Table of Contents
Generative Artificial Intelligence (GenAI) is a rapidly evolving field with immense potential. It involves the creation of AI systems that can generate data, images, text, or even entire simulations autonomously. This transformative technology is reshaping industries across the globe and has applications in areas such as healthcare, finance, entertainment, and more.
The “Generative AI Learning Path” refers to the journey individuals undertake to master this complex and exciting field. This path is open to individuals with varying levels of expertise, from complete beginners to seasoned professionals.
Generative AI Learning Path
The concept of a “Generative AI Learning Path: From Basics to Brilliance” outlines a structured approach for individuals interested in mastering Generative Artificial Intelligence (AI). It acknowledges that becoming proficient in this field involves a gradual progression from foundational knowledge to advanced expertise, ultimately reaching a level of brilliance.
The journey begins with a strong emphasis on understanding the fundamental concepts of Generative AI. This includes grasping the core principles, algorithms, and terminologies. Learners should gain proficiency in concepts like neural networks, deep learning, and generative models, which serve as the bedrock of the Generative AI learning path.
To move from basics to brilliance, learners must explore advanced topics within the Generative AI learning path. This might involve investigating state-of-the-art research papers, experimenting with cutting-edge techniques, and staying updated with the latest advancements in the field.
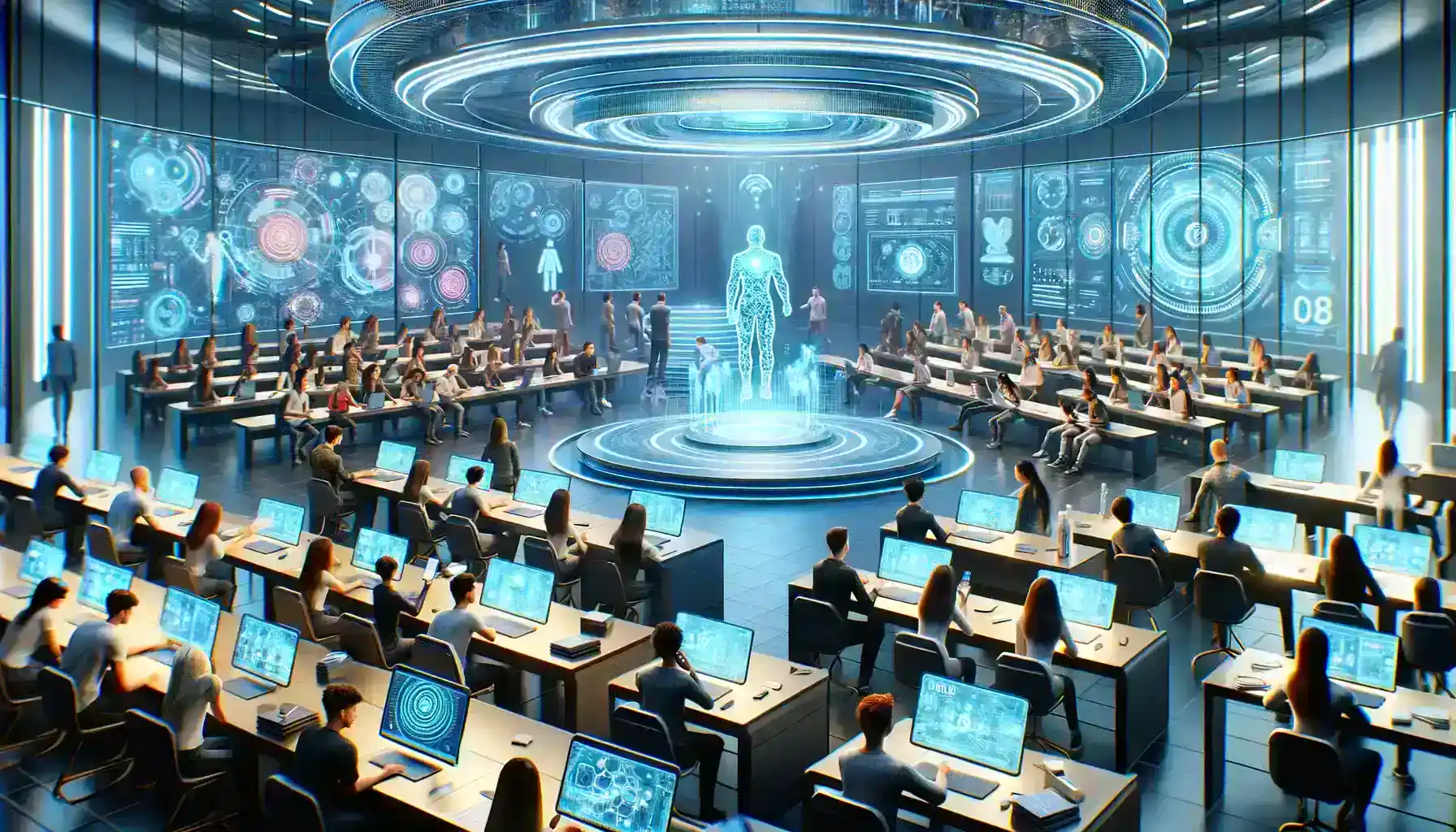
1. Choosing the Right Learning Resources
When embarking on your Generative AI learning path, selecting the right learning resources is one of the most critical decisions you’ll make. This choice can significantly impact the effectiveness and efficiency of your journey from basics to brilliance in the Generative AI learning path.
- Reputable Online Courses: Reputable online courses are a cornerstone of an effective Generative AI learning path. Platforms like Coursera, edX, and fast.ai offer a wide range of comprehensive courses, often taught by world-renowned experts. These courses cover topics from the fundamentals of machine learning and neural networks to advanced Generative AI techniques.
- Tutorials and Online Documentation: In addition to formal courses, you can benefit from tutorials and online documentation provided by libraries and frameworks like TensorFlow, PyTorch, and Keras.
- Research Papers: While research papers may be more advanced, they are essential for staying updated with the latest breakthroughs in the Generative AI learning path. Platforms like arXiv and Google Scholar are valuable resources for accessing research papers.
2. Hands-on Experience and Projects
Theory is undoubtedly crucial when understanding the concepts and principles of Generative AI, but real mastery comes from practical application. This section will delve deeper into the significance of hands-on experience and projects in your journey along the Generative AI learning path. Theoretical knowledge provides you with a framework, but it’s the practical application that cements your understanding and expertise.
- Concrete Understanding: When you work on practical projects, you get a concrete understanding of how generative models function. You can witness firsthand how data is transformed into meaningful outputs.
- Problem Solving: Real-world projects often come with challenges and roadblocks. By working through these issues, you develop problem-solving skills that are invaluable in the field of Generative AI.
- Creativity: Generative AI is not just about replicating existing patterns but also about creating something new and innovative. Hands-on projects allow you to unleash your creativity and experiment with different ideas
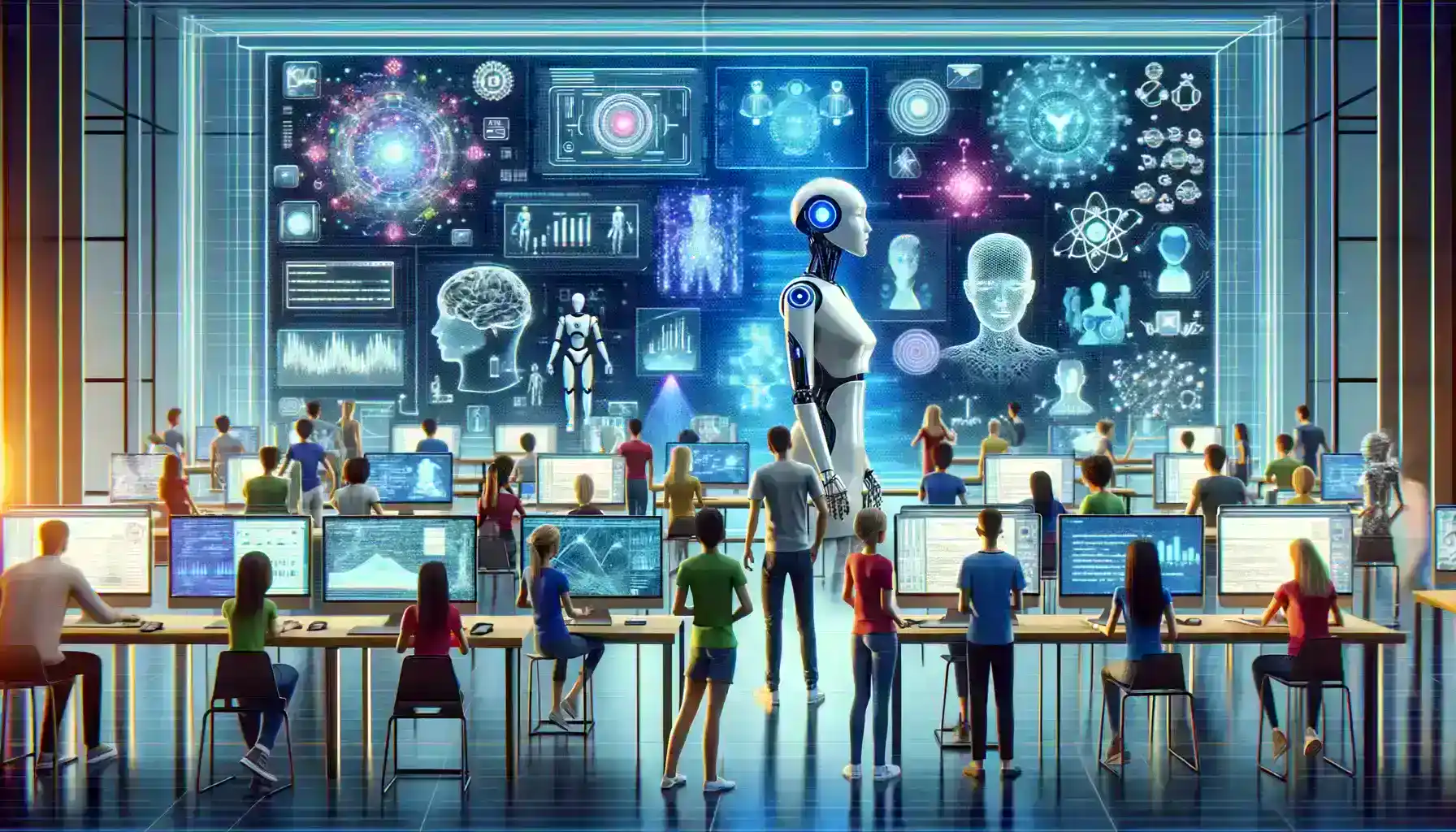
Continuous Learning and Iteration
It’s important to note that hands-on experience is not a one-time endeavor. Generative AI is a rapidly evolving field, and technologies advance quickly. As you work on projects, stay updated with the latest developments, and be open to iterating on your work. Continuous learning and refinement of your projects will help you stay at the cutting edge of Generative AI.
3. Stay Updated and Experiment
Generative AI, with its dynamic nature and constant advancements, demands a commitment to staying informed and engaged with the latest developments. In the ever-evolving landscape of Generative AI, the key to excellence lies in both keeping yourself updated and actively experimenting.
- Continuous Learning: To embark on the journey towards expertise in the Generative AI learning path, you must make learning a lifelong endeavor. This begins with staying updated on the newest research papers, publications, and breakthroughs in the field. Follow leading academic journals, online forums, and reputable sources such as arXiv, Google AI, and OpenAI to stay informed about cutting-edge research.
- Attend Conferences and Workshops: Generative AI conferences and workshops, such as NeurIPS, ICLR, and CVPR, serve as invaluable platforms for acquiring knowledge and networking with experts. Participating in these events allows you to gain insights from thought leaders, witness live demonstrations of innovative technologies, and engage in meaningful discussions that can inspire your work.
- Experimentation: Knowledge without practical application can only take you so far. Experimentation is a crucial component of mastering Generative AI. It involves hands-on work with different architectures, models, and techniques. By experimenting, you not only deepen your understanding but also gain valuable insights into the strengths and weaknesses of various approaches.
- Pushing Boundaries: Generative AI thrives on innovation and pushing boundaries. Don’t limit yourself to the status quo; challenge conventions and explore uncharted territories. Pioneering work often emerges from those who are willing to take calculated risks and explore unconventional ideas.
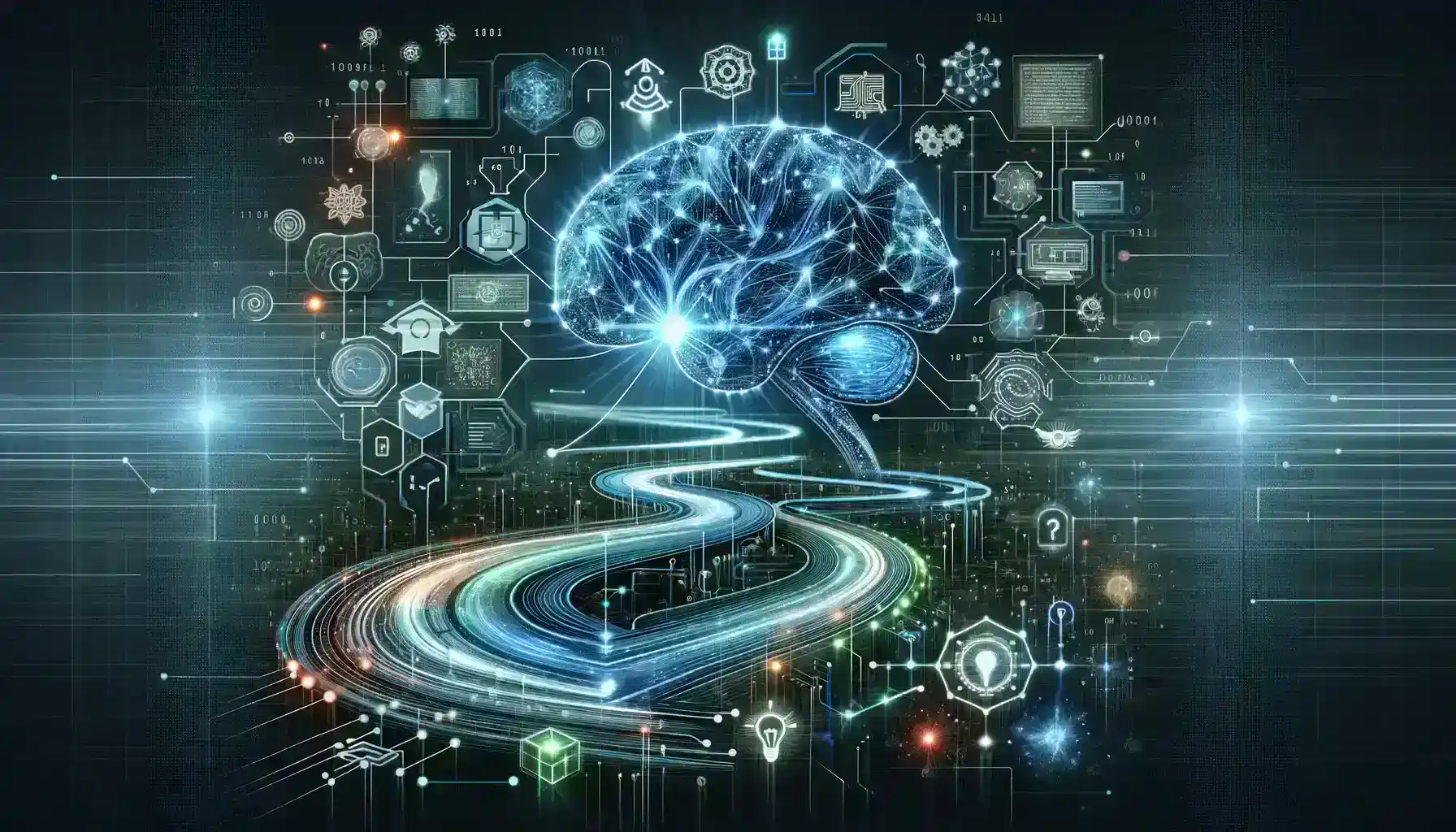
4. Collaborate and Seek Mentorship
In the dynamic world of Generative AI, collaboration and mentorship play pivotal roles in accelerating your learning journey. As you embark on your path toward mastering Generative AI, understanding the importance of these aspects can make a significant difference in your growth and development.
Networking and Collaboration: Networking and collaboration are essential components of the Generative AI learning path. Engaging with others who share your passion for Generative AI can provide several benefits.
- Knowledge Sharing: By joining online forums, communities, and social media groups dedicated to Generative AI, you gain access to a wealth of knowledge and expertise. These platforms are often hubs where enthusiasts, researchers, and practitioners share their insights, research findings, and practical experiences.
- Problem Solving: In the world of AI, it’s common to encounter challenges and roadblocks. Collaborating with others allows you to tap into collective wisdom when you face complex issues. You can seek advice, discuss potential solutions, and learn from the experiences of those who have overcome similar obstacles. Moreover, you can use ERG software, which enables seamless collaboration across different teams and fosters inclusive work environments.
- Staying Updated: The field of Generative AI evolves rapidly. Being part of a network ensures that you stay up-to-date with the latest developments, research papers, and breakthroughs. This knowledge is vital for maintaining your expertise and relevance in the field.
Seeking Mentorship: In addition to networking and collaborating with peers, seeking mentorship from experienced professionals is a valuable strategy for advancing your Generative AI learning path.
- Guidance and Direction: A mentor can provide you with guidance and direction as you navigate the complexities of Generative AI. They can help you set clear goals, prioritize your learning, and provide a roadmap for your growth.
- Learning from Experience: Experienced mentors have likely faced and overcome challenges similar to those you may encounter. They can share their real-world experiences, mistakes, and lessons learned, allowing you to avoid common pitfalls and make informed decisions.
- Access to Resources: Mentors often have access to resources, research materials, and opportunities that can significantly enhance your learning experience. They may introduce you to specialized tools, datasets, or research papers that accelerate your understanding.
5. Advanced Topics and Specialization
Once you’ve established a strong foundation in the Generative AI learning path by understanding the fundamentals and gaining practical experience, it’s time to explore advanced topics and specialize in areas that align with your interests and career goals.
- Generative Adversarial Networks (GANs): GANs are a fascinating and essential area within Generative AI. They consist of two neural networks, a generator, and a discriminator, that compete against each other. The generator tries to create realistic data, while the discriminator aims to distinguish between real and generated data.
- Variational Autoencoders (VAEs): VAEs are another critical aspect of Generative AI. They are used for generative tasks, but they also have applications in data compression and unsupervised learning.
- Reinforcement Learning for Generative Tasks: Reinforcement learning can be applied to generative tasks where agents learn to interact with an environment to maximize a reward signal.
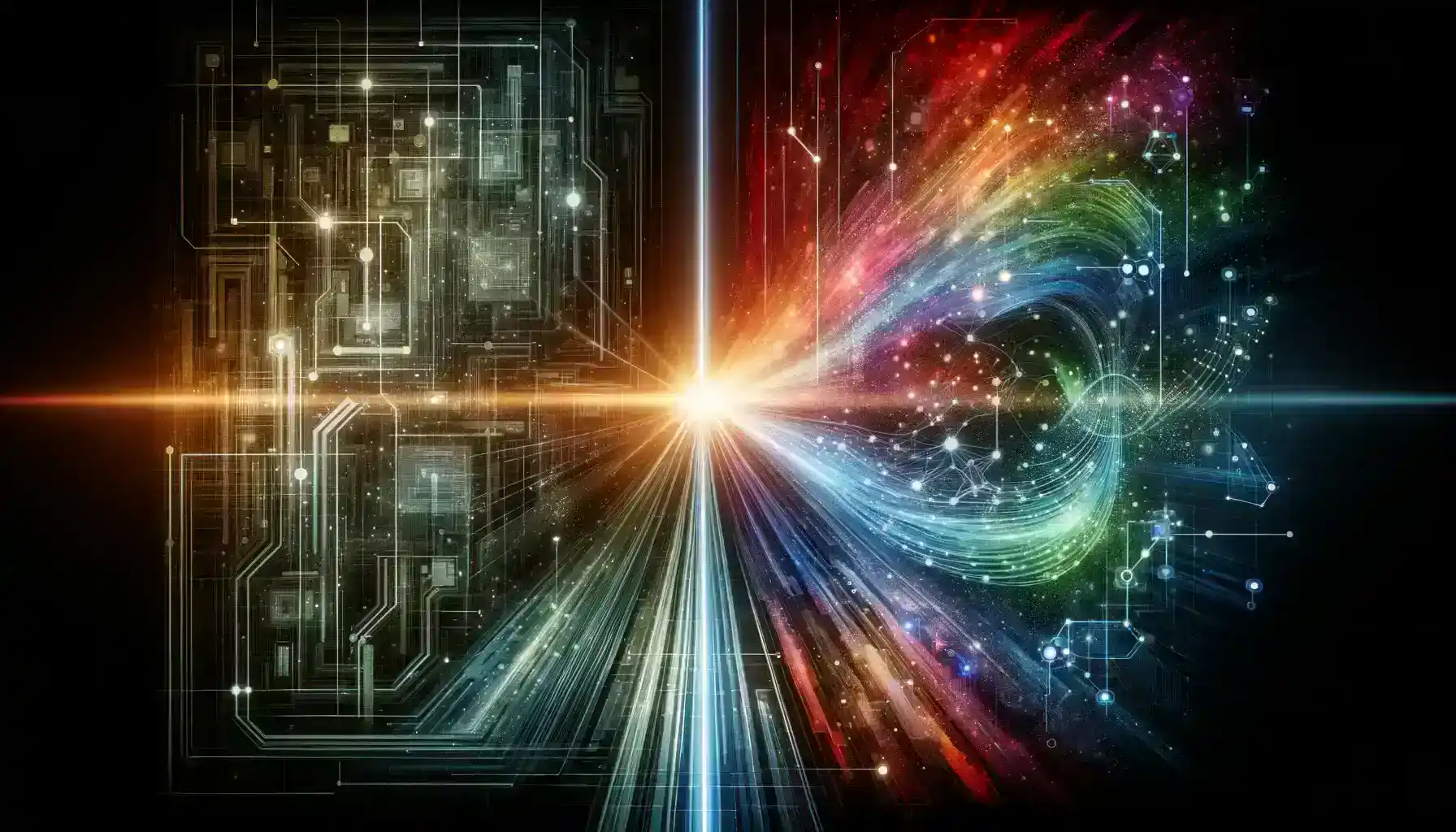
Real-World Applications
- Natural Language Processing (NLP): In the realm of NLP, Generative AI plays a significant role. It can be used for text generation, chatbots, and even content creation. Language models like GPT-3 have demonstrated the ability to generate human-like text, revolutionizing content generation and customer support. Additionally, a prime example can be an AI sentence generator by summarizer.org. The tool works on both NLP and Gen AI technologies to create quality sentences according to the input prompt.
- Healthcare: Generative AI is making strides in healthcare. It can generate synthetic medical images for training machine learning models, simulate the effect of drugs on biological systems, and even assist in drug discovery by generating molecular structures. Additionally, it’s used in predicting disease outcomes and generating medical reports.
Conclusion
Embarking on the journey from basics to brilliance in the Generative AI leawwwwrning path is an exciting and transformative endeavor. By following the expert strategies outlined in this article, you can pave a path to mastery in this rapidly evolving field.
Understanding the fundamentals, choosing the right resources, gaining hands-on experience, staying updated, and collaborating with peers are all vital components of your learning journey.
Generative AI offers a wealth of real-world applications, from art and entertainment to healthcare and finance, and by identifying opportunities to apply your expertise, you can contribute to meaningful advancements in various industries.