Table of Contents
Introduction to MLCommons AI Benchmarks
As the influence of artificial intelligence (AI) continues to shift from centralized cloud computing to on-device applications, the challenge of determining the optimal performance of consumer PCs for specific AI-powered tasks becomes increasingly critical. The ability to discern whether a new laptop, desktop, or all-in-one will execute generative AI-powered applications more efficiently than its counterparts can make a substantial difference in user experience and productivity.
Recognizing this need, MLCommons, a prominent industry group shaping AI-related hardware benchmarking standards, has embarked on an initiative to simplify the process of comparing consumer PCs. The group has introduced performance benchmarks aimed at “client systems,” encompassing desktops, laptops, and workstations running various operating systems, including Windows and Linux.
MLPerf Client Working Group Formation
In a recent announcement, MLCommons revealed the establishment of a new working group named MLPerf Client. This working group’s primary objective is to define and implement AI benchmarks tailored for client systems, thereby offering consumers a standardized metric to evaluate and compare the AI performance of their devices. Unlike traditional benchmarks, MLPerf Client’s benchmarks are described as “scenario-driven,” meaning they focus on real-world end-user use cases.
This approach ensures that the benchmarks align closely with practical applications, providing users with insights into how their consumer PCs will perform in everyday scenarios. Furthermore, MLCommons emphasizes that these benchmarks are developed based on feedback from the community, reflecting a collaborative effort to address the evolving needs of users.
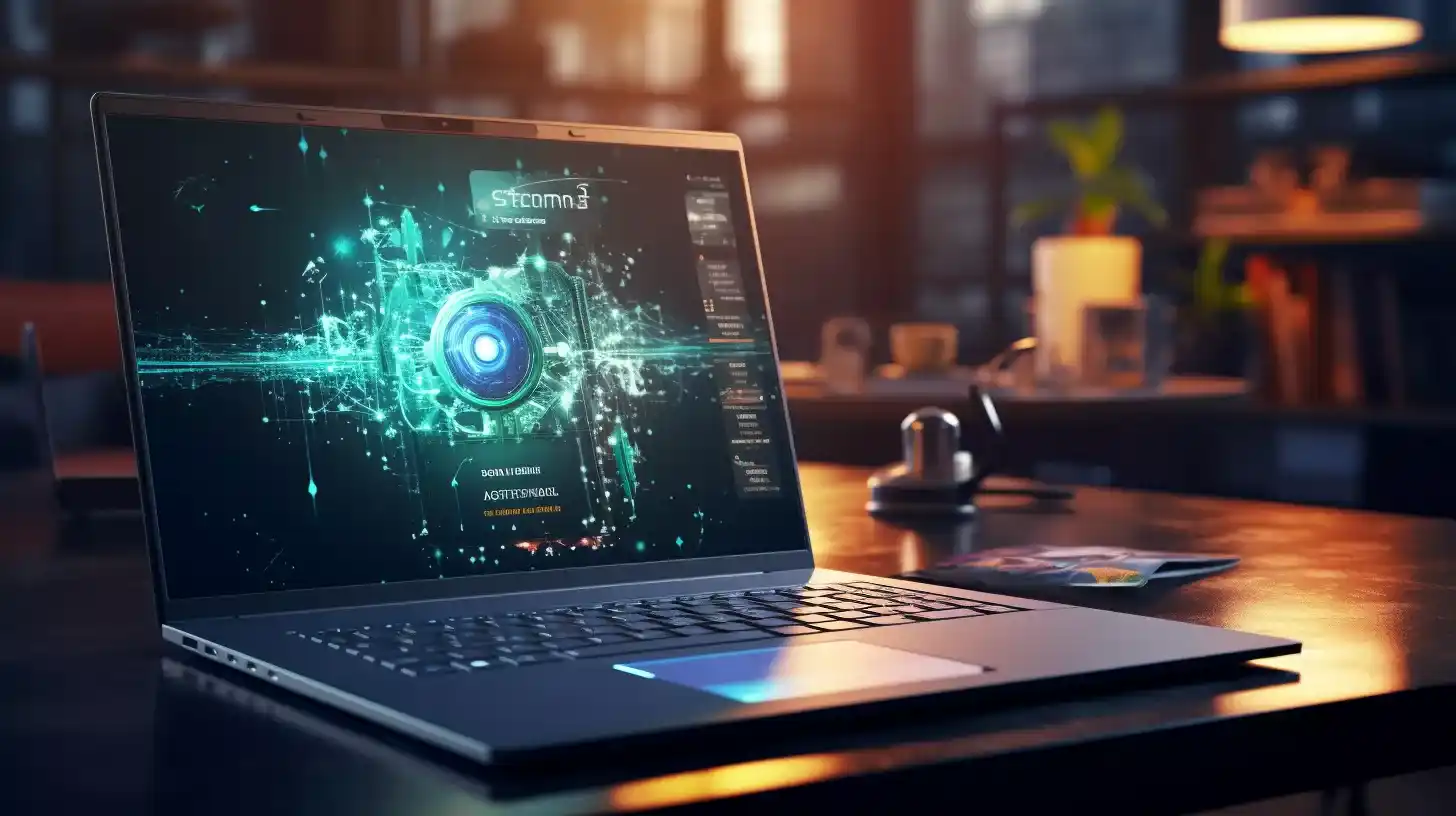
Real-World Use Cases and Community Feedback
MLCommons recognizes the importance of benchmarks that go beyond theoretical metrics and resonate with real-world applications. As AI applications become integral to various aspects of daily life, the benchmarks introduced by MLPerf Client will concentrate on scenario-driven evaluations.
This approach involves considering specific user interactions and tasks, such as text generation, which can have a direct impact on user experience. By grounding the benchmarks in real end-user use cases, MLCommons aims to provide consumers with practical insights into the performance differences among consumer PCs.
Focus on Text-Generating Models
The first benchmark introduced by MLPerf Client targets text-generating models, with a specific emphasis on Meta’s Llama 2. This choice is not arbitrary; it stems from Llama 2’s prominence and relevance in the AI landscape. MLCommons executive director David Kanter highlights that Llama 2 has already been integrated into MLCommons‘ benchmarking suites designed for data center hardware. This indicates a strategic alignment, ensuring consistency in benchmarking across different hardware categories.
Moreover, Meta has collaborated extensively with Qualcomm and Microsoft to optimize Llama 2 for Windows, showcasing a commitment to enhancing the performance of text-generating models on Windows-running devices. This collaborative effort further reinforces the practicality and applicability of the benchmarks to the diverse ecosystem of consumer PCs.
The Importance of Timely and Relevant Benchmarks
MLCommons acknowledges the significance of timely and relevant benchmarks, especially in a rapidly evolving technological landscape. With AI workloads moving closer to end-user devices, the benchmarks developed by MLPerf Client become essential tools for consumers, enabling them to make informed decisions about their device purchases.
The benchmarks address the critical factor of time, emphasizing the potential impact on user experience by illustrating the speed differences in executing AI tasks. As the saying goes, time is money, and in the realm of AI-powered applications, the efficiency of text generation or similar tasks can be a decisive factor for users.
Conclusion
MLCommons’ initiative through the formation of the MLPerf Client working group represents a significant step towards providing consumers with standardized benchmarks for evaluating AI performance on consumer PCs. By focusing on scenario-driven benchmarks grounded in real-world use cases and incorporating community feedback, MLPerf Client aims to offer a comprehensive and practical metric for comparing client systems.
The specific emphasis on text-generating models, particularly Meta’s Llama 2, showcases a strategic approach to aligning with prominent AI technologies. As AI continues to become an integral part of our daily computing experiences, MLPerf Client’s benchmarks are poised to play a pivotal role in empowering consumers to make informed decisions and ensuring that their AI-powered devices deliver optimal performance.