Table of Contents
Google DeepMind, the company behind the groundbreaking AlphaFold, has developed a new artificial intelligence tool that can predict the structures and stability of millions of new materials. The tool, called Graph Networks for Materials Exploration (GNoME), could have wide-reaching benefits in sectors such as renewable energy and computing.
How GNoME works and what it can do
GNoME is based on graph neural networks, a type of machine learning model that can learn from complex data such as graphs, networks, and molecules. GNoME was trained on data from the Materials Project, a multinational research effort that has computationally predicted the structures and stability of 28,000 materials.
Using GNoME, Google DeepMind was able to generate more than 2.2 million new crystal structures that are likely to be stable. Among them, 381,000 were selected as the most promising candidates and published in the Inorganic Crystal Structures Database (ICSD), the world’s largest database of identified materials. This increased the number of known stable materials by a factor of ten.
Stable materials are those that do not spontaneously decompose due to weak bonds between their atoms. They are essential for many applications, such as energy storage, solar cells, and superconductor chips. However, finding new stable materials is a challenging and time-consuming task, as only certain combinations of elements can form them.
“Humanity knows only about a few tens of thousands of stable materials, while materials play a very critical role in almost any technology,” said Ekin Dogus Cubuk, a staff research scientist at Google Brain who worked on GNoME. “For example, if you want to find a new solid electrolyte for better batteries, you have to apply many filters, such as ionic conductivity, electronic insulation, non-toxicity, and non-radioactivity. Once you do that, we only have a few options that we can go with, which end up not really revolutionizing our batteries.”
The impact of GNoME on materials science and technology
GNoME can help materials scientists discover new materials that meet their desired criteria by suggesting novel structures that are likely to be stable. To verify the predictions of GNoME, Google DeepMind collaborated with external researchers, who successfully synthesized 736 of the new materials in the lab.
Among the new materials predicted by GNoME, there were 528 potential lithium ion conductors that might be used in batteries and 52,000 new layered compounds with a similar structure to graphene, opening up the possibility that some of these could be the basis for new superconducting materials. “We believe that some of these will be made in the lab, which will hopefully lead to very exciting applications,” said Cubuk.
GNoME: A breakthrough for materials science
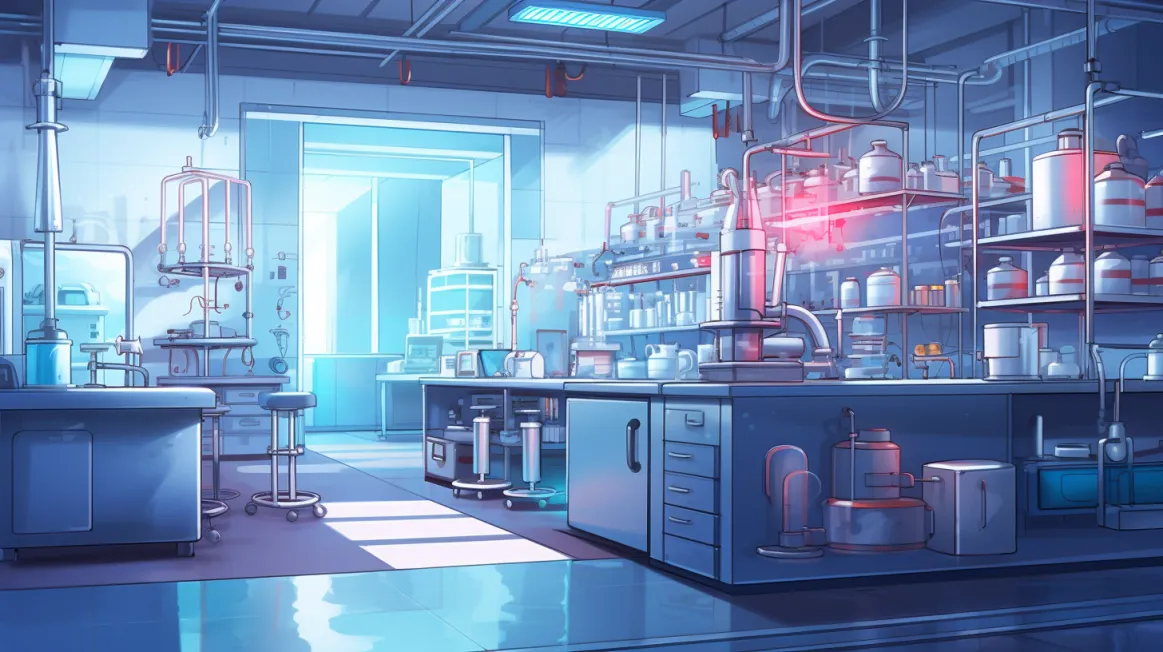
GNoME is not the first AI tool to predict new materials, but it is the most powerful and accurate one so far. It outperforms previous methods, such as the Materials Project, by orders of magnitude in terms of the number and diversity of materials it can generate.
GNoME also has the advantage of being able to predict not only the stability of materials but also some of their properties, such as ionic conductivity. This is important for applications such as batteries, where the performance of the material depends on how well it can transport ions.
The Google DeepMind researchers are optimistic that future AI tools will be able to predict other useful properties, such as electrical conductivity, thermal conductivity, and optical properties. “Machine learning models, when trained on a lot of data, really learn interesting aspects of quantum mechanics and are able to generalize and make predictions about things that they were never trained on,” Cubuk said. “Which makes us very excited about our next challenges, such as predicting synthesizability.”
Predicting new materials is only the first step towards creating them. The next step is to synthesize them in the lab, which can also be a lengthy and costly process. To speed up this step, researchers at the Lawrence Berkeley National Laboratory are working on an automated materials synthesis system, called the A-Lab, that can attempt to synthesize materials predicted by the Materials Project. The A-Lab worked 24 hours a day, 7 days a week, for 17 days to synthesize 58 materials, succeeding in 41 cases.
“This is the future—to design materials autonomously using computers, but also then to make them autonomously using these robotic labs and learn from the process,” said Kristin Persson, the founder of the Materials Project, at a briefing.
GNoME is the latest achievement from Google DeepMind, which has also produced the protein-folding-predicting AlphaFold, the genetic disease screening tool AlphaMissense, and the weather-forecasting GraphCast. These tools demonstrate the potential of AI to solve complex scientific problems and advance human knowledge.
“If you think about the protein structure prediction problem and if you think about material stability, both of them are root node problems, [that] we think unlock a number of different applications beyond those problems themselves,” said Pushmeet Kohli, who leads Google DeepMind’s AI for Science team. “This specific problem has implications for so many other problems that society really cares about today.”