Table of Contents
The pursuit of artificial intelligence (AI) has led to groundbreaking advancements, but it has also brought to light a critical challenge: the immense computational resources required for AI training. Training state-of-the-art AI models is an energy-intensive process that places a significant burden on the environment and economy. As AI models continue to grow in complexity, the demand for computational power is escalating rapidly, raising concerns about sustainability and accessibility.
A Promising Solution for AI Training Efficiency
In a bid to address these challenges, Google DeepMind has unveiled a groundbreaking new technique called Joint Example Selection (JEST). JEST represents a significant leap forward in AI training efficiency, promising to dramatically accelerate training speeds while drastically reducing energy consumption. This innovation has the potential to reshape the AI landscape and mitigate the environmental impact of AI development.
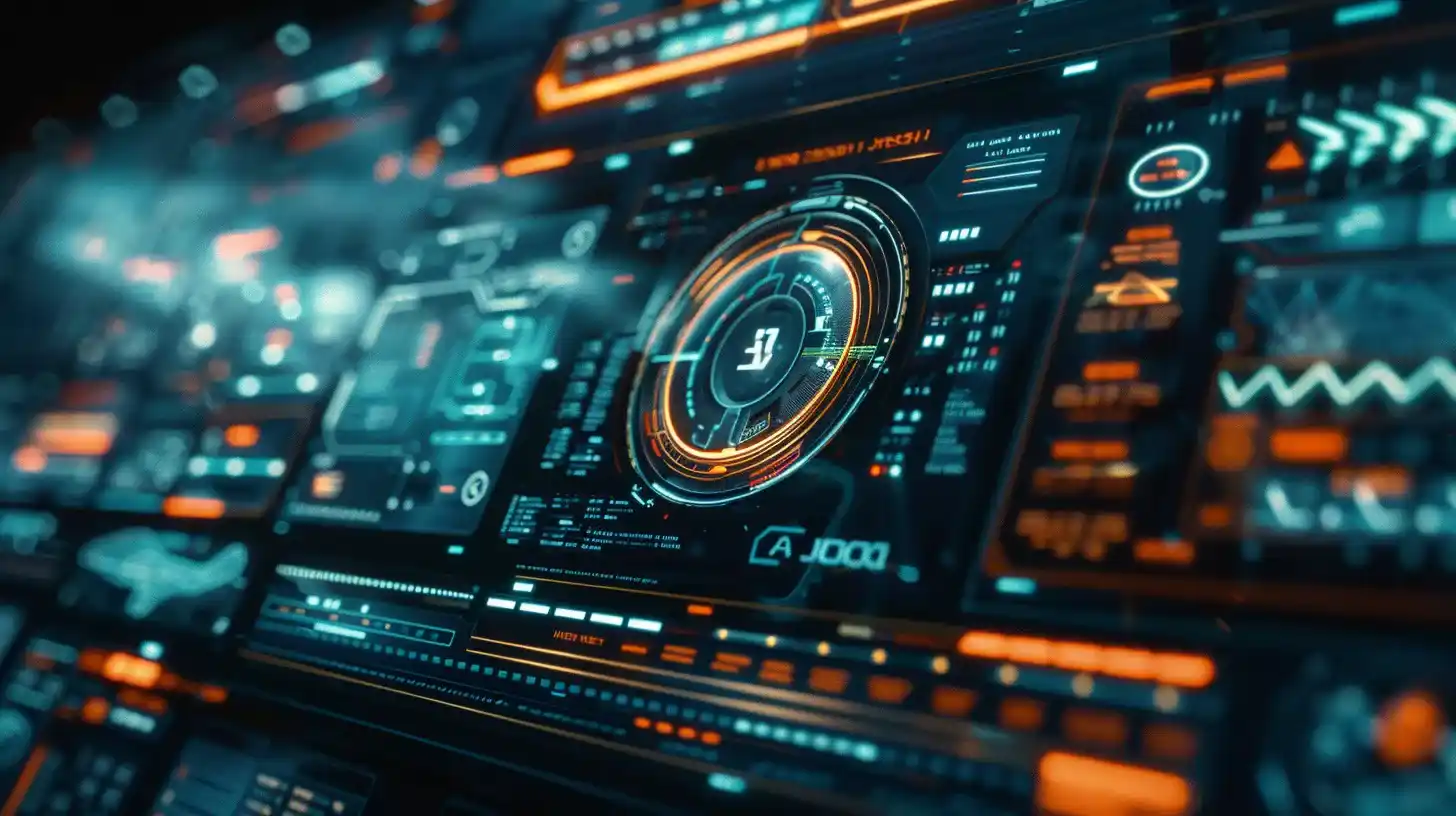
How JEST Works
JEST diverges from traditional AI training methods by focusing on entire batches of data rather than individual data points. This approach enables more efficient utilization of computational resources and accelerates the training process. JEST employs a two-tiered strategy:
- Data Quality Assessment: A smaller AI model is responsible for evaluating the quality of different data batches. This model acts as a discerning curator, ranking batches based on their potential contribution to the training process.
- Efficient Training: The highest-quality batches, as identified by the smaller model, are then fed into a larger model for training. By concentrating on the most valuable data, JEST maximizes training efficiency and minimizes computational waste.
The Importance of High-Quality Data for AI Training Efficiency
DeepMind emphasizes the crucial role of high-quality training data in the success of the JEST method. By carefully selecting and prioritizing data, JEST can significantly reduce the number of training iterations required, leading to substantial time and energy savings. The researchers claim that JEST surpasses existing state-of-the-art models by up to 13 times in terms of training iterations and 10 times in computational efficiency.
The Environmental and Economic Impact of AI Training Efficiency
The environmental impact of AI training has become a pressing concern. Data centers powering AI workloads consumed a staggering 4.3 gigawatts of electricity in 2023, equivalent to the annual energy consumption of a small country. This figure is projected to skyrocket as AI models become more complex.
Moreover, the economic costs of AI training are staggering. Training large-scale AI models can cost hundreds of millions of dollars, making AI development prohibitively expensive for many organizations.
JEST’s Potential to Transform AI Training Efficiency
JEST offers a promising solution to both the environmental and economic challenges of AI training. By drastically reducing computational requirements, it could pave the way for more sustainable and affordable AI development. However, the extent to which it will be adopted by industry giants remains to be seen.
Economic incentives will undoubtedly play a role in the adoption of JEST. While there is a strong motivation to reduce costs and minimize environmental impact, the relentless pursuit of AI supremacy may drive companies to prioritize speed and performance over efficiency.
The Future of AI Training Efficiency
DeepMind’s JEST represents a significant step forward in AI training efficiency, but it is just one piece of the puzzle. As the AI landscape continues to evolve, the development and adoption of innovative training techniques will be crucial in shaping the future of this transformative technology.
The future of AI training efficiency will be determined by a delicate balance between cost, speed, and sustainability. Striking the right balance will be essential for unlocking the full potential of AI while minimizing its environmental and economic impact.