Table of Contents
Unveiling the Future: Kinetica’s Generative AI
In the vanguard of real-time analytics, Kinetica heralds a transformative generative AI solution, unveiled at the NVIDIA GTC. This avant-garde technology heralds a new era in retrieval-augmented generation (RAG), a paradigm shift for enterprise clientele.
RAG, the backbone of generative AI applications, now taps into contemporary knowledge bases, transcending the confines of a large language model’s (LLM) foundational training data. Traditional vector similarity searches, the go-to for contextual enrichment, falter with quantitative data, their design rooted in textual comprehension. Moreover, the latency from obligatory reindexing hampers the immediacy of data availability, thwarting real-time operational data interfacing.
The Core of Innovation: Kinetica’s Technological Synergy
Enter Kinetica’s brainchild — a synergy of NVIDIA NeMo’s prowess, part of the NVIDIA AI Enterprise suite, and the vigor of NVIDIA’s accelerated computing. At its core, two pivotal elements: the swift vector search, courtesy of NVIDIA RAPIDS RAFT, and the adeptness in executing intricate data queries instantaneously. This potent duo bestows enterprises with the understanding to instantly augment generative AI applications with analytical insights, freshly mined from operational data.
Kinetica transcends further. For AI to fathom data, it necessitates context — an understanding of the data’s structure, interrelations, and semantics. Kinetica’s ingenuity lies in its native database objects, empowering users to impart semantic context to enterprise data. These objects equip an LLM with the referential insight required for context-aware database interaction.
Real-Time RAG: A New Dawn for Data Interaction
Nima Negahban, Kinetica’s Cofounder and CEO, elucidates, “Our real-time RAG solution, fueled by NVIDIA NeMo Retriever microservices, melds LLMs with the pulse of real-time data streams, eclipsing traditional constraints.” He adds, “This breakthrough empowers our clients to glean business acumen from operational data, like telco network analytics, through the simplicity of English queries.”
Kinetica’s generative AI solution, accessible to developers via a relational SQL API and LangChain plugins, ensures that application builders can leverage the full spectrum of a relational database’s enterprise-grade capabilities. This encompasses data access governance (Role-Based Access Control), minimizing data transference from extant data repositories (via query federation), and the preservation of established relational schemas.
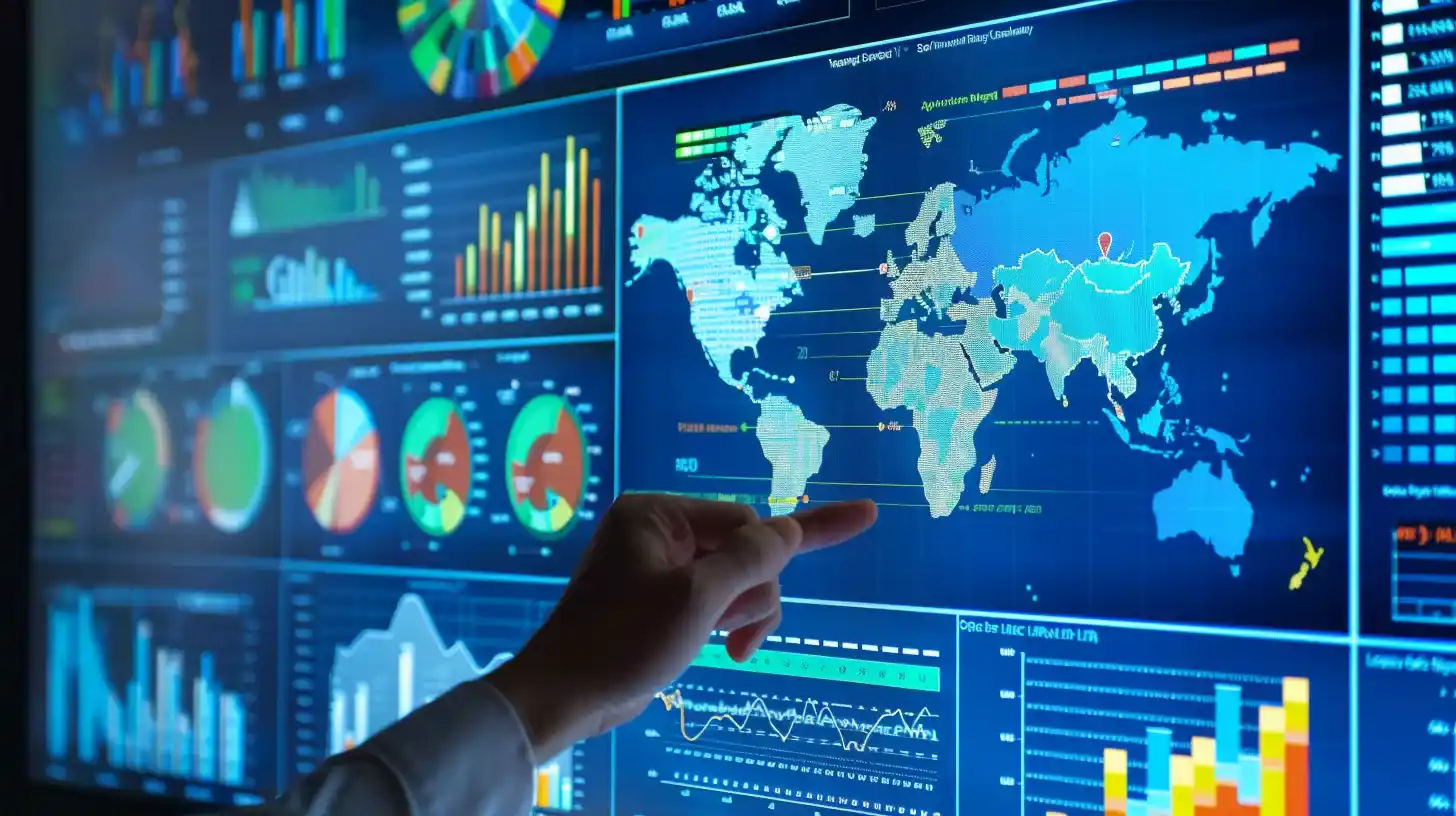
Ronnie Vasishta, NVIDIA’s Senior Vice President of Telecom, remarks, “Data is AI’s cornerstone, and the corporate world is keen to link it with generative AI applications.” He continues, “Kinetica, utilizing NVIDIA’s AI Enterprise platform and accelerated computing infrastructure, injects real-time data into LLMs, catalyzing customer productivity through generative AI.”
How It Works
Kinetica’s generative AI solution abolishes the reindexing prerequisite for vectors, readying them for immediate querying. It boasts an ingestion rate of vector embeddings quintuple that of its predecessors, as per the VectorDBBench benchmark. This amalgamation delivers unparalleled vector similarity search performance, catering to real-time applications.
Responsiveness is paramount for user-facing applications. Kinetica ensures that generative AI applications have the data runtime to maintain fluid conversations, free from the dreaded spinning wheel of delay.
Beneath the surface, Kinetica harnesses NVIDIA CUDA Toolkit to craft vectorized database kernels, exploiting NVIDIA GPUs’ parallelism. Its repository of analytical functions, fully vectorized, spans essential operations like filtering, joining, and aggregating, alongside specialized functions for spatial, time-series, and graph analytics.
Use Cases
Kinetica’s analytical prowess extends across various domains, proving invaluable for domain-specific generative AI applications. In telecommunications, for instance, Kinetica’s solution enables real-time analysis of pcap traces, leveraging complex spatial joins, aggregations, and time-series operations.
Traditionally, network engineers rely on tools like Wireshark, which demand protocol expertise. Kinetica’s real-time RAG solution simplifies this, allowing engineers to query network traffic using generative AI in plain English.
Another application involves two data streams: L2/L3 radio telemetry and a vector table of telecom-specific rules and definitions. A telco-specific LLM, trained on telecom data and schema and integrated with NVIDIA NeMo, powers a chatbot application. This LLM translates user inquiries into real-time queries, with NeMo rendering the results into comprehensible responses.