Table of Contents
Meta AI Video Recommendations: In an era where artificial intelligence (AI) is reshaping the digital landscape, Meta has embarked on a groundbreaking journey to revolutionize its video recommendation engine. This initiative is poised to unify the video experience across all its platforms, leveraging the power of AI to deliver content that resonates with users’ preferences.
The Roadmap to 2026: A Vision for Meta AI Video Recommendations
Tom Alison, the head of Facebook, unveiled Meta AI Video Recommendations for an ambitious technology roadmap extending to 2026. Central to this vision is the development of an AI recommendation model capable of powering both the TikTok-like Reels short video service and traditional, longer videos. This marks a strategic shift from Meta’s previous approach of using separate models for different products, such as Reels, Groups, and the core Facebook Feed.
Investing in the Core: GPUs at the Heart of Meta’s AI Strategy
Meta’s foray into AI is backed by a substantial investment in Nvidia graphics processing units (GPUs). These GPUs have become the go-to hardware for AI researchers, essential for training large language models (LLMs) like those powering OpenAI’s ChatGPT and other generative AI models.
Phase 1: Transitioning to GPUs for Enhanced Performance
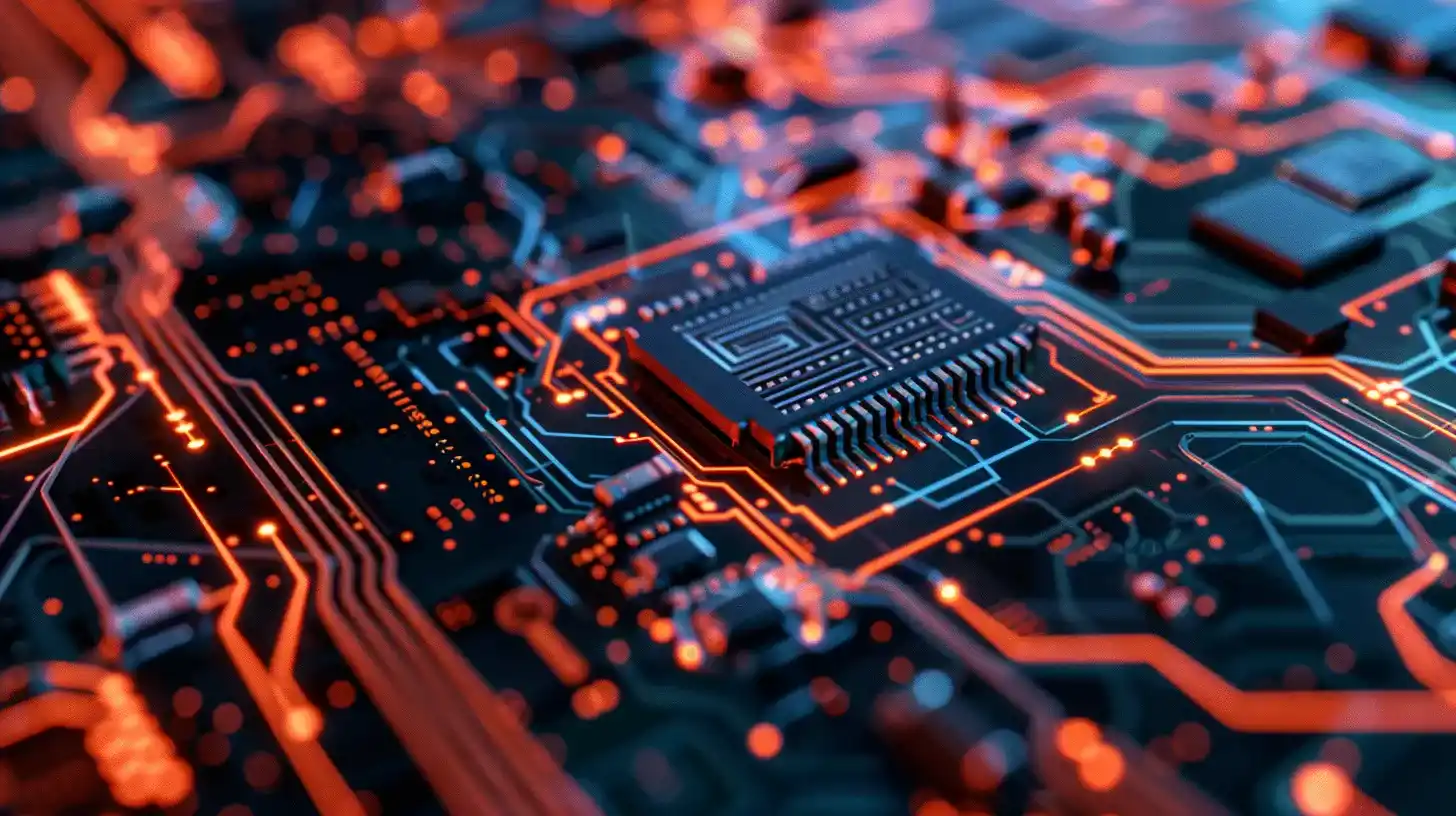
The first phase of the Meta AI Video Recommendations technology overhaul involved transitioning its current recommendation systems to GPUs. This strategic move has significantly improved the performance of its products, setting the stage for more advanced AI applications.
LLMs: The Catalyst for Meta’s AI Revolution
The surge in interest in LLMs last year caught Meta AI Video Recommendations’ attention, particularly their ability to process vast amounts of data and perform general-purpose tasks. This insight led to the conception of a giant recommendation model that could be applied across various products. By last year, Meta had developed a new model architecture and tested it on Reels, resulting in an 8% to 10% increase in watch time on the core Facebook app.
Phase 2: Validating and Expanding the AI Model
Meta AI Video Recommendations is currently in the third phase of its system re-architecture, focusing on validating the technology and extending it across multiple products. The goal is to power the entire video ecosystem with this single model and eventually integrate the Feed recommendation product.
The Future of Content Discovery: Enhanced Engagement and Responsiveness
If successful, this unified AI model will not only make Meta AI Video Recommendations more engaging and relevant but also improve their responsiveness. For instance, if a user enjoys content in Reels, the Feed could then display more similar content, creating a seamless content discovery experience.
Meta’s Generative AI: Beyond Video Recommendations
Meta’s vast GPU stockpile will also support broader generative AI efforts, including developing digital assistants. These projects range from integrating sophisticated chatting tools into the core Feed to enabling users to learn more about topics like Taylor Swift with a simple click.
AI in Social Spaces: The Multiplayer Consumer Environment
Meta envisions placing generative AI in a ‘multiplayer consumer environment.’ For example, within Facebook Groups, a member could ask a question about desserts and receive an answer from a digital assistant, enhancing the community experience.
This rewrite incorporates the focus keyword and related terms throughout the content, ensuring SEO optimization while providing a comprehensive overview of Meta AI Video Recommendations initiatives. If you need further adjustments or additional sections, feel free to let me know!
Understanding Generative AI
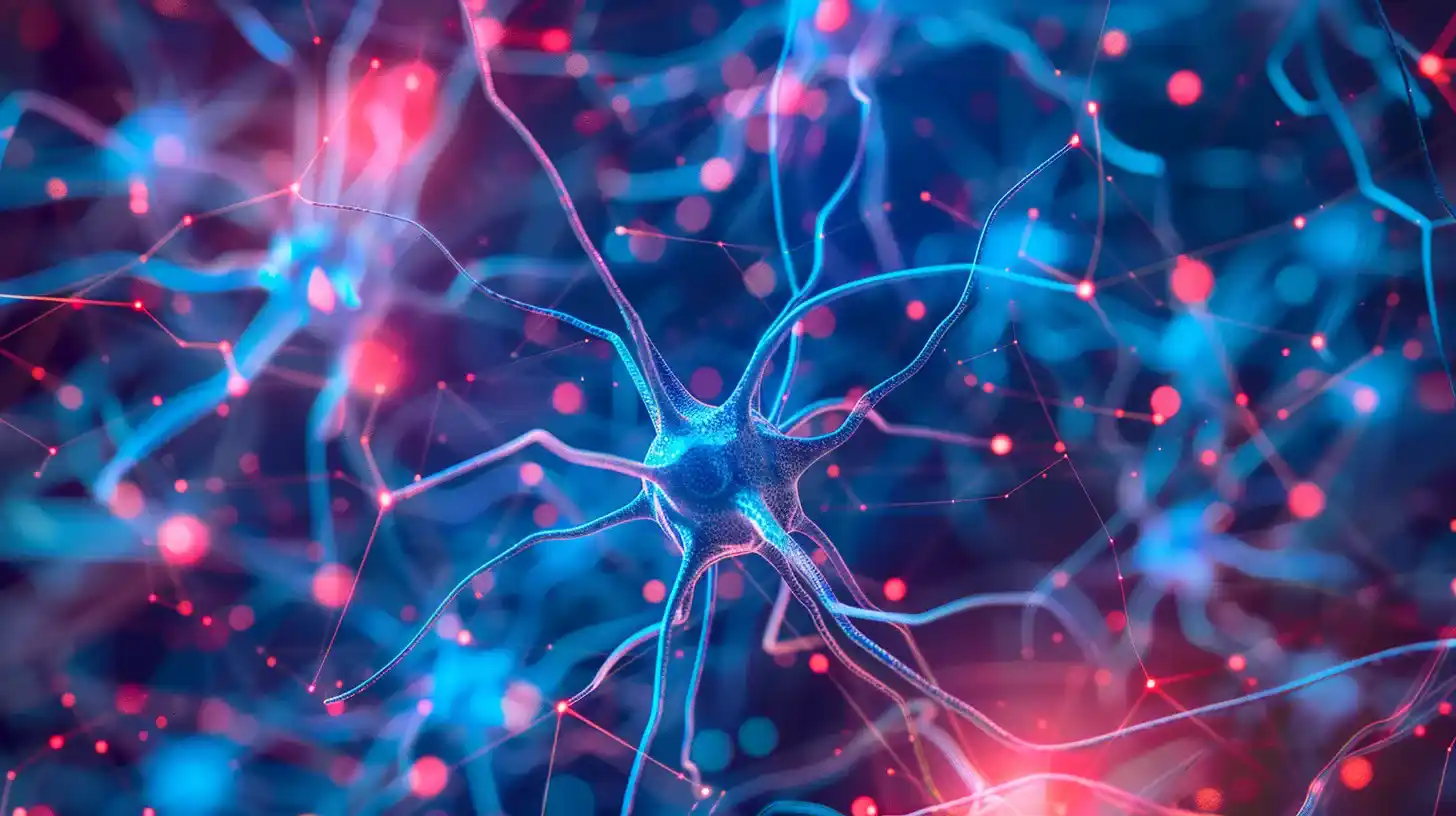
Generative AI models are trained on large datasets to learn patterns, structures, and designs within the data. Once trained, these models can generate new examples that are similar to the training data, effectively creating new content without direct human input. This process involves training AI models to understand different patterns and structures within existing data and using that knowledge to generate new, original data.
How Generative AI Works
A generative model is a type of machine learning model that generates new data instances that resemble those in a given dataset. It learns the underlying patterns and structures of the training data before generating fresh samples. These models are capable of capturing the features and complexity of the training data, allowing them to produce innovative and diverse outputs through Meta AI Video Recommendations
Examples of Generative Models
Some popular architectures for generative models include:
- Generative Adversarial Networks (GANs): These involve two neural networks, a Generator and a Discriminator, that work against each other. The Generator creates new data samples, while the Discriminator evaluates their authenticity.
- Variational Autoencoders (VAEs): These are used for generating new instances that are similar to the input data.
- Autoregressive models: These predict the next data point in a sequence, given the previous data.
- Transformers: These are models that handle sequential data and are known for their effectiveness in natural language processing tasks.
Applications of Generative AI
Generative AI has a wide range of applications, including:
- Image synthesis: Creating new images that don’t exist but look realistic.
- Text generation: Writing articles, stories, or code.
- Music composition: Generating new music pieces.
- Data augmentation: Enhancing datasets to improve machine learning models.
Impact of Generative AI
The rise of generative AI has been significant due to its ability to create content in response to natural language prompts, making it a versatile tool across various industries. It’s being used for writing assistance, research, coding, designing, and more.
Generative AI continues to evolve as it trains on more data, becoming more sophisticated and capable of producing results that closely mimic human creativity and decision-making processes.