Table of Contents
The integration of technology with healthcare has always shown promising results. Artificial Intelligence (AI), especially its generative aspect, is at the forefront of this evolution. Let’s delve into the diverse applications of Generative AI in healthcare and its transformative potential.
Generative AI Applications in Healthcare
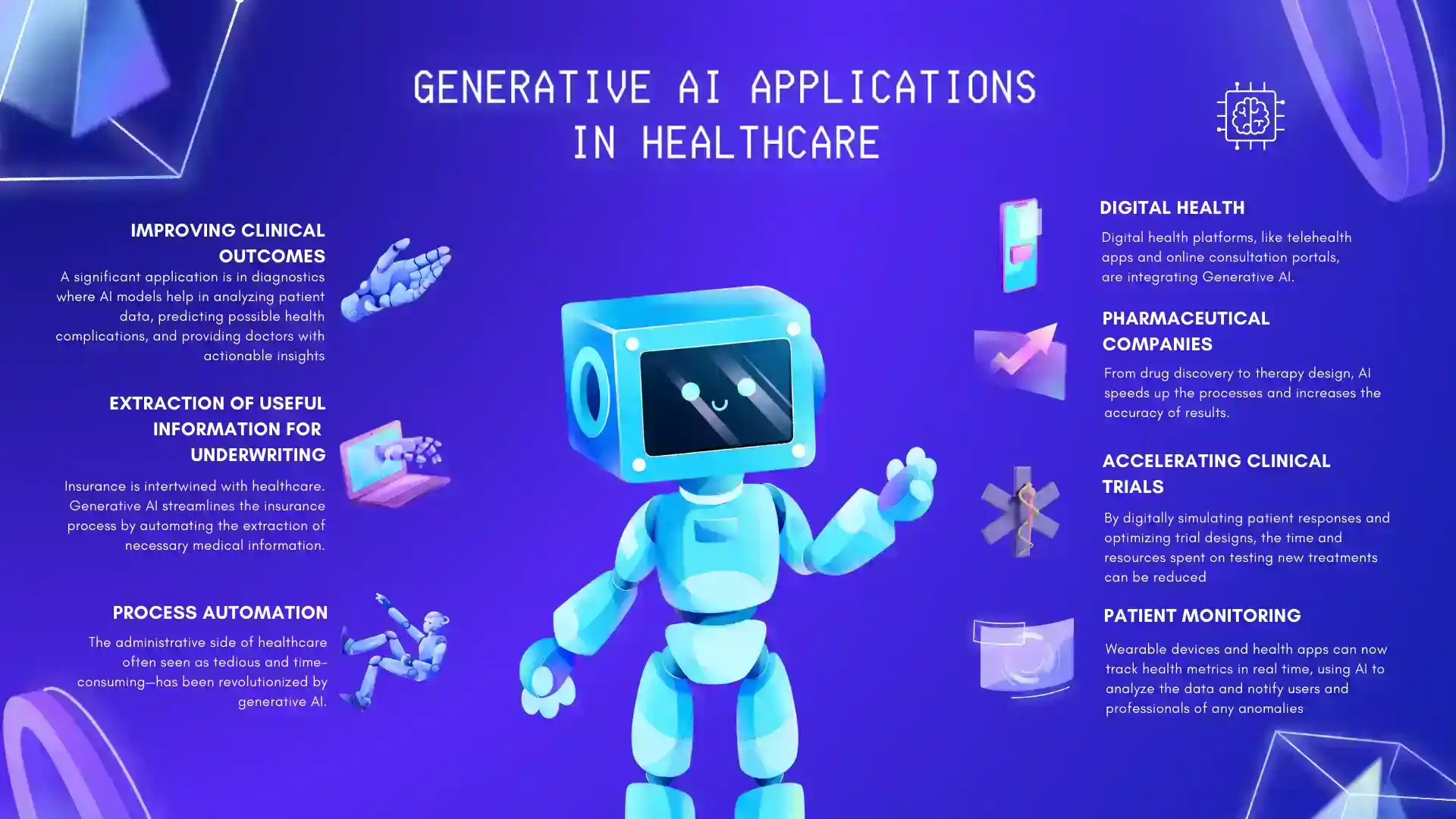
1. Improving Clinical Outcomes
Generative AI aims to refine patient care processes and outcomes. A significant application is in diagnostics where AI models help in analyzing patient data, predicting possible health complications, and providing doctors with actionable insights. The objective is simple: early detection leads to timely intervention.
2. Extraction of Useful Information for Underwriting
Insurance is intertwined with healthcare. Generative AI streamlines the insurance process by automating the extraction of necessary medical information. This aids in faster and more accurate risk assessment, leading to quicker approval or denial of claims. As AI becomes more proficient, patients might experience reduced waiting times for insurance decisions.
3. Process Automation
The administrative side of healthcare often seen as tedious and time-consuming—has been revolutionized by generative AI. Whether it’s patient onboarding, electronic record maintenance, or claims processing, AI ensures these tasks are carried out with increased efficiency, allowing healthcare professionals to focus more on patient care.
4. Digital Health
Digital health platforms, like telehealth apps and online consultation portals, are integrating Generative AI. This not only personalizes the patient experience but also optimizes the treatment pathways. A notable example is AI-driven chatbots which guide patients, providing instant medical advice and reducing the strain on medical professionals.
5. Patient Monitoring
Generative AI is changing the face of patient monitoring. Wearable devices and health apps can now track health metrics in real time, using AI to analyze the data and notify users and professionals of any anomalies. This promotes proactive healthcare and timely interventions, especially beneficial for patients with chronic conditions.
6. Pharmaceutical Companies
The pharmaceutical realm is abuzz with the possibilities Generative AI offers. From drug discovery to therapy design, AI speeds up the processes and increases the accuracy of results. With the assistance of AI, drug trials are more efficient, and the pathway from laboratory research to patient availability is significantly shortened.
7. Accelerating Clinical Trials
Generative AI’s potential doesn’t stop at drug discovery. It’s playing a pivotal role in enhancing the clinical trial process. By digitally simulating patient responses and optimizing trial designs, the time and resources spent on testing new treatments can be reduced, ensuring quicker access to groundbreaking therapies for patients.
Potential Uses of Generative AI in Healthcare
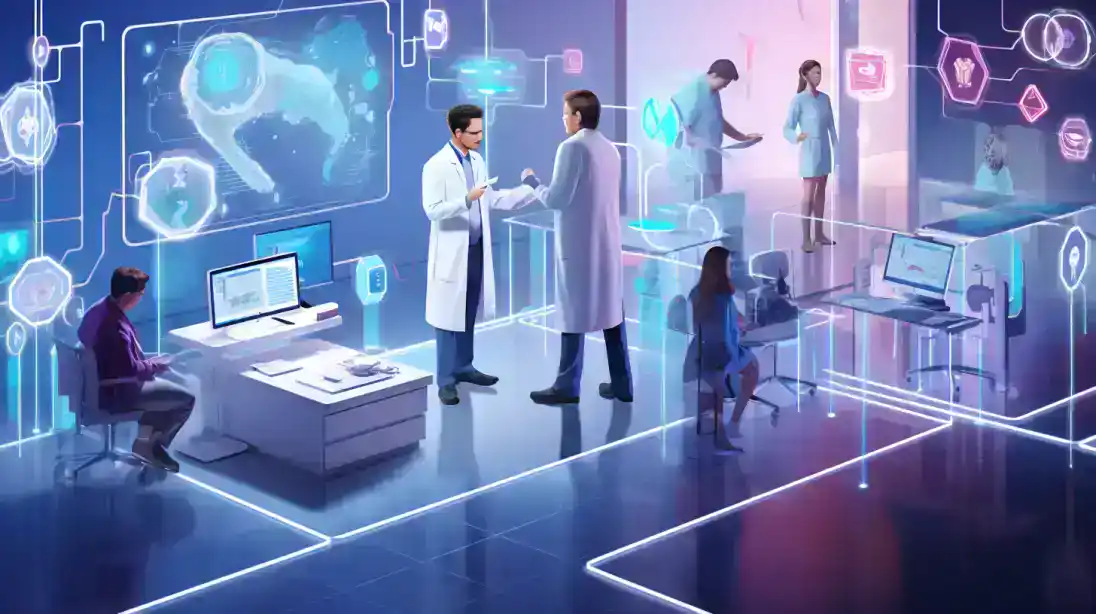
Healthcare is continuously evolving, with technology at the helm of its transformation. The potential of Generative AI in healthcare is vast. Global Generative Ai in Healthcare market has reached a valuation of USD 0.8 Billion. Here’s how it could reshape two vital segments:
(a)Private Payers
Healthcare Management:
Generative AI can process and synthesize clinical notes for care managers, making it easier to generate care plans and comprehensive summaries for members.
Member Services:
Customized coverage summaries addressing specific benefit queries can be produced. AI-driven chatbots can suggest healthcare providers based on various factors, thereby improving member-provider relations.
Provider Relationship Management:
There’s potential in using AI to contrast plan features, generate standard communications, and draft reports for performance evaluation.
Corporate Functions:
Generative AI in healthcare can be instrumental in human resources, creating onboarding videos, generating responses to RFPs, and automating many internal processes, including healthcare accounting and regulatory updates.
Claims Management:
AI’s capability to aggregate complex claims information can reduce processing time and enhance efficiency in claims resolution.
Marketing and Sales:
By analyzing customer data, AI can assist in tailoring personalized plans and in developing draft materials to simplify the sales process.
(b)Hospitals and Physician Groups:
Continuity of Care:
Generative AI ensures streamlined patient care, offering summarized discharge details and effectively synthesizing specialist notes for primary healthcare teams.
Quality and Safety:
By leveraging AI, customized risk assessments can be formulated for patients, enhancing their safety.
Value-based Care:
AI can ensure precise documentation and assist in the creation of patient-centric education materials.
Network and Market Insights:
Generative AI in healthcare can provide in-depth market performance summaries and highlight insights that drive decisions.
Reimbursement:
AI can automate prior authorization documents, code generation based on patient data, and pinpoint coding errors across claims.
Clinical Operations:
AI can produce post-visit summaries, streamline care coordination notes, and even develop personalized training paths for clinicians.
Corporate Functions:
From IT to finance, generative AI can assist in various functions such as drafting RFPs, cybersecurity test-case generation, and financial report creation.
Clinical Analytics:
Generative AI in healthcare can automate coding tasks and offer insights through conversational language analytics.
Consumer:
By analyzing customer feedback, generative AI can tailor patient care instructions, improve chatbot interfaces, and automate notifications.
In a nutshell, Generative AI in healthcare holds promise in revolutionizing both private payer segments and hospital groups, catering to a broad spectrum of needs. As healthcare becomes more data-driven, AI’s role is bound to become more integral, ensuring efficiency, personalization, and safety.
The Potential Benefits of GenAI in Healthcare
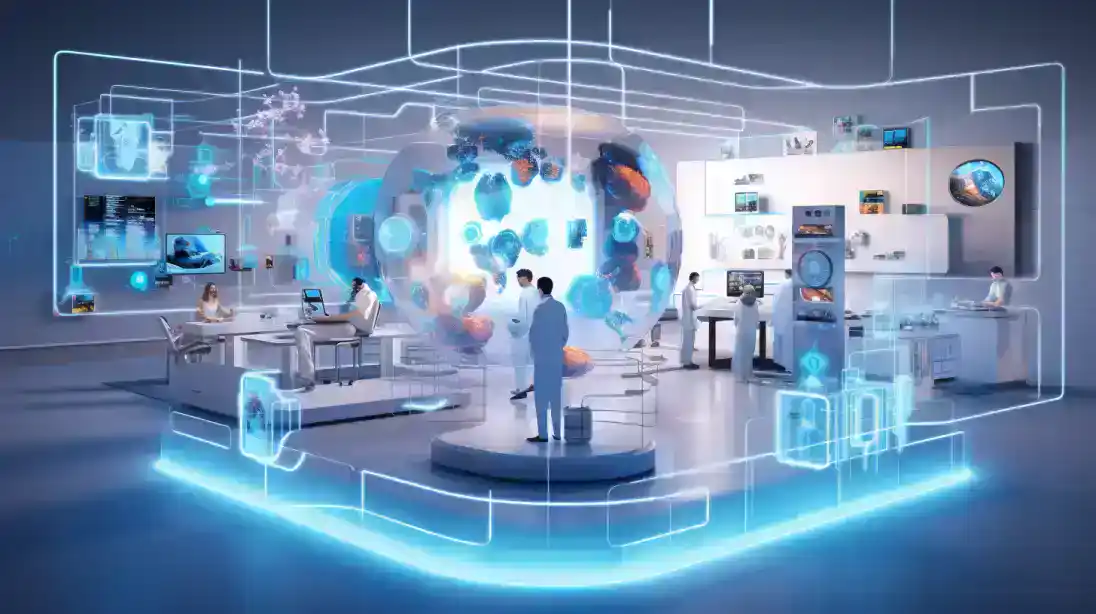
As healthcare transitions into a more technologically advanced era, GenAI emerges as a pivotal tool, offering benefits in several spheres. The capability of GenAI to simulate and generate complex data holds significant implications for the healthcare domain.
Enhancing Medical Imaging and Diagnostics:
Medical imaging is indispensable for diagnostics and treatment planning. With the introduction of GenAI techniques, such as Generative Adversarial Networks (GANs) and Variational Autoencoders (VAEs), the landscape of medical image analysis has undergone a profound transformation. These algorithms, adept at producing synthetic medical visuals mirroring real patient data, play a substantial role in validating and training machine learning models. Additionally, they address the issue of data scarcity, by producing supplementary samples, thereby refining the preciseness of image-dependent diagnoses.
Facilitating Drug Discovery and Development:
The path to drug discovery and its subsequent development is intricate and often marred by financial and time constraints. GenAI emerges as a beacon of hope in this domain by introducing virtual molecules and compounds equipped with desired traits. This facilitates researchers in their quest to navigate the expansive realm of chemical compounds, leading to the inception of pioneering drug candidates. With GenAI’s adeptness at assimilating existing drug structures and related attributes, it becomes feasible to introduce molecules aligned with the required specifications.
Read about some advances of generative AI in drug discovery
Personalized Medicine and Treatment:
The ethos of personalized medicine is to provide tailor-made treatment plans, considering the unique intricacies of each patient. GenAI, with its data analysis prowess, can sift through copious amounts of patient data – be it electronic health records, genetic makeup, or clinical results. This allows GenAI to devise bespoke treatment recommendations, anticipate disease trajectories, and predict how patients might react to various interventions, bestowing healthcare professionals with insights for effective decision-making.
Medical Research and Knowledge Generation:
Medical research often grapples with the challenge of data sensitivity. GenAI offers a solution by producing synthetic data tailored to meet specific parameters. This not only preserves patient confidentiality but also paves the way for researchers to glean essential insights. The synthetic data, while mirroring real-world scenarios, grants researchers the liberty to explore innovative hypotheses without compromising on data security.
Challenges and Limitations of Generative AI in Healthcare
While GenAI offers transformative potential in healthcare, it comes with certain challenges that demand attention:
Reliability and Accuracy:
GenAI’s effectiveness hinges on the precision of its outputs. Biases or inaccuracies can jeopardize patient care. Ensuring rigorous validation of the models is essential to maintain the integrity of therapeutic decisions.
Privacy and Data Security:
With GenAI utilizing vast patient data, robust data protection is critical. Advanced anonymization methods and secure data-sharing frameworks are vital to maintaining patient trust and compliance with regulations.
Ambiguity and Interpretability:
The complex nature of some AI models can lead to doubts among healthcare professionals. Demystifying GenAI’s decision-making process is crucial for its wider acceptance and trustworthiness in medical settings.
Embrace the future of GenAI in healthcare. With unparalleled potential, generative AI is set to revolutionize healthcare in ways beyond our current imagination. As it matures, the convergence of GenAI with technologies like virtual and augmented reality offers a new horizon for healthcare delivery. Dive in and be part of this transformative journey.
FAQS
What challenges does generative AI face in healthcare?
A. Some challenges include ensuring the reliability and accuracy of generated outputs, adhering to privacy and data security standards, and addressing ambiguity and interpretability in complex models.
Is genAI in healthcare limited to only imaging?
A. No, while imaging is a prominent application, GenAI also extends to drug discovery, personalized medicine, research, and knowledge generation among other areas.
How do healthcare professionals interact with genAI models?
A. Professionals typically interact through specialized software interfaces. These tools allow them to input data, interpret outputs, and integrate AI-generated insights into their decision-making processes.
How is the data used to train generative AI models sourced?
A. The data is sourced from diverse sets including electronic health records, clinical trials, genetic databases, and imaging repositories, ensuring comprehensive and varied information for training.
How quickly is generative AI advancing in healthcare?
A. GenAI is rapidly advancing in healthcare, driven by the increasing availability of data, advances in computing power, and continuous research in AI algorithms. As more successful implementations emerge, its adoption rate in healthcare is expected to grow.