Table of Contents
In today’s rapidly evolving healthcare landscape, the integration of ML in Healthcare stands as a transformative force, reshaping patient care and outcomes. As a subset of artificial intelligence (AI), ML algorithms empower computers to sift through vast datasets, uncovering intricate patterns and insights that elude human observation. This capability has propelled ML to the forefront of healthcare innovation, facilitating swift data processing and informed decision-making.
ML classifications in healthcare represent a cornerstone of modern healthcare analytics, encompassing a diverse array of applications. From disease diagnosis and personalized treatment planning to patient risk stratification and medication adherence monitoring, ML algorithms offer unparalleled assistance to healthcare professionals across various domains. By leveraging patient data and evidence-based guidelines, ML-based Clinical Decision Support Systems (CDSS) augment clinical expertise, improving diagnostic accuracy, treatment efficacy, and overall patient outcomes.
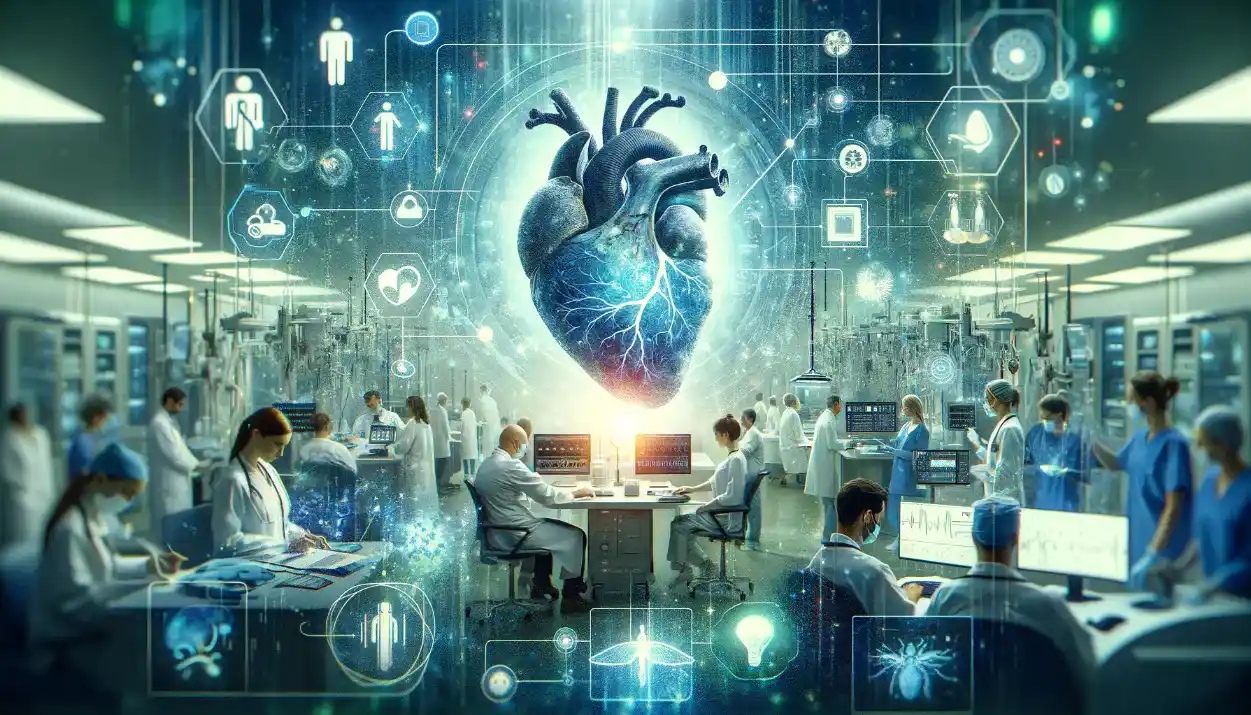
The exponential growth of ML in healthcare underscores its indispensable role in driving advancements and efficiencies within the industry. Through predictive analytics, genomic data analysis, and population health management, ML enables healthcare organizations to harness the full potential of data-driven insights, ultimately delivering more precise, personalized, and effective care to patients. As ML continues to evolve and permeate every facet of healthcare delivery, its impact on improving health outcomes and transforming the patient experience is poised to be profound and enduring.
1. Disease Diagnosis
ML in healthcare employs algorithms to scrutinize patient symptoms, medical records, diagnostic exams, and imaging findings, aiding in disease classification. ML models excel in categorizing medical images like X-rays and MRIs, supporting radiologists in pinpointing abnormalities suggestive of various conditions such as cancer, fractures, or neurological disorders.
By analyzing intricate patterns within these data sets, ML algorithms enhance diagnostic accuracy and efficiency, facilitating earlier detection and intervention. This application of ML transforms the diagnostic process, enabling healthcare professionals to make well-informed decisions and improve patient outcomes through timely and precise identification of diseases.
2. Patient Risk Stratification
In healthcare, ML algorithms play a pivotal role in patient risk stratification, enabling healthcare providers to assess and prioritize interventions based on individual patient risk profiles. By analyzing diverse patient data, including medical history, demographics, and clinical parameters, ML models can accurately predict the likelihood of specific adverse events or medical conditions.
For example, these algorithms can forecast the risk of hospital readmission, sepsis, or post-surgical complications with high precision. Armed with this predictive insight, healthcare professionals can proactively allocate resources and implement targeted interventions to mitigate risks, ultimately improving patient outcomes and optimizing healthcare resource utilization. ML in healthcare thus serves as a valuable tool for enhancing risk management strategies and delivering personalized care.
3. Treatment Response Prediction
ML in Healthcare models plays a pivotal role in predicting patient responses to treatments or medications, leveraging clinical characteristics and medical history. This predictive capability enables a personalized approach to treatment planning, allowing healthcare providers to tailor interventions according to individual patient needs. By analyzing vast datasets, ML algorithms can identify patterns and correlations that aid in anticipating treatment outcomes, optimizing efficacy, and reducing adverse effects.
This proactive strategy not only enhances patient care but also contributes to more efficient resource utilization within healthcare systems. Ultimately, the integration of ML in healthcare facilitates a shift towards precision medicine, where treatments are tailored to each patient’s unique profile, improving overall therapeutic outcomes and patient satisfaction.
4. Medication Adherence Monitoring
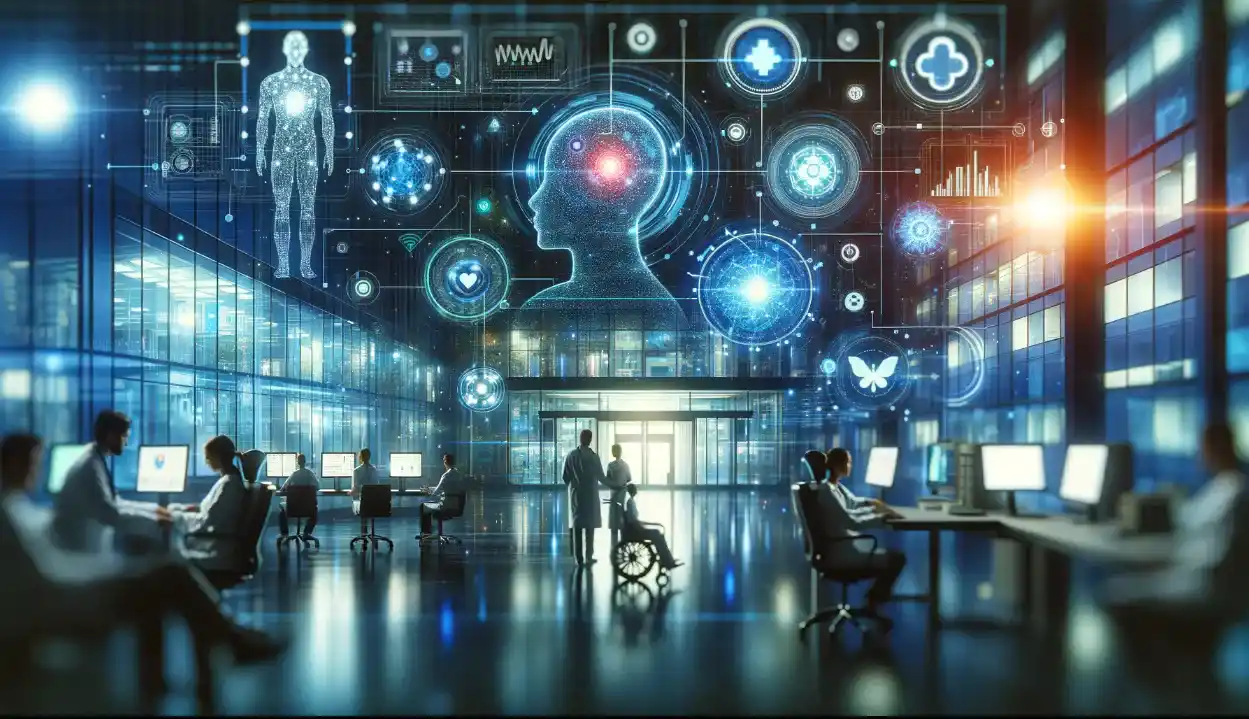
In medication adherence monitoring, ML algorithms utilize a diverse range of patient data, encompassing medication history, demographics, and behavioral patterns. These algorithms meticulously analyze this data to predict and categorize medication adherence levels accurately. By discerning patients at risk of non-adherence, ML in Healthcare empowers healthcare providers to intervene proactively with targeted strategies.
Such interventions are instrumental in enhancing adherence rates and ultimately improving treatment outcomes. ML’s predictive capabilities enable personalized approaches to patient care, ensuring that interventions are tailored to individual needs. Consequently, ML serves as a valuable tool in medication management, fostering better patient adherence and contributing to the overall efficacy of healthcare interventions.
5. Healthcare Fraud Detection
In the realm of Healthcare Fraud Detection, ML techniques stand as a powerful tool for uncovering deceptive practices within billing, insurance claims, and reimbursement processes. ML algorithms meticulously scrutinize vast volumes of data, seeking patterns and anomalies that betray fraudulent behavior.
By delving into billing data intricacies, these algorithms pinpoint suspicious claims, aiding healthcare organizations in staunching financial losses and preserving the integrity of the healthcare system. ML in Healthcare not only fortifies defenses against fraudulent activities but also fosters trust and transparency, ensuring that resources are allocated judiciously and that patient care remains paramount amidst the complexities of healthcare administration.
6. Genomic Data Analysis
Genomic Data Analysis plays a pivotal role in healthcare, where ML algorithms interpret genetic data to identify disease-related genetic variants, predict drug responses, and optimize treatment outcomes. By leveraging ML in healthcare, providers can offer personalized medicine strategies tailored to individual genetic profiles. This approach enhances treatment efficacy while minimizing adverse effects, ultimately improving patient safety and outcomes.
ML’s ability to sift through vast genomic datasets enables healthcare professionals to uncover intricate genetic patterns that may influence disease susceptibility and treatment response. Consequently, integrating ML-driven genomic analysis into clinical practice empowers healthcare providers to make data-driven decisions and deliver more precise and effective treatments to patients, marking a significant advancement in personalized healthcare.
7. Clinical Decision Support Systems
Clinical Decision Support Systems (CDSS) powered by ML in healthcare offer invaluable assistance to healthcare providers by leveraging patient data and evidence-based guidelines to provide recommendations, alerts, and risk assessments. These systems serve as indispensable tools in clinical decision-making, enhancing diagnostic accuracy, treatment planning, and ultimately, patient outcomes.
By analyzing vast amounts of patient data, ML-based CDSS can identify patterns, trends, and correlations that may not be immediately apparent to human clinicians. This data-driven approach augments clinical expertise, providing healthcare professionals with timely and relevant insights to support their decision-making process. As a result, ML-based CDSS contributes to more informed and effective healthcare delivery, improving the quality of care and patient satisfaction.
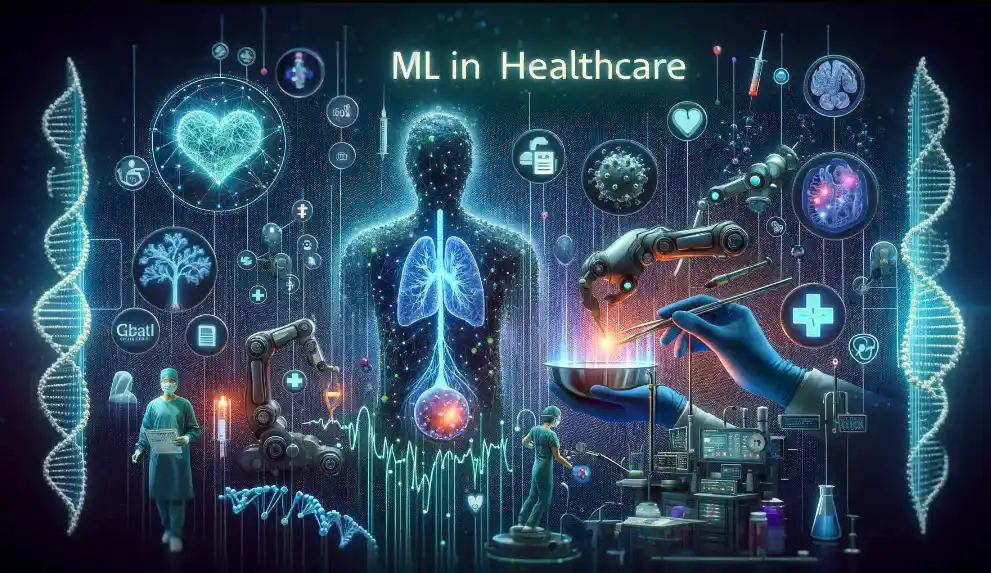
ML in Healthcare: A Paradigm Shift
The integration of ML in healthcare heralds a transformative era, promising unparalleled advancements in patient care and health outcomes. ML-powered solutions span various domains, from predictive analytics and personalized treatment plans to medical imaging interpretation and population health management, revolutionizing healthcare delivery. As healthcare organizations embrace the potential of ML, they must uphold ethical standards, prioritize data privacy, and ensure algorithmic transparency to foster equitable and patient-centered care.
Responsible innovation in ML holds the key to unlocking the full potential of precision medicine, ushering in a future where healthcare is tailored to individual needs and accessible to all. Classification of ML in healthcare empowers organizations with the tools to harness vast troves of healthcare data, driving improvements in patient care, operational efficiency, and outcomes. By leveraging ML algorithms, healthcare providers can glean actionable insights, enabling informed decisions, and personalized treatments, and ultimately, enhancing the overall quality and effectiveness of healthcare delivery.