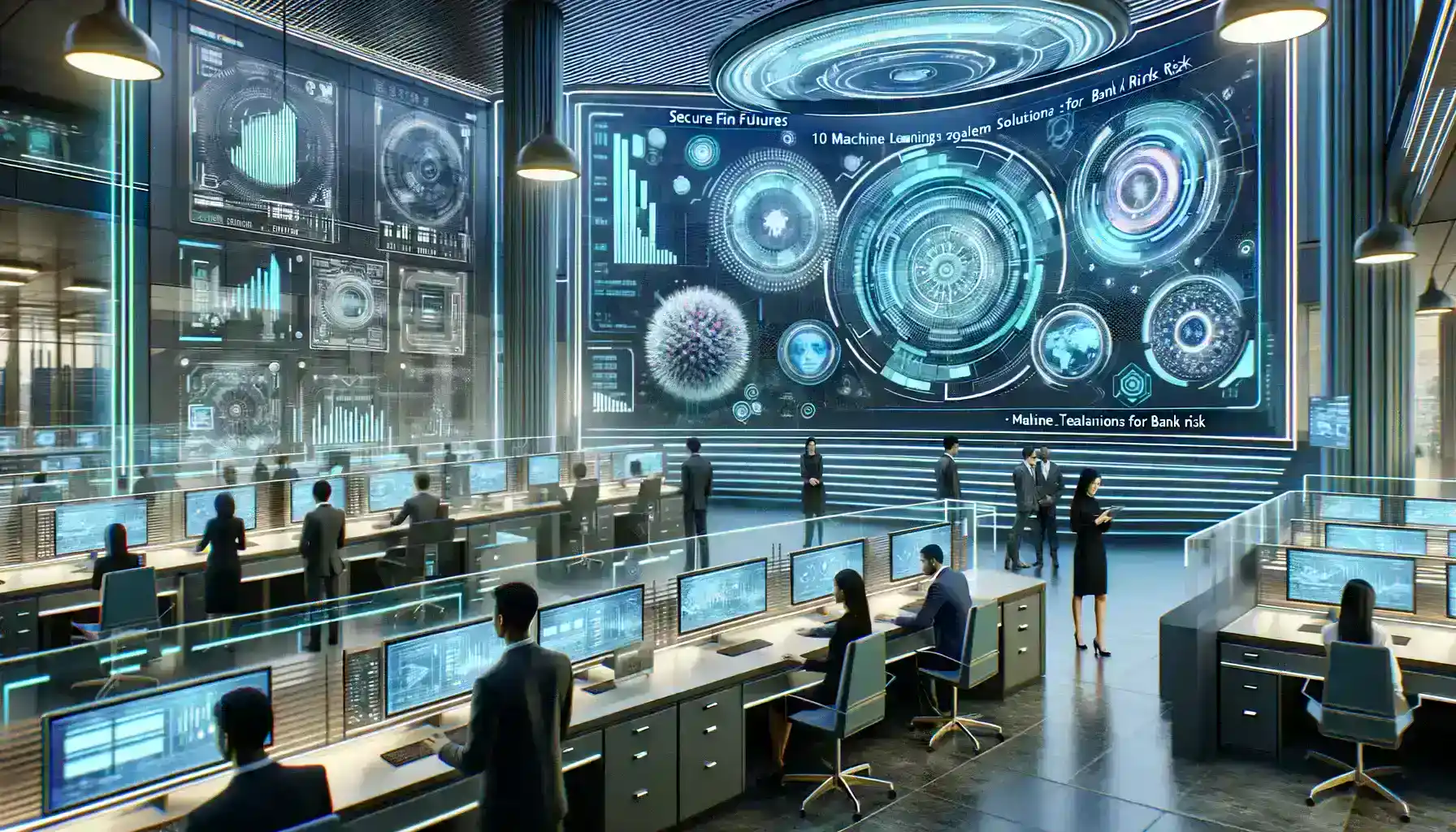
Additionally, regulatory compliance enhancements, customer behavior analysis, and stress-testing simulations contribute to a comprehensive approach to risk management.
As we delve into real-world success stories and glimpse into the future, it becomes evident that “Secure Fin Futures” is not just a concept but a transformative paradigm, ensuring banks remain robust, adaptive, and secure Fin Futures in an ever-changing financial landscape.
The Landscape of Financial Risks
In the dynamic realm of finance, comprehending the intricate web of risks is paramount. Banks contend with a spectrum of uncertainties, ranging from the unpredictable undulations of market forces to the stringent demands of ever-evolving regulatory landscapes.
This intricate interplay of variables underscores the need for a strategic and innovative approach to risk management. “Secure Fin Futures” emerges as a proactive solution, poised to confront these challenges head-on.
The initiative revolves around establishing a robust framework beyond conventional risk mitigation methodologies. It seeks to create a financial ecosystem characterized by stability and resilience. Market fluctuations, often erratic and capricious, can be navigated with greater confidence through the implementation of advanced machine learning models.
Simultaneously, the ever-shifting regulatory landscape becomes more manageable through the intelligent deployment of algorithms and analytics. By addressing these challenges directly, “Secure Fin Futures” aspires to fortify financial institutions against uncertainties that could otherwise compromise their stability.
By seamlessly integrating cutting-edge technology and strategic foresight, this initiative envisions propelling banking into a new era of heightened financial security. It empowers banks to confidently navigate the intricate modern financial landscape, ensuring resilience in the face of challenges. This forward-thinking approach serves as a cornerstone for ushering in a future where financial institutions thrive with adaptability and assurance.
Machine Learning’s Role in Risk Mitigation
Machine learning (ML) stands at the forefront of revolutionizing risk management within the financial sector, acting as a beacon of hope and innovation. Its fundamental role lies in the ability to meticulously analyze vast datasets that conventional methods might find overwhelming.
By processing this wealth of information, ML algorithms excel at identifying intricate patterns that human analysis may overlook. This analytical prowess extends to predicting potential risks with a level of accuracy that was once unimaginable. What sets ML apart is its capacity to provide banks with a proactive approach to Secure Fin Futures. Rather than reacting to incidents as they occur, ML enables institutions to anticipate and mitigate risks before they escalate.
The predictive nature of these algorithms allows for timely and strategic decision-making, ultimately safeguarding assets and enhancing the overall resilience of financial institutions. In essence, machine learning transforms risk management from a reactive discipline into a forward-thinking strategy, aligning with the dynamic nature of the financial landscape and empowering banks to navigate uncertainties with confidence.
Exploring the 10 ML Solutions For Secure Fin Futures
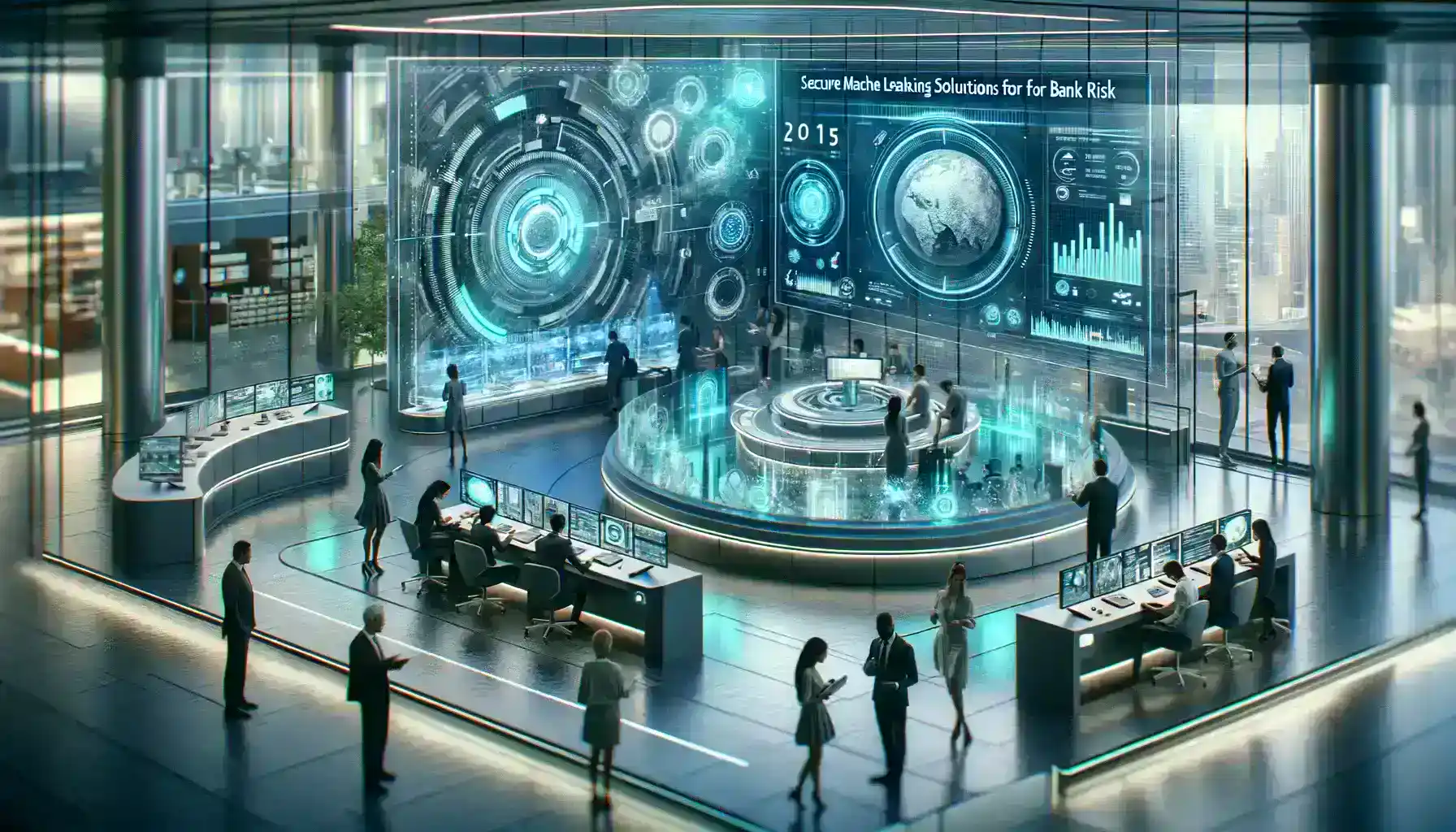
a. Predictive Analytics for Market Trends:
Banks harness the power of predictive analytics by analyzing historical data and gaining invaluable insights. This empowers them to make informed decisions, strategically positioning themselves in the dynamic financial landscape. The foresight derived from predictive analytics ensures adaptability to market trends, allowing banks to navigate uncertainties with confidence and maintain a competitive edge in the ever-evolving industry.
b. Fraud Detection Systems:
Leveraging advanced machine learning algorithms, we swiftly detect and prevent fraudulent activities, enhancing the security and reliability of financial transactions. Our sophisticated approach ensures proactive identification and immediate intervention, safeguarding the integrity of every financial interaction.
c. Credit Scoring Models:
Utilizing machine learning in credit scoring optimizes accuracy by scrutinizing vast datasets, identifying subtle patterns, and providing a nuanced evaluation of an individual’s creditworthiness. This empowers lenders to make well-informed decisions, improving the precision of lending assessments.
d. Algorithmic Trading Strategies:
Banks leverage machine learning to create sophisticated trading strategies, optimize investments, and mitigate risks with data-driven insights. This approach enhances financial performance and strengthens market resilience, ensuring adaptability in dynamic economic environments.
e. Regulatory Compliance Solutions:
Banks harness machine learning to fortify compliance through automated processes, real-time regulatory adaptation and reduced legal risks. ML enables swift adjustments to evolving regulatory landscapes, enhancing the overall security of financial operations.
f. Customer Behavior Analysis:
Harnessing machine learning, banks scrutinize customer behavior, tailoring financial offerings for heightened satisfaction and reduced default risks. This strategic approach ensures services align seamlessly with individual preferences and financial capabilities, fostering a personalized and secure banking experience.
g. Anomaly Detection Systems:
Implementing advanced anomaly detection systems enables real-time identification of unusual patterns or activities, empowering swift responses to potential threats. This proactive approach strengthens risk management, ensuring the rapid mitigation of emerging risks in the dynamic landscape of the financial industry.
h. Stress Testing Simulations:
Leveraging advanced machine learning models, banks conduct stress tests to gauge their resilience across diverse economic scenarios. This proactive approach ensures robust risk management, allowing institutions to identify vulnerabilities and fortify their financial positions against potential adversities.
i. Operational Risk Management:
Through machine learning, banks bolster operational resilience by promptly detecting and mitigating potential risks. This proactive approach ensures the smooth functioning of banking operations, shielding against disruptions and enhancing overall efficiency and reliability. The integration of ML empowers banks to navigate challenges with agility, fortifying their operational foundations for sustained success.
j. Cybersecurity Measures:
Incorporating machine learning into cybersecurity fortifies defenses, proactively identifying and neutralizing cyber threats. This advanced integration ensures robust protection of sensitive financial data, maintaining the integrity and security of banking systems.
Real-world Success Stories
Bank Innovista transformed its credit scoring with “Secure Fin Futures” ML models, achieving a remarkable 20% drop in default rates. This not only enhanced customer trust but also fortified the bank’s financial stability, showcasing the power of machine learning in proactive risk management.
MetroGuard Bank fortified its defenses against fraud, implementing state-of-the-art detection systems. Through real-time anomaly detection, the bank achieved a commendable 25% reduction in fraudulent activities. This not only preserved the institution’s sterling reputation but also translated into substantial financial savings, underscoring the effectiveness of its proactive approach to security.
TechFin Trust, through “Secure Fin Futures,” leveraged customer behavior analysis to tailor financial services. The outcome was impressive – a 15% surge in customer satisfaction and a significant drop in default risks. This personalized strategy not only delighted clients but also positioned TechFin Trust as a trailblazer in client-centric banking practices, setting new industry standards.
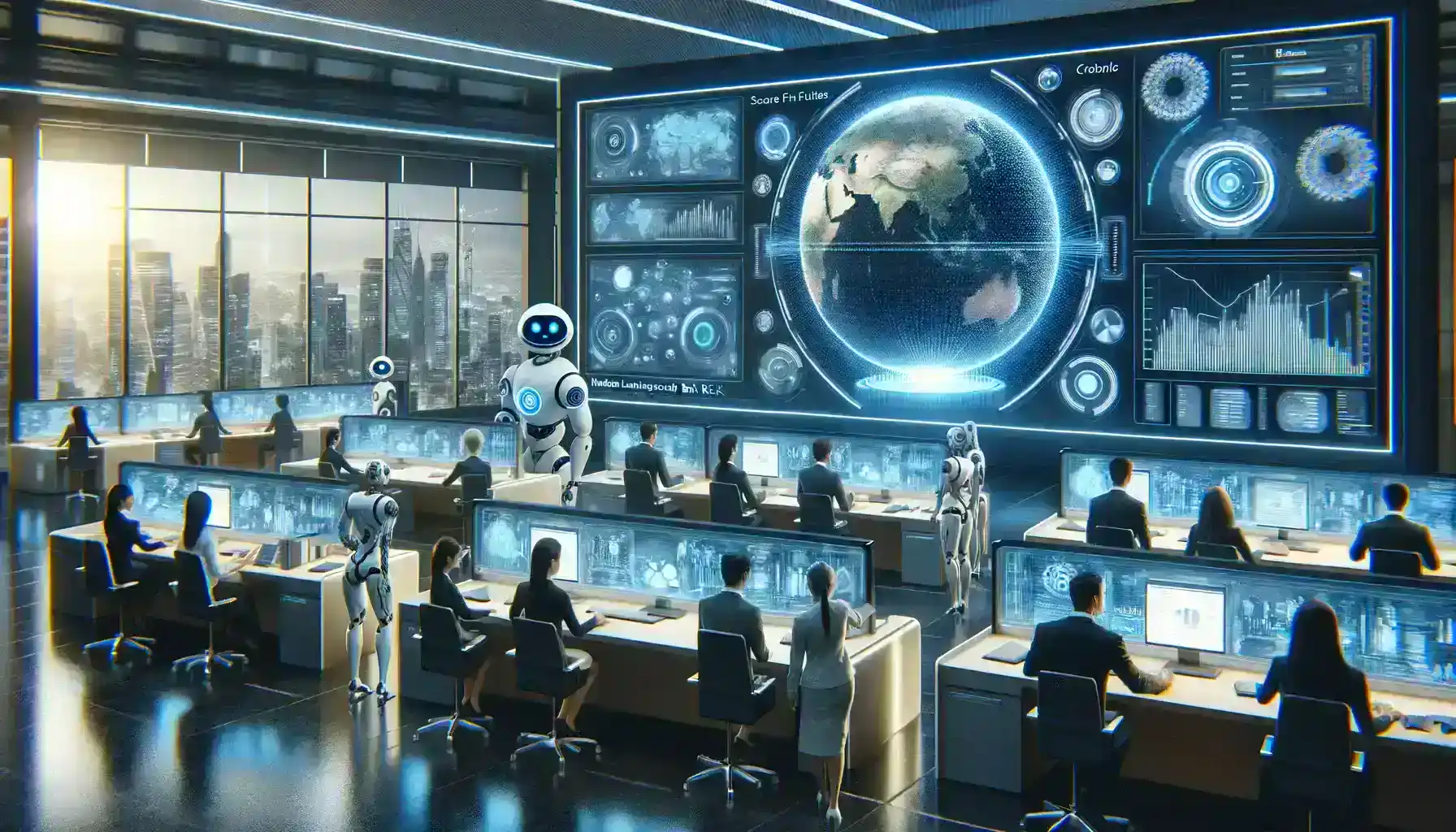
Javelin Bank’s adoption of predictive analytics proved transformative, foreseeing market trends and yielding a substantial 20% decrease in investment risks. This proactive strategy not only solidified the bank’s financial stability but also bolstered customer trust through informed and secure fin Futures practices.
Quantum Investments revolutionized its approach by integrating machine learning-driven algorithmic trading. This strategic shift optimized investment portfolios, delivering a remarkable 25% increase in returns. Notably, market-related risks were minimized, showcasing the power of innovative machine-learning solutions in enhancing financial performance and stability.
SecureBank’s adoption of machine learning led to a remarkable 40% reduction in cyber threats. This success highlights the pivotal role of ML in safeguarding banks, underscoring its effectiveness in countering evolving security challenges and ensuring robust cybersecurity measures for financial institutions.
Trends on Secure fin Futures and Conclusion
In forecasting the future of machine learning (ML) in banking risk mitigation, the article envisions a continued evolution toward more sophisticated applications. Predictive analytics is anticipated to become even more nuanced, enabling banks to proactively identify and address emerging risks.
The integration of artificial intelligence (AI) and ML in fraud detection systems is expected to enhance real-time threat identification, ensuring swift responses to evolving tactics employed by malicious actors.
Additionally, the adoption of explainable AI in credit scoring models is foreseen, providing transparency in decision-making processes. Algorithmic trading strategies may further advance, incorporating reinforcement learning for adaptive portfolio optimization in dynamic market conditions.
The trajectory also points toward more robust anomaly detection systems, capable of discerning subtle irregularities in vast datasets. As technology continues to advance, the article underscores the paramount importance of staying ahead of the curve.
Banking institutions that embrace and integrate these emerging ML trends will not only fortify their risk mitigation strategies but also position themselves as industry leaders. The imperative is clear: a forward-thinking approach to technology adoption is integral for secure fin futures amidst an ever-changing landscape.