Table of Contents
AMIE (Articulate Medical Intelligence Explorer) is a significant development in the domain of artificial intelligence (AI) and healthcare. Developed by Google Research and Google DeepMind, It represents an advanced research AI system designed for diagnostic medical reasoning and conversations. This system is based on Large Language Models (LLMs) and has been trained on real-world datasets that include medical reasoning, medical summarization, and clinical conversations.
To address the challenges of limited real-world data and the complexities of medical dialogues, It employs a novel self-play-based simulated learning environment. This environment facilitates the scaling of AMIE’s knowledge across a wide range of medical conditions and contexts. It involves two self-play loops for continuous learning and improvement.
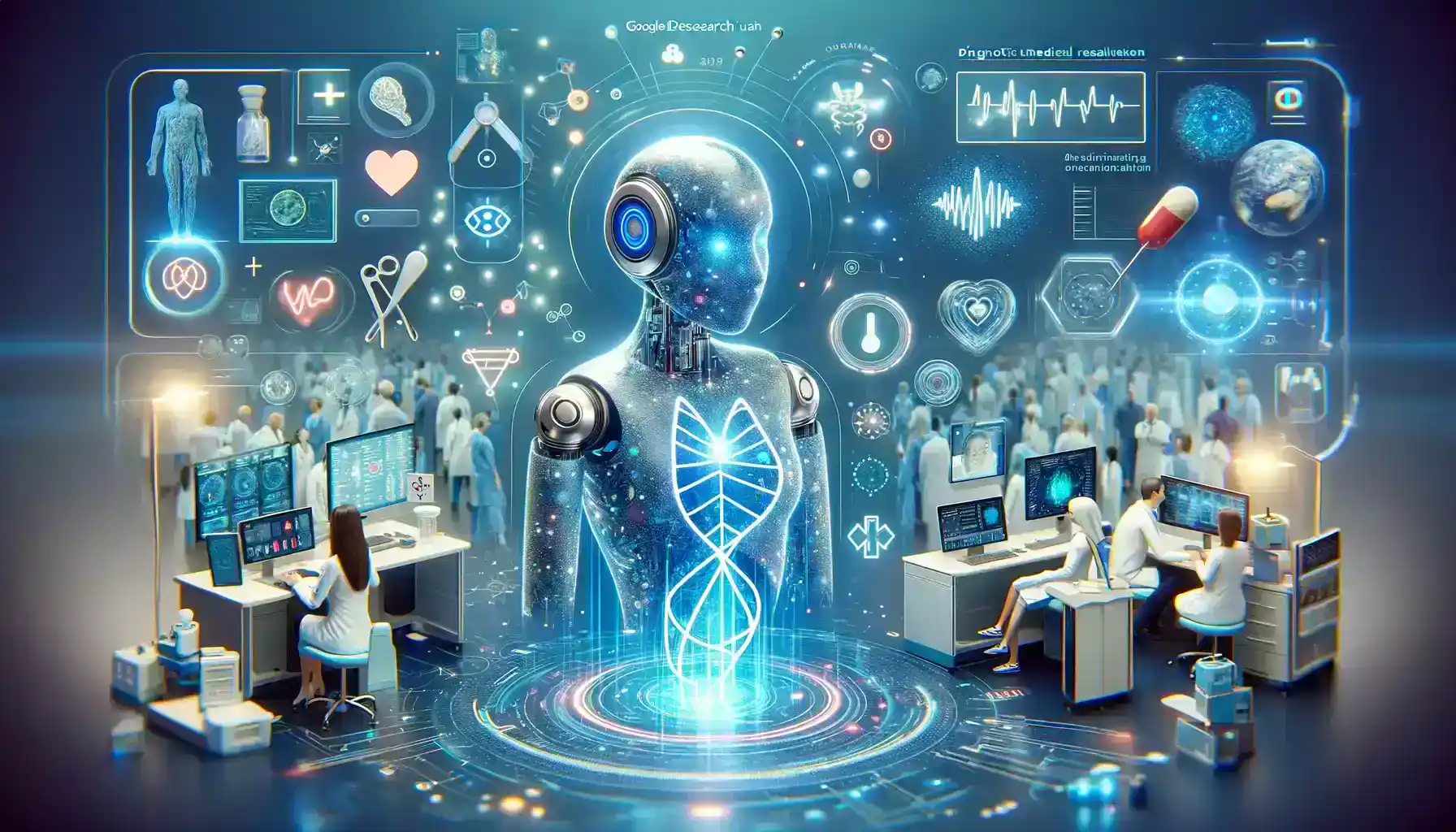
The first, an “inner” self-play loop, enables AMIE to refine its behavior in simulated conversations with an AI patient simulator. The second, an “outer” self-play loop, incorporates the refined simulated dialogues into further fine-tuning iterations. This process helps it to progressively refine its responses in diagnostic conversations, ensuring more informed and grounded replies.
In a randomized, double-blind crossover study, AMIE demonstrated its effectiveness in simulated diagnostic consultations via a text interface, achieving higher diagnostic accuracy and better performance in many clinically important aspects of consultation quality compared to human doctors. The results of this study, which included simulated patients played by trained actors, were evaluated by both medical specialists and patient actors. Despite these promising results, the study also acknowledges that real conversations often occur face-to-face, and the text-based interface used in the study may underestimate the value of human conversations. Additionally, the study simulated rarer illnesses, and extensive further research is needed to transform AMIE from a research prototype into a robust clinical tool.
The development of AMIE addresses a gap in medical interviews, where some doctors may fall short in their bedside manner. Leveraging the empathetic and helpful traits often exhibited by LLMs like ChatGPT, and AMIE aims to improve the quality of medical interviews. This approach not only enhances diagnostic accuracy but also focuses on improving the system’s bedside manner. The system has been tested for its diagnostic accuracy and patient interaction quality with trained actors simulating patients.
Overall, while it shows promise in enhancing the quality and accessibility of healthcare, it is essential to note that it remains a research prototype. Transitioning it into a safe and robust tool for real-world use will require addressing limitations and conducting further research in areas such as health equity, fairness, privacy, robustness, and performance under real-world constraints.
Assessing the Efficacy of AI in Diagnostic Conversations
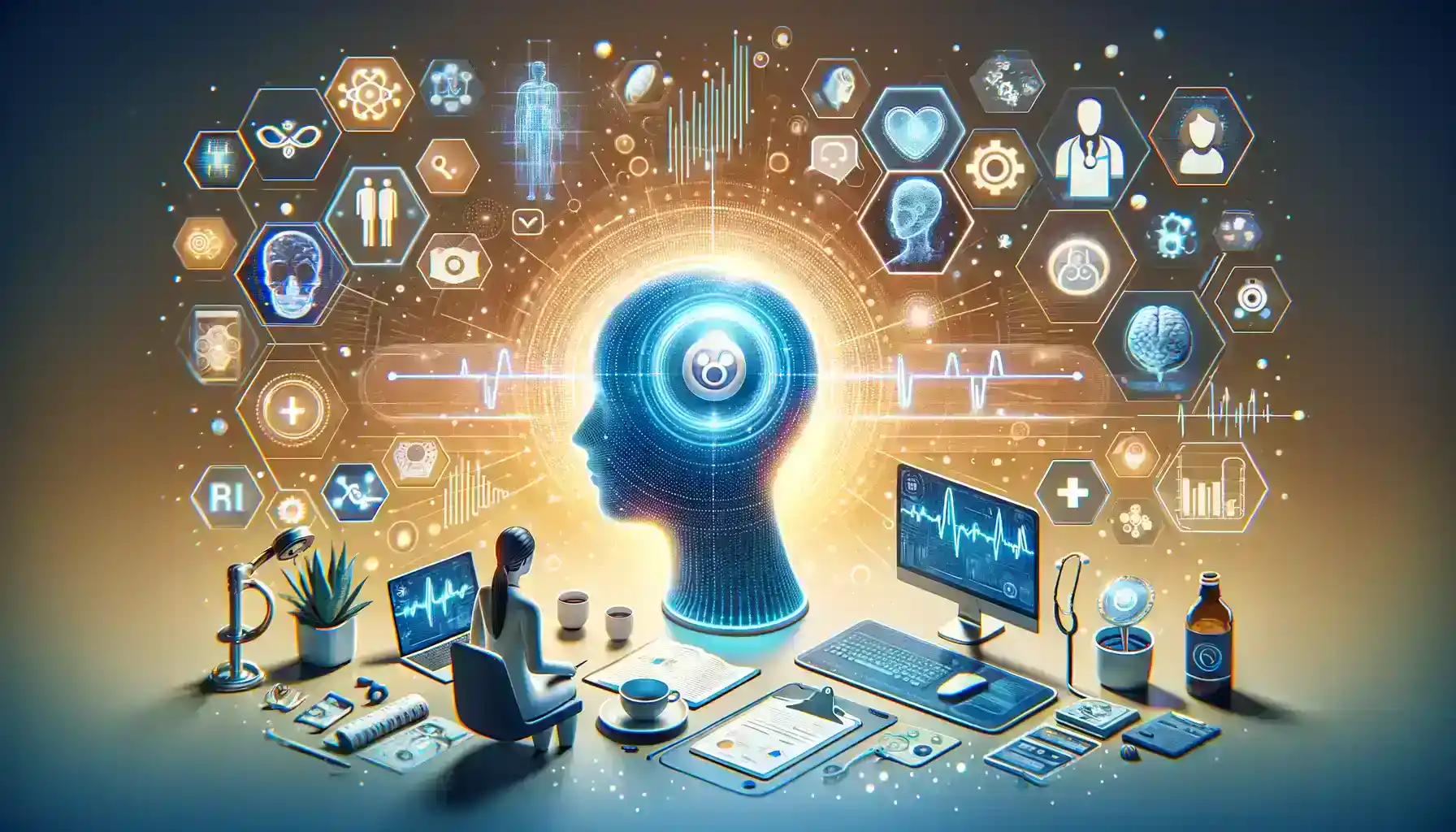
AMIE stands out in its approach to conversational diagnostic AI. It is trained on extensive real-world datasets encompassing medical reasoning, summarization, and clinical conversations. This training allows it to engage in nuanced and complex medical dialogues, replicating the intricacies of human medical consultations. What sets it apart is its ability to adapt and learn through a self-play simulated learning environment, making it proficient in handling a vast array of medical conditions and contexts.
To evaluate AI systems for diagnostic conversations, we developed a pilot evaluation rubric based on real-world consultation quality and clinical communication metrics. This rubric measures various aspects, including history-taking, diagnostic accuracy, clinical management, communication skills, relationship-building, and empathy.
We conducted a randomized, double-blind crossover study using text-based consultations. The participants included validated patient actors and board-certified primary care physicians (PCPs), comparing their performance with the AI system. The setup resembled an Objective Structured Clinical Examination (OSCE), a standard method to assess clinicians’ skills in realistic scenarios. In these simulations, clinicians interact with trained actors representing patients with specific conditions. The consultations were conducted via a synchronous text-chat interface, similar to those used in common large language model (LLM) applications.
AMIE Revolutionizing Healthcare with LLM-Based Diagnostic AI
At its core, AMIE is based on Large Language Models (LLMs), enabling it to process and understand medical dialogue at an unprecedented level. This system undergoes continuous refinement through a dual self-play loop. The inner loop focuses on simulated conversations with an AI patient simulator, while the outer loop integrates these dialogues into subsequent iterations for further enhancement. This unique method ensures that AMIE’s responses are not only accurate but also contextually relevant and informed.
Training LLMs for medical conversations using real-world dialogues poses two major challenges: limited scope of medical conditions and scenarios, and data noise including ambiguous language and ungrammatical utterances. To overcome these, we developed a self-play simulated learning environment for AMIE, enhancing its diagnostic dialogue capabilities in a virtual care setting. This approach includes a dual self-play loop system.
The first, an inner loop, uses in-context feedback for AMIE to refine its interactions with an AI patient simulator. The second, an outer loop, integrates these refined dialogues into further training. This iterative process fosters continuous learning and improvement. Additionally, AMIE employs a chain-of-reasoning strategy, allowing it to adapt its responses based on the ongoing conversation for more accurate and relevant replies.
AMIE Performance in AI-Powered Medical Diagnostics
AMIE’s performance has been rigorously evaluated in a study involving simulated patients, played by trained actors, and compared against board-certified primary care physicians (PCPs). The results were remarkable – AMIE demonstrated at least equal, if not superior, diagnostic accuracy and consultation quality compared to the PCPs. This was assessed along multiple clinically meaningful axes, including history-taking, clinical management, and empathy.
In comparative evaluations, AMIE matched or surpassed primary care physicians (PCPs) in simulated diagnostic conversations. It excelled in 28 out of 32 criteria assessed by specialist physicians and in 24 out of 26 criteria according to patient actors, demonstrating higher diagnostic accuracy and overall superior performance in most of the evaluated areas of consultation quality.
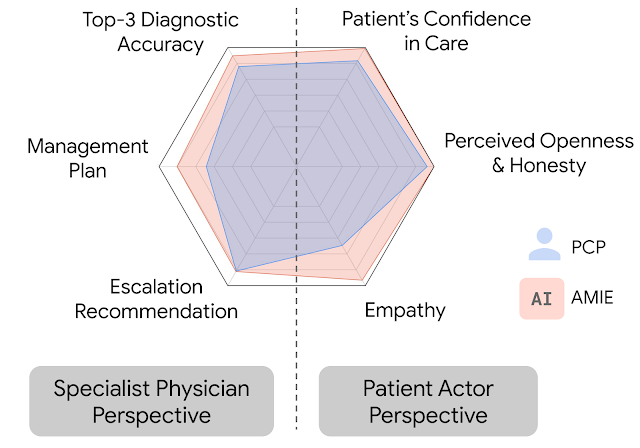
Challenges of AMIE
Despite its groundbreaking advancements, AMIE is not without its limitations. The research acknowledges that the real-world value of human conversations might be underestimated due to the study’s reliance on a text-based interface. Moreover, the prototype nature of AMIE means that further research and development are needed to address concerns like health equity, privacy, robustness, and performance in real-life conditions.
Our study has limitations that need careful consideration. First, the evaluation, conducted via a text-chat interface unfamiliar to clinicians, may not fully capture the essence of real-world human conversations and clinical practice. Second, this research should be viewed as an initial step in a much larger journey.
Data Privacy and Security: Handling sensitive medical data requires stringent security measures and adherence to privacy laws, posing a significant challenge.
Ethical Considerations: The use of AI in healthcare raises ethical questions, particularly around decision-making in critical care situations.
Regulatory Compliance: Navigating the complex landscape of healthcare regulations and gaining approval from relevant authorities will be crucial for widespread adoption.
Overcoming Limitations in Training Data: As AMIE relies on available data, ensuring comprehensive and unbiased data sets is necessary to avoid skewed diagnostics.
Clinician Acceptance and Integration: Gaining trust and acceptance from healthcare professionals and effectively integrating AMIE into existing medical workflows remains a challenge.
Handling Complex Cases: While AMIE shows promise, its ability to handle highly complex or rare medical cases compared to experienced physicians is yet to be fully understood.
Future Prospects
Looking ahead, AMIE’s potential in transforming healthcare is immense. As it evolves, it is expected to become an invaluable tool not only in diagnostic accuracy but also in enhancing the patient-doctor relationship. The focus will be on transitioning AMIE from a research prototype to a robust, real-world application, addressing its current limitations, and exploring its applications in various medical settings.
Integration into Clinical Practice: AMIE could become an integral tool in clinical settings, assisting healthcare professionals in making faster, more accurate diagnoses.
Enhanced Patient Care: By reducing diagnostic time and increasing accuracy, AMIE has the potential to improve patient outcomes and overall healthcare efficiency.
Continuous Learning and Adaptation: With its AI foundation, AMIE can continuously learn from new medical data, staying updated with the latest medical trends and treatments.
Global Healthcare Access: AMIE could significantly aid in regions with limited access to healthcare professionals, providing expert-level diagnostic support remotely.
Personalized Medicine: Leveraging AI, AMIE might evolve to offer more personalized healthcare recommendations based on individual patient histories and genetic information.
Conclusion
AMIE’s unveiling in 2024 marks a milestone in medical technology. Its innovations in conversational diagnostic AI, learning methodologies, and performance are significant strides toward a future where AI and healthcare are seamlessly integrated. While there are challenges to overcome, the future outlook for AMIE is promising, potentially leading to more accessible, accurate, and empathetic healthcare services. As the technology matures, it holds the promise of revolutionizing medical diagnostics and patient care on a global scale.
In summary, AMIE stands at the forefront of a healthcare revolution, offering enhanced diagnostic capabilities through AI. However, realizing its full potential requires navigating through a myriad of challenges, including ethical, regulatory, and technological hurdles. With careful management and ongoing development, It has the potential to significantly augment healthcare delivery globally.