Discover How Google and Anthropic Tackle Generative AI Systems’ Limitations
Table of Contents
Generative AI systems can create new content, such as text, images, or music, based on existing data. They have many applications, such as content creation, data augmentation, and natural language processing. However, they also have some limitations that need to be addressed, such as hallucinations, data provenance, and hardware requirements.
Hallucinations
Hallucinations are instances where generative AI systems produce incorrect or misleading statements with high confidence. This can pose a serious problem for customers who rely on accurate information, especially in regulated industries. For example, Lawrence Fitzpatrick, chief technology officer of OneMain Financial, expressed his concern about hallucinations at The Wall Street Journal CIO Network Summit on Monday (Feb. 12).
To address this issue, Google and Anthropic, two leading companies in the field of generative AI, are working on different solutions. Google’s vice president of product management at DeepMind, Eli Collins, suggested that AI systems should provide the sources of their information so that users can verify and trust their outputs.
Anthropic’s co-founder and chief science officer, Jared Kaplan, proposed that generative AI systems should respond with “I don’t know” when they lack sufficient data, and only provide answers with citations. Both solutions aim to increase the transparency and accuracy of generative AI systems.
However, finding the right balance between caution and usefulness is not easy. Kaplan admitted that AI systems that are too cautious may not be very helpful, as they may say “I don’t know” to everything. He said that the goal is to make generative AI systems that are both reliable and useful.
Data Provenance
Another challenge that generative AI systems face is the provenance of their training data. This refers to the origin and ownership of the data that is used to train the AI models. Recently, The New York Times filed a lawsuit against Microsoft and OpenAI, accusing them of using its content without permission to train their AI systems. Kaplan explained that it is hard to remove specific content from trained models, as they may have learned from many sources.
Hardware Requirements
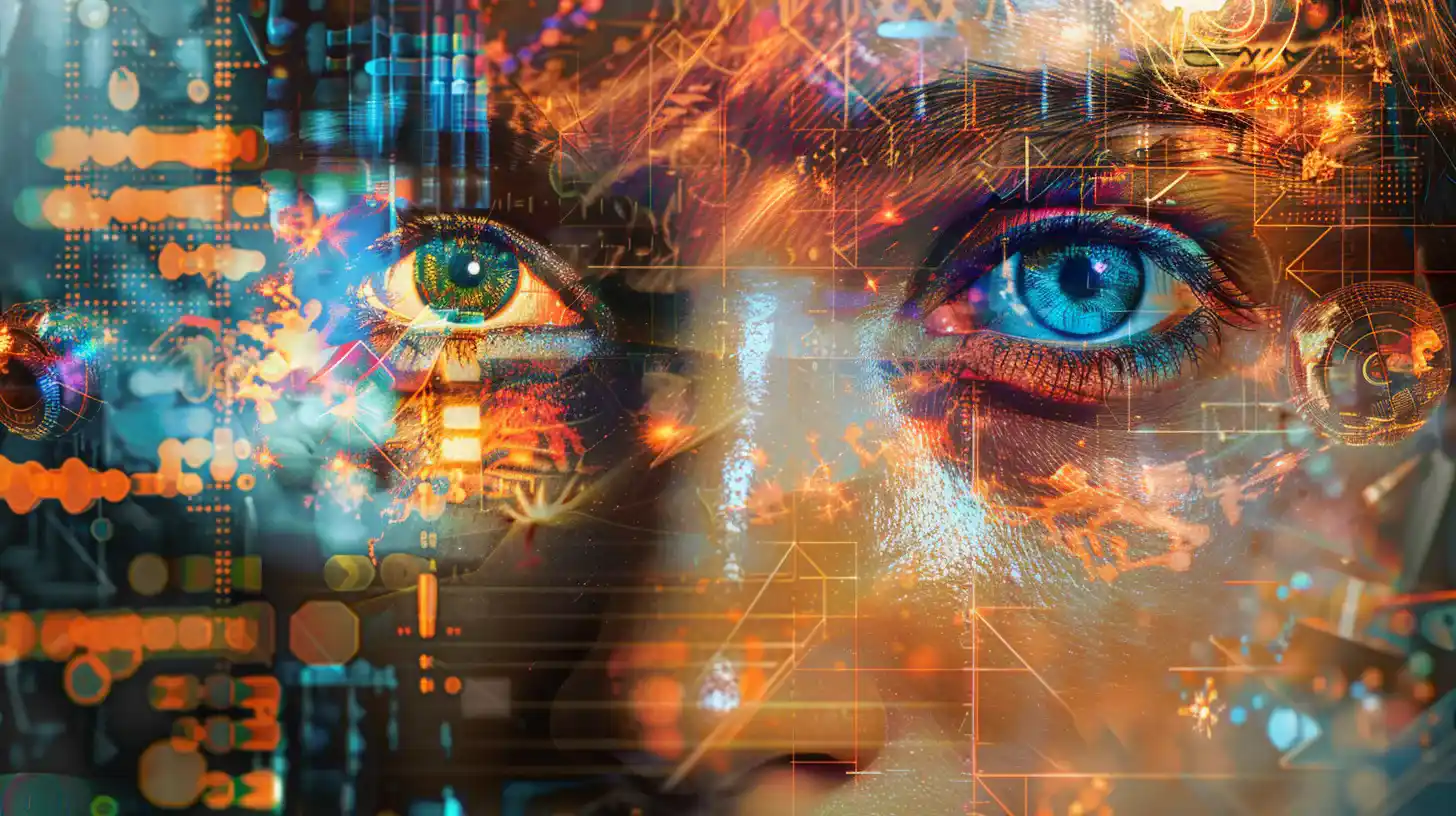
Finally, generative AI systems also depend on hardware, such as AI chips, to perform their tasks. These chips are specialized devices that can process large amounts of data faster and more efficiently than conventional chips. However, they are also expensive and scarce, limiting the accessibility and scalability of AI systems.
Google and Anthropic are aware of this challenge and are working to improve the availability, capacity, and cost-effectiveness of AI chips. Google has developed its own AI chips, called Tensor Processing Units (TPUs), to reduce costs and increase efficiency.
What are some examples of generative AI systems?
Generative AI systems are artificial intelligence systems that can create new content, such as text, images, video, or audio, based on existing data. Some examples of generative AI systems are:
- ChatGPT: A chatbot that can generate realistic and engaging conversations in natural language, based on a large corpus of web text.
- Bard: A tool that can generate original lyrics for songs in different genres and styles, based on a prompt from the user.
- DALL-E: A system that can generate images from text descriptions, using a combination of natural language and vision models.
- Midjourney: A platform that can generate realistic 3D models of human faces, bodies, and clothing, based on a few input parameters.
- DeepMind: A research company that develops generative AI systems for various domains, such as gaming, healthcare, and science. Some of its projects include AlphaGo, WaveNet, and AlphaFold.
These are just a few examples of the many generative AI systems that exist or are being developed.
Conclusion
Generative AI systems have great potential but also face some challenges. Google and Anthropic are working to overcome them and build trust with customers. This news coincided with the mixed reviews that Microsoft’s AI assistant, Copilot for Microsoft 365, received from business users. While some praised its usefulness, others criticized its price and limited functionality.
Featured Insights
Integrating Real-Time Weather Data into Your Travel and Navigation App
The Future is Now: AI and ML Technologies Reshaping Healthcare
Introduction to Digital Twin Technology in Manufacturing with Unprecedented Efficiency and Innovation
Adobe’s Firefly AI: New Tools for Photoshop and Illustrator
Introducing Meta Imagine Me: Your Digital Doppelganger
AI in Gaming is Transforming the Industry