Table of Contents
Machine learning is a branch of artificial intelligence that enables systems to learn from data and improve performance without explicit programming. A supply chain is the network of activities, processes, and resources that deliver value to customers, from raw materials to finished products.
Machine learning can help supply chain management in various ways, such as optimizing inventory, forecasting demand, reducing costs, enhancing quality, and improving customer satisfaction.
In this blog post, we will explore the concepts, trends, and applications of machine learning in supply chain, as well as the best practices and challenges for its implementation.
What is Machine Learning in Supply Chain?
Machine learning in supply chain is the application of machine learning techniques and algorithms to supply chain data and problems. Machine learning can help supply chain managers and practitioners analyze large and complex data sets, extract meaningful insights, and make data-driven decisions. Machine learning can also enable supply chain systems to adapt and respond to changing conditions, such as market fluctuations, customer preferences, and environmental factors.
Different machine learning techniques can be used in supply chain, such as supervised, unsupervised, and reinforcement learning.
- Supervised learning is when the system learns from labeled data, such as historical sales and demand data, and predicts the outcomes, such as future demand and sales.
- Unsupervised learning is when the system learns from unlabeled data, such as customer feedback and reviews, and discovers patterns, such as customer segments and preferences.
- Reinforcement learning is when the system learns from its actions and feedback, such as inventory levels and costs, and optimizes its behavior, such as inventory replenishment and pricing.
Machine learning in supply chain requires a lot of data, which can come from various sources, such as internal systems, external partners, and third-party platforms. However, data quality, availability, and integration are some of the major challenges for machine learning in the supply chain, as the data can be noisy, incomplete, inconsistent, or outdated. Therefore, data cleaning, preprocessing, and standardization are essential steps for machine learning in supply chain environment.
What are the Current Trends in Machine Learning Supply Chain?
Machine learning in the supply chain is evolving rapidly, and many current trends and applications are transforming the supply chain industry. Some of the most prominent ones are:
- Demand forecasting: By combining multiple data sources, such as historical sales, market trends, weather, events, and social media, machine learning can help to improve the accuracy and reliability of demand forecasting. Machine learning can also assist in capturing the nonlinear and dynamic relationships between demand and various factors, as well as in dealing with demand uncertainty and variability. For example, Walmart forecasts demand for over 500 million items across its stores and e-commerce platforms using machine learning.
- Inventory optimization: By balancing the trade-off between service level and inventory cost, machine learning can assist in optimizing inventory levels and locations. By utilizing data such as shelf life, expiration date, and quality, machine learning can also help to reduce inventory obsolescence, shrinkage, and waste. Amazon, for example, uses machine learning to improve inventory management and fulfillment by analyzing data such as customer orders, product availability, and shipping options.
- Supply chain network design: Machine learning can assist in the design and configuration of the supply chain network by taking into account the optimal number, location, and capacity of facilities such as suppliers, factories, warehouses, and distribution centers. Machine learning can also assist in weighing the benefits and drawbacks of various network design scenarios, such as centralized vs. decentralized, single vs. multiple sourcing, and make-to-stock vs. make-to-order. Procter & Gamble, for example, uses machine learning to design and optimize its global supply chain network by analyzing data such as demand, cost, service, and risk.
- Quality control: Machine learning can help to improve the quality and consistency of products and processes by detecting and preventing defects, errors, and anomalies. Machine learning can also help to improve the quality of inspection and testing by utilizing data such as images, videos, and sensors. BMW, for example, uses machine learning to monitor and improve the quality of its car production by utilizing data from cameras, lasers, and microphones.
- Supplier relationship management: Machine learning can help to improve the quality and consistency of products and processes by detecting and preventing defects, errors, and anomalies. Machine learning can also help to improve the quality of inspection and testing by utilizing data such as images, videos, and sensors. BMW, for example, uses machine learning to monitor and improve the quality of its car production by utilizing data from cameras, lasers, and microphones.
These are some of the current trends and applications of machine learning in supply chain, but they are not exhaustive. Machine learning can also be applied to other areas of the supply chain, such as transportation, logistics, procurement, and customer service.
However, each trend and application has its benefits and challenges and requires different levels of data, technology, and expertise. Therefore, it is important to follow the best practices and recommendations for machine learning in supply chain, such as:
- Define the business problem and objective clearly and precisely
- Identify and collect the relevant and reliable data sources and features
- Choose and apply the appropriate machine-learning technique and algorithm
- Validate and test the machine learning model and results
- Deploy and monitor the machine learning solution and performance
- Update and improve the machine learning solution and performance
What are the Future Applications of Machine Learning in Supply Chain?
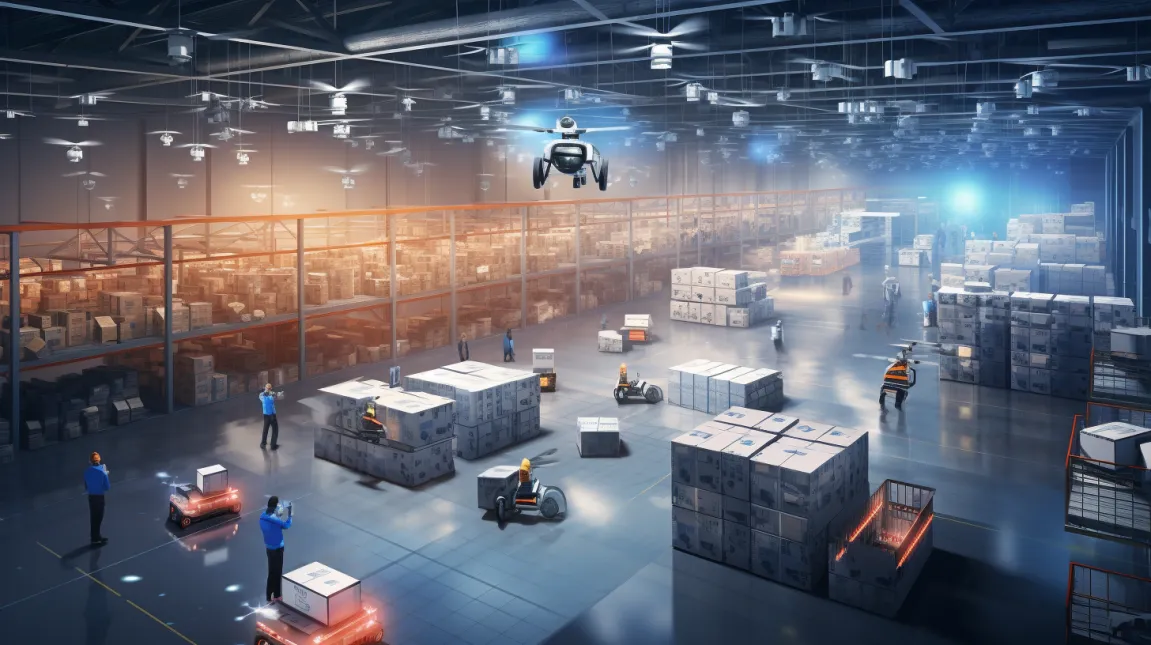
Machine learning in supply chain is not only a current trend, but also a future opportunity. Machine learning can help to create new and innovative applications and solutions for the supply chain, that can improve the supply chain performance and efficiency, as well as customer satisfaction and loyalty. Some of the possible future applications of machine learning in supply chain are:
- Personalized supply chain: Machine learning can help to create a personalized supply chain, that can tailor the products and services to the individual needs and preferences of the customers, by using data such as customer profiles, behavior, and feedback. Machine learning can also help analyze the supply chain, which can adapt and respond to the individual situations and conditions of the supply chain, by using data such as demand, supply, and risk. For example, Netflix uses machine learning to create a personalized supply chain, that can recommend the best movies and shows to its customers, by using data such as customer ratings, preferences, and history.
- Autonomous supply chain: Machine learning can help to create an autonomous supply chain, that can operate and optimize itself without human intervention, by using data such as sensors, cameras, and GPS. Machine learning can also help to create an autonomous supply chain, that can learn and improve itself continuously, by using data such as feedback, outcomes, and rewards. For example, Tesla uses machine learning to create an autonomous supply chain, that can produce and deliver its electric cars, by using data such as robots, drones, and self-driving vehicles.
- Sustainable supply chain: Machine learning can help to create a sustainable supply chain, that can reduce the environmental and social impact of the supply chain, by using data such as carbon footprint, energy consumption, and waste generation. Machine learning can also help to create a sustainable supply chain, that can enhance the economic and social value of the supply chain, by using data such as profitability, efficiency, and innovation. For example, Starbucks uses machine learning to create a sustainable supply chain that can source and produce its coffee using data such as climate, soil, and farmers.
These are some of the potential future applications of machine learning in supply chain, but they are not definitive. Machine learning can also enable other future applications and solutions for the supply chain, that can address the emerging and evolving needs and challenges of the supply chain, such as digitalization, globalization, and customization.
However, each future application and solution has its impact and implications for the supply chain stakeholders and customers and requires different levels of data, technology, and expertise. Therefore, it is important to consider the opportunities and risks of machine learning in supply chain, such as:
Opportunities | Risks |
Increase the supply chain efficiency and effectiveness | Increase the supply chain complexity and uncertainty |
Enhance the supply chain innovation and differentiation | Reduce the supply chain transparency and accountability |
Improve the supply chain resilience and agility | Expose the supply chain to cyberattacks and data breaches |
Boost the supply chain competitiveness and profitability | Disrupt the supply chain workforce and skills |
Elevate the supply chain customer satisfaction and loyalty | Challenge the supply chain ethics and regulations |
How to Implement Machine Learning in Supply Chain?
Machine learning in supply chain is not only a trend and an opportunity, but also a challenge and a responsibility. Machine learning in the supply chain requires a lot of data, technology, and expertise, as well as a clear vision, strategy, and execution. Therefore, it is not easy to implement machine learning in supply chain, and it requires a lot of planning, preparation, and practice.
Here is a step-by-step guide on how to implement machine learning in supply chain:
Step 1
- Examine the supply chain’s current state and readiness.
- Examine the supply chain’s current performance and capabilities.
- Determine the supply chain’s pain points and opportunities for improvement.
- Assess the supply chain’s data quality, availability, and integration.
- Examine the supply chain’s technological infrastructure, tools, and platforms.
- Examine the supply chain’s skills, competencies, and culture.
Step 2
- Define the vision and strategy for supply chain machine learning.
- Define the supply chain’s business problem and goal for machine learning.
- Align the vision and strategy of machine learning with the vision and strategy of the supply chain.
- Prioritize the supply chain machine learning use cases and applications.
- Calculate the value of machine learning and its impact on the supply chain.
- Create a roadmap and plan for implementing machine learning in the supply chain.
Step 3
- Implement the supply chain machine learning project and solution.
- In the supply chain, collect and prepare data for machine learning.
- Select and implement the supply chain machine learning technique and algorithm.
- Train and test the supply chain machine learning model and solution.
- Deploy and monitor the machine learning solution and supply chain performance.
- Update and improve the machine learning solution and supply chain performance.
Step 4
- Scale and sustain the adoption and transformation of machine learning in the supply chain
- Communicate and demonstrate the importance of machine learning in the supply chain.
- Use machine learning to engage and empower supply chain stakeholders and customers.
- Incorporate and standardize machine learning processes and practices throughout the supply chain.
- Invest in and innovate with machine learning technology and supply chain talent.
- Govern and manage the supply chain’s machine learning ethics and risks.
These are the general steps for implementing machine learning in supply chain, but they are not rigid or fixed. Each supply chain is different and unique, and each machine learning project and solution is different and unique. Therefore, it is important to adapt and customize the steps according to the specific needs and context of the supply chain and the machine learning project and solution.
Conclusion
Machine learning is a powerful and promising technology that can help supply chain management in various ways. Machine learning in supply chain can help improve the supply chain performance and efficiency, enhance the supply chain innovation and differentiation, and elevate the supply chain customer satisfaction and loyalty.
However, machine learning is also a complex and challenging technology that requires a lot of data, technology, and expertise, as well as a clear vision, strategy, and execution. Therefore, it is important to understand the concept, trends, and applications of machine learning in the supply chain, as well as the best practices and challenges for its implementation.
We hope you enjoyed reading this blog post and learned something new and useful about machine learning in supply chain. If you have any questions, comments, or feedback, please feel free to share them with us. We would love to hear from you and learn from you. Thank you for your time and attention.