Table of Contents
Diversified AI: Chess puzzles are not just a fun way to test your skills, but also a challenge for artificial intelligence (AI). Some puzzles are so hard that even the best chess programs fail to find the right moves, while human players can easily solve them. What makes these puzzles so difficult for computers, and how can AI overcome them?
This is the question that intrigued Tom Zahavy, a computer scientist and chess enthusiast who works at Google DeepMind. He was fascinated by the puzzles created by Sir Roger Penrose, a mathematician and Nobel laureate, who showed that computers often misjudge the position of the pieces on the board and give the wrong advice. Penrose’s puzzles revealed a gap between human and machine intelligence and inspired Zahavy to find a way to bridge it.
Diversified AI: A Multi-Agent Approach for Creative Problem-Solving
Zahavy and his colleagues came up with a novel idea: instead of using a single AI program to solve the puzzles, why not use a team of different AI agents, each with its own strategy and style? They created a new system that combines up to 10 AI agents, based on DeepMind’s powerful chess program AlphaZero, and lets them collaborate and compete to find the best moves. The system also rewards diversity, encouraging the agents to explore different options and avoid getting stuck in a rut.
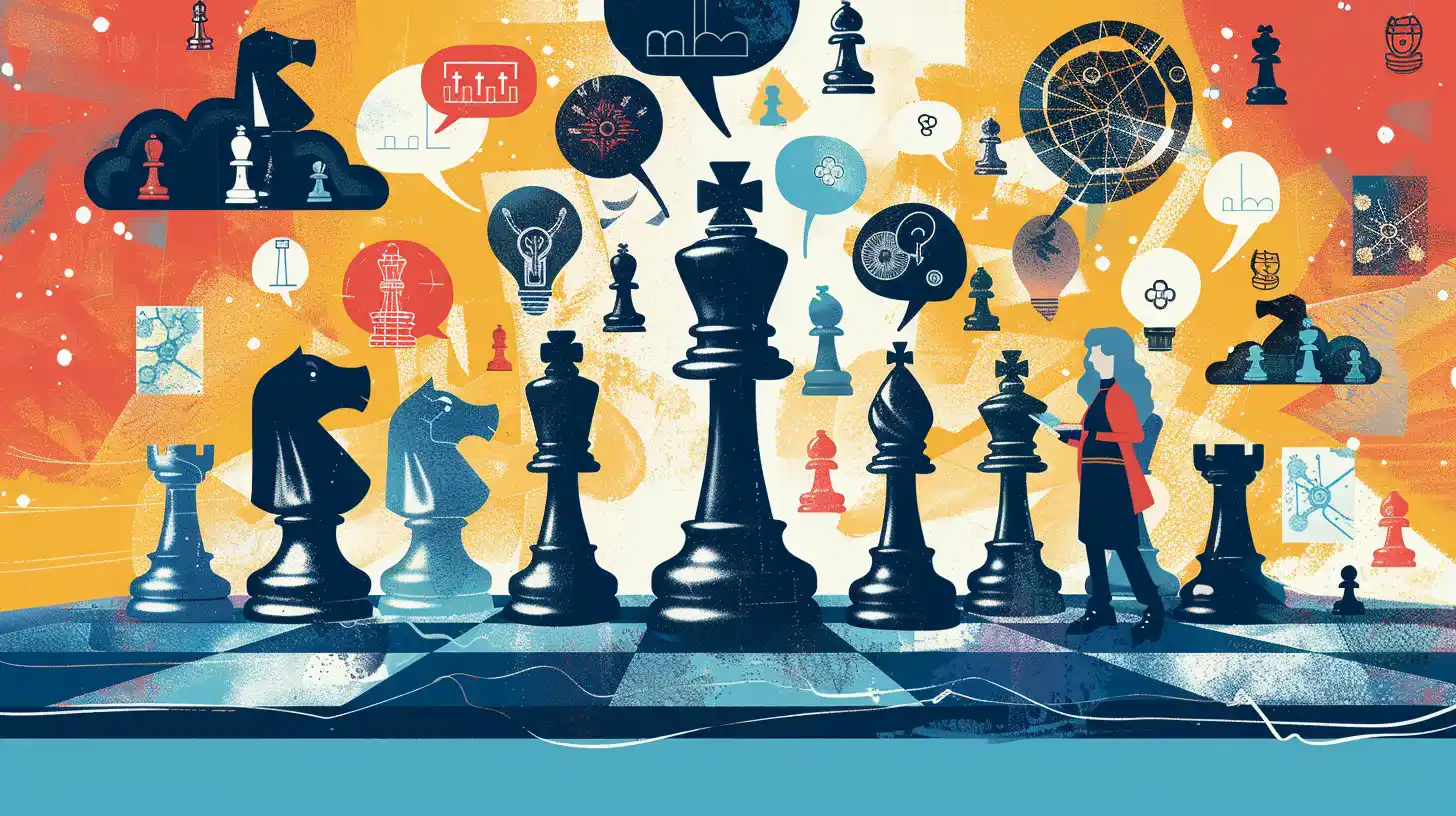
The results were impressive: the new system, dubbed diversified AI, outperformed AlphaZero in chess games and puzzles and showed more skill and creativity. It solved more than half of the Penrose puzzles, and twice as many challenge puzzles as AlphaZero. It also experimented with new openings and tactics, such as casting on different sides of the board. The system demonstrated that having multiple perspectives and approaches can help AI solve hard problems and discover new possibilities.
The benefits of diversified AI are not limited to chess. The system could also tackle other domains that require creativity and problem-solving, such as robotics, drug discovery, and stock trading. Antoine Cully, an AI researcher at Imperial College London who was not involved in the project, praised the work as a great example of how looking for more than one way to solve a problem can lead to better solutions. He compared it to a human brainstorming session, where different ideas are generated and evaluated.
Zahavy’s work also sheds light on the nature of intelligence and creativity. He suggested that intelligence is a matter of computational power and that creativity is a matter of considering and selecting from a large enough set of options. By increasing the diversity and complexity of AI agents, he hoped to emulate the creative problem-solving strategies that humans use. He also acknowledged that there is still room for improvement and that there may not be a single answer to every question.
Embracing Diversity: The Exciting Potential of Diversified AI in AI Research
Diversified AI is a promising and exciting direction for AI research. It shows that AI can learn from itself, and from others and that cooperation can lead to innovation. It also challenges the notion that AI is a monolithic and rigid entity and reveals that it can be flexible and diverse. As Zahavy said, “It’s not clear to me that given all the data in the world.
Diversified AI is a term that refers to the use of multiple AI agents or systems that have different strategies, styles, or objectives to solve a problem or perform a task. The idea is that by combining the strengths and perspectives of different AI agents, the overall system can achieve better results, more creativity, and more generalization than a single AI agent.
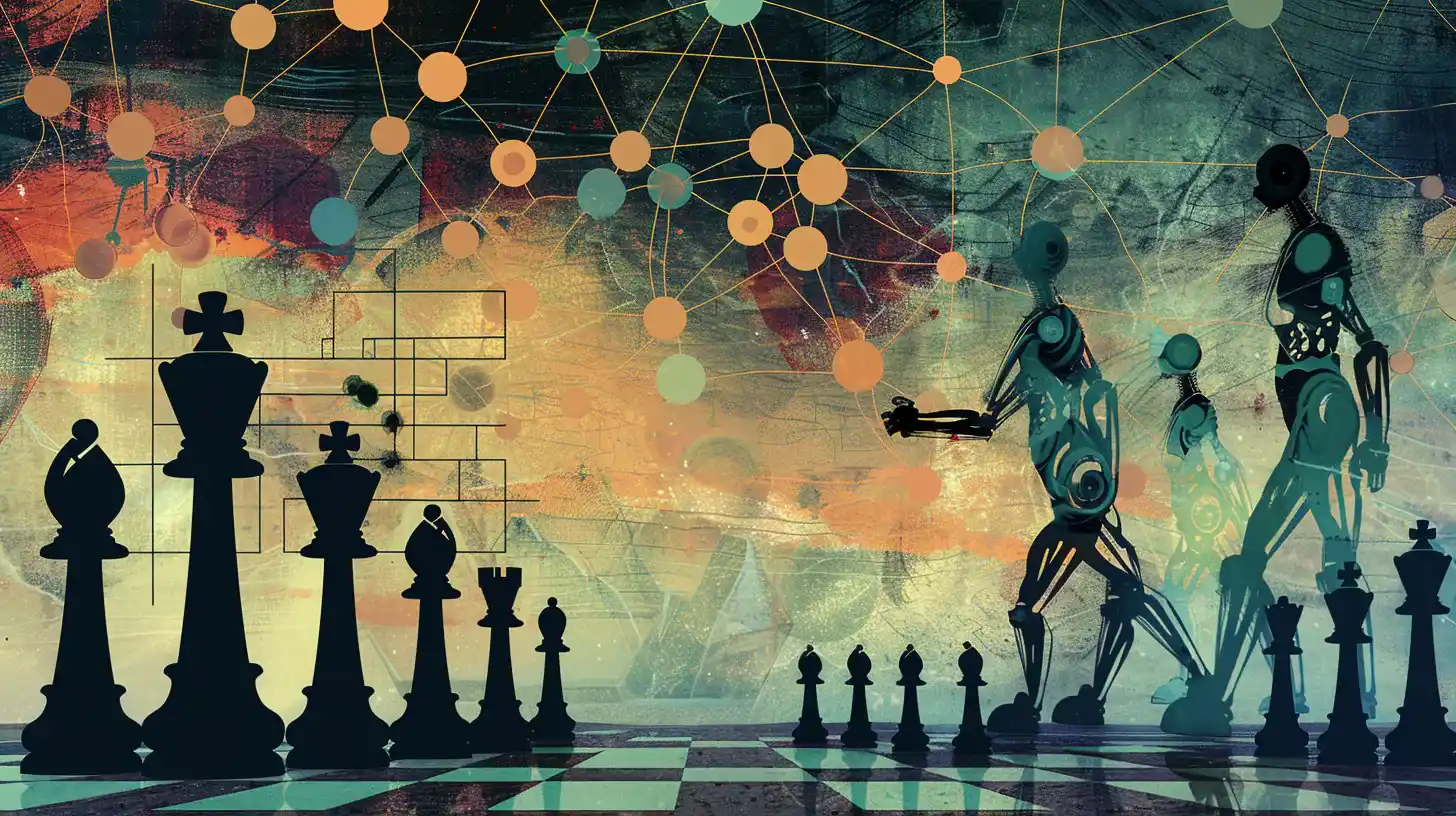
One example of diversified AI is the system developed by Google DeepMind to solve chess puzzles that are hard for traditional AI programs. The system consists of up to 10 AI agents, each based on DeepMind’s powerful chess program AlphaZero, but trained on different situations and rewarded for diversity. The system can switch between the agents depending on the position and the puzzle and can solve more puzzles and play more creatively than AlphaZero alone.
Another example of diversified AI is the system developed by Imperial College London to control a six-legged robot that can adapt to different environments and injuries. The system uses a large collection of different solutions for moving the robot, each generated by a different AI agent. The system can select the best solution for the current situation, or combine different solutions to create new ones.
Applications and Challenges of Diversified AI: Navigating the Potential and Pitfalls
Diversified AI has many potential applications and benefits for various domains that require creativity and problem-solving, such as robotics, drug discovery, and stock trading. It can also help AI systems learn from themselves and from others, and cooperate to achieve innovation. It can also challenge the notion that AI is a monolithic and rigid entity, and reveal that it can be flexible and diverse.
Some of the challenges and limitations of diversified AI include the computational cost of maintaining and coordinating multiple AI agents, the difficulty of ensuring that the agents are aligned and compatible with each other and with the overall goal, and the ethical and social implications of having AI systems that can act in unpredictable and diverse ways.