Nvidia H200: A breakthrough GPU for generative AI models
Table of Contents
What is the H200 and why is it important?
Nvidia has announced the Nvidia H200, a new graphics processing unit (GPU) that is designed to handle the training and deployment of large and complex artificial intelligence (AI) models. The H200 is an improvement over the H100, which was used by OpenAI to train its state-of-the-art language model, GPT-4.
The H200 is aimed at the generative AI market, which is growing rapidly as more companies, startups and government agencies are using AI models to create new content, such as text, images and predictions. These models require a lot of computing power and memory to train and run, and there is a high demand for the GPUs that can support them.
How does the H200 compare to the H100 and other GPUs?
The H100 was one of the most powerful GPUs for AI training when it was released in 2022, but it had some limitations for AI deployment, or inference. Inference is the process of using a trained model to generate output, such as writing a text or producing an image. The H100 had 80GB of memory, which was insufficient to accommodate some of the chip’s largest models.
The Nvidia H200 solves this problem by having 141GB of memory, which is based on the next-generation “HBM3” technology. This enables the H200 to perform inference more quickly and efficiently than the H100. Based on a test using Meta’s Llama 2 LLM, a large language model, Nvidia claims that the H200 can generate output nearly twice as fast as the H100.
The H200 also has other features that make it more suitable for generative AI applications, such as improved tensor cores, which are specialized units that perform the mathematical operations needed for AI. The H200 has 512 tensor cores, compared to 384 in the H100, and they can operate at higher precision and speed.
The H200 is expected to ship in the second quarter of 2024, and it will compete with AMD’s MI300X GPU, which was also announced recently. The MI300X has similar specifications to the H200, such as 128GB of HBM3 memory and 512 tensor cores, and it is also designed for AI training and inference.
What are the advantages and challenges of the H200?
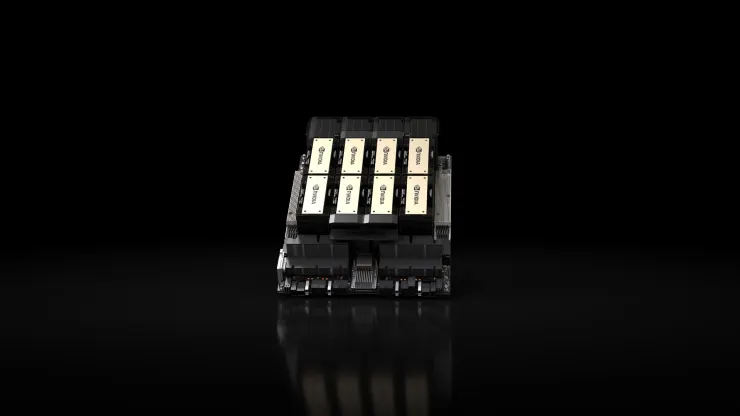
One advantage that Nvidia has over AMD is that the Nvidia H200 is compatible with the H100, meaning that customers who already have the H100 can easily upgrade to the H200 without changing their hardware or software. The H200 will be available in four-GPU or eight-GPU server configurations on Nvidia’s HGX complete systems, as well as in a chip called GH200, which combines the H200 GPU with an Arm-based processor.
However, Nvidia may not be able to maintain its lead in the AI GPU market for long, as it faces competition from other chipmakers, such as Intel and Graphcore, as well as new architectures and technologies that could offer more performance and efficiency. Nvidia itself is planning to release a new GPU, the B100, based on the Blackwell architecture, in 2024, which could be a major leap forward for AI computing.
How does the Nvidia H200 compare to other Nvidia GPUs?
The Nvidia H200 is the most recent and powerful GPU for generative AI and high-performance computing (HPC) workloads. It has the same Hopper architecture as the H100, but much more memory capacity and bandwidth, as well as improved tensor core performance.
The H200 has 141GB of HBM3e memory, which is nearly double the capacity of the H100, which had 80GB of HBM3 memory. The H200 also has 4.8 TB/s of memory bandwidth, which is 1.4X more than the H100, which had 3.35 TB/s. This means that the H200 can handle larger and more complex AI models, such as large language models (LLMs) and generative models, faster and more efficiently than the H100.
The Nvidia H200 also has 512 tensor cores, compared to 384 in the H100, and they can operate at higher precision and speed. Tensor cores are specialized units that perform the mathematical operations needed for AI. The H200 can deliver up to 2 TFLOPS of AI compute per GPU, which is the same as the H100, but it can achieve higher throughput and lower latency for AI inference, which is the process of using a trained model to generate output, such as text, images or predictions.
According to Nvidia, the H200 can generate output nearly twice as fast as the H100 for LLMs like Llama2, and up to 110X faster than CPUs for HPC applications like simulations, scientific research and artificial intelligence.
Featured Insights
How to Build a Custom CRM (Customer Relationship Management)
AI Agriculture Breakthrough: RhizoNet Ushers in a New Era of Farming
Framework vs Library: Key Differences Every Developer Should Know
The Gemini AI and Google AI Features that We Have Been Waiting For
AI Prompt Engineering 2.0: Why Static Prompts Are a Thing of the Past
Vision Pro: Apple’s AI Plans Extend to the Headset