Table of Contents
In the realm of artificial intelligence, multimodal models have emerged as a powerful tool capable of creating diverse and rich content by understanding and generating across multiple modalities, such as text, images, and even audio. This step-by-step guide aims to elucidate the process of leveraging multimodal AI effectively.
What is Multimodal AI?
Multimodal AI is an advanced form of artificial intelligence that can understand and create content across various modalities like text, images, and audio simultaneously. It combines information from different sources using neural network architectures, allowing it to generate richer, contextually relevant outputs.
Models like DALL-E by OpenAI exemplify this capability, creating images from text inputs. This technology finds applications in diverse fields like creative design, content generation, virtual assistants, and healthcare, offering deeper insights into data and producing more expressive and diverse outputs.
Understanding Multimodal AI
Multimodal AI involves the fusion of various data types to generate content. It employs neural networks and sophisticated algorithms to comprehend and create across multiple domains, allowing for a more holistic understanding of the data.
Step 1: Familiarize Yourself with Multimodal Models
Familiarizing oneself with Multimodal Models is an essential preliminary step in navigating the complexities of these advanced AI architectures. These models, such as CLIP (Contrastive Language-Image Pretraining) and DALL-E, amalgamate diverse data types like text and images, enabling the generation of contextually rich and diverse content. Understanding the underlying principles, architectures, and capabilities of these models is crucial for users aiming to harness their potential.
Step 2: Gather High-Quality Data
The quality of data significantly influences the output of models. Collect diverse datasets that encompass various modalities relevant to your project, ensuring they are labeled and organized efficiently.
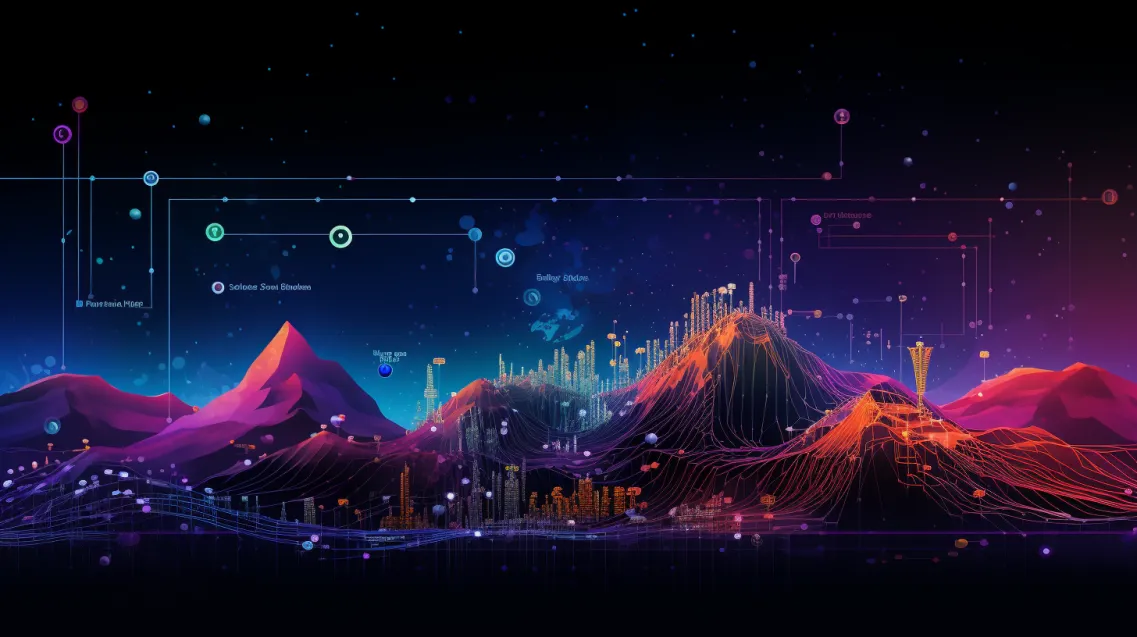
Step 3: Preprocessing and Data Preparation
Preprocessing and data preparation are fundamental steps in multimodal AI. In this phase, collected datasets undergo crucial transformations to ensure they align with the model’s requirements. For text data, processes such as tokenization, normalization, and encoding are applied to convert textual information into a format understandable by the AI model. For image data, resizing, normalization, and standardization procedures are implemented to unify image sizes and enhance model compatibility.
Step 4: Selecting and Fine-Tuning the Model
Choose an appropriate multimodal model based on your project’s requirements. Fine-tune the chosen model using transfer learning on your specific dataset to enhance its performance and adaptability to your task.
Step 5: Training the Model
Train the fine-tuned multimodal model using the prepared dataset. This step might require substantial computational resources and time, depending on the complexity and size of your data.
Step 6: Generating Content
Once the model is trained, use it to generate multimodal content. Input text prompts or image descriptions to the model and observe the diverse outputs it generates across different modalities.
Step 7: Evaluation and Iteration
Evaluation and iteration are crucial phases in leveraging multimodal AI effectively. Once content is generated, it undergoes evaluation based on predefined metrics or human judgment to assess its quality, relevance, and adherence to the intended purpose. This evaluation phase helps identify any biases, inconsistencies, or shortcomings in the generated outputs.
Subsequently, iterative improvements are made to the model by incorporating feedback, adjusting parameters, or expanding the dataset to enhance the quality and diversity of the generated content.
Step 8: Ethical Considerations
Ethical considerations are paramount in the development and application of multimodal AI. The technology’s potential to create diverse content across multiple modalities demands a conscientious approach to mitigate ethical risks. Ensuring fairness and preventing biases in generated outputs is crucial.
AI models can inadvertently perpetuate societal prejudices present in the training data. Safeguarding against the generation of misleading, harmful, or inappropriate content is essential to prevent potential misuse. Transparency in the use of AI-generated content, disclosure of its origins, and clear delineation between human and AI-generated content are imperative for ethical deployment.
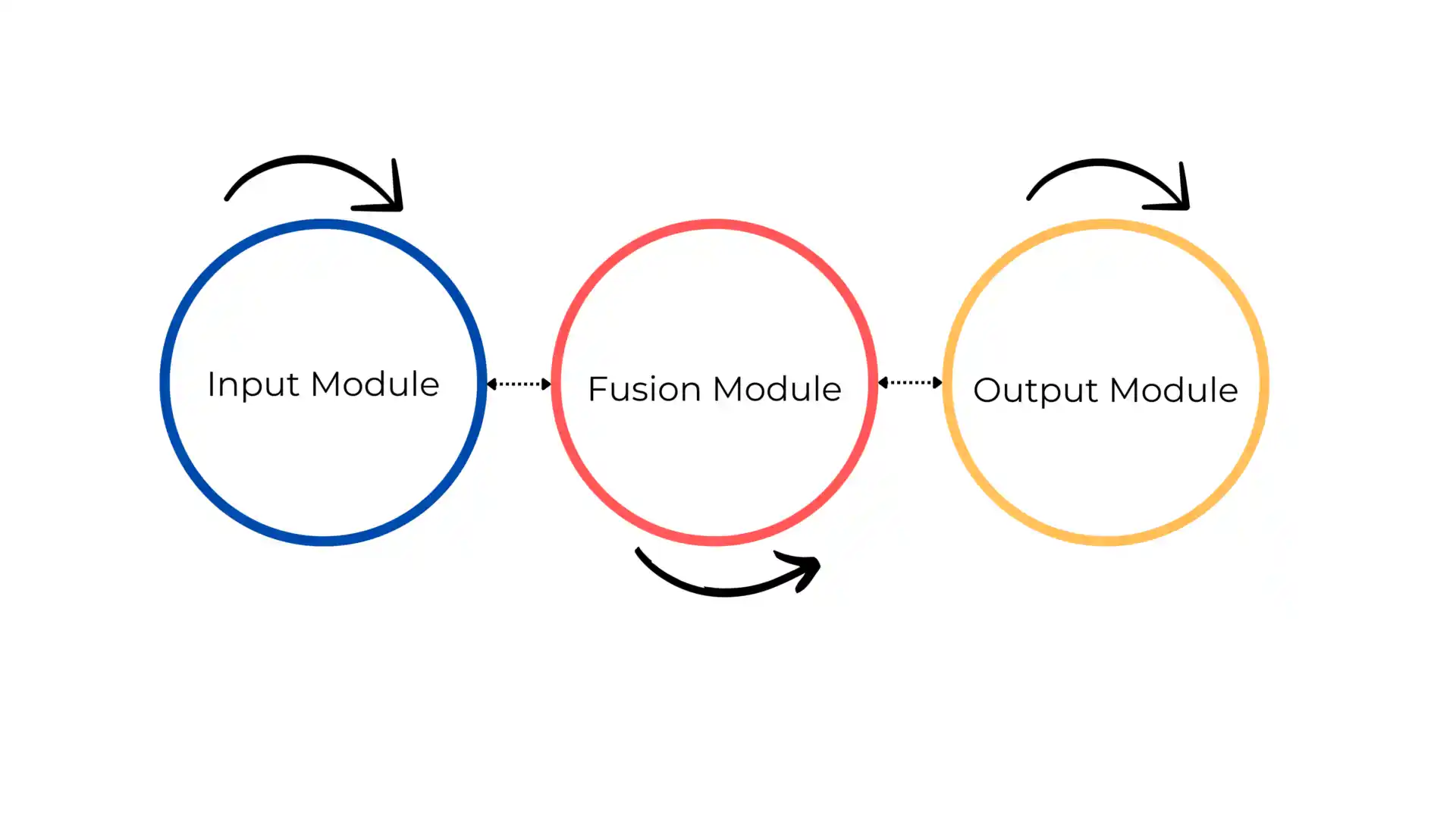
Step 9: Deployment and Integration
Deploying and integrating multimodal AI involves several key steps to ensure its seamless application. Once the model is trained and refined, it needs to be prepared for deployment in the desired environment or platform.
This involves optimizing the model for performance, scalability, and user experience considerations. Integration into existing systems or applications requires careful planning and compatibility checks to ensure smooth functionality. Additionally, ongoing monitoring and maintenance are crucial to address any issues that may arise post-deployment.
Step 10: Continuous Learning and Updates
Continuous learning and updates are fundamental elements in the realm of Multimodal AI, ensuring the perpetual evolution and enhancement of these sophisticated models. In this dynamic landscape, staying abreast of the latest advancements, research breakthroughs, and iterative improvements is imperative.
Continuous learning involves assimilating new data, refining existing models, and adapting to emerging trends to bolster the model’s adaptability. Efficiency, and performance. Regular updates not only fortify the model’s capabilities but also aid in addressing biases, improving content quality, and aligning with ethical considerations.
Embracing a culture of ongoing learning and updates in Multimodal AI enables practitioners to push the boundaries of innovation and drive meaningful progress in various applications across multiple modalities.
Conclusion
Multimodal AI presents unparalleled opportunities for creating diverse and contextually rich content across multiple modalities. By following these steps, one can harness the power of this technology effectively, paving the way for innovative applications in various fields, including art, design, communication, and more.
However, responsible usage and ethical considerations should always accompany the deployment of such powerful AI tools to ensure their positive impact on society.
As you embark on your journey with multimodal AI, remember that experimentation, exploration, and continual learning are pivotal in unlocking the full potential of this groundbreaking technology.
FAQs on Utilizing Multimodal AI
1. What are the primary benefits of using multimodal AI?
Multimodal AI offers the capability to generate diverse content across multiple modalities like text, images, and audio. Its primary benefits include the creation of contextually rich and varied content, aiding in tasks such as content generation, artistic creation, and problem-solving across diverse domains.
2. How crucial is data quality in training multimodal models?
Data quality significantly influences the performance of models. High-quality, diverse datasets are vital for training models effectively. Ensuring accurate labels, proper organization, and relevance to the task at hand is crucial for obtaining desirable outputs.
3. What steps are involved in deploying a multimodal AI model?
Deploying a multimodal AI model involves several key steps. These include model selection and fine-tuning, training on prepared datasets, generating content, evaluating outputs, addressing ethical considerations, deploying the model in the desired application or platform, and continuously updating and refining the model based on ongoing learning and advancements.