Table of Contents
Introduction to Digital Twin Technology in Manufacturing
Definition of Digital Twin
Digital Twin technology is revolutionizing manufacturing, offering unprecedented levels of efficiency and innovation. By creating a virtual representation of physical systems, digital twins enable real-time monitoring, predictive maintenance, and process optimization.
According to a recent market analysis, the global digital twin market is expected to grow from USD 3.1 billion in 2020 to USD 48.2 billion by 2026, reflecting a compound annual growth rate (CAGR) of 58%. This significant revenue potential highlights the transformative impact digital twins can have on the manufacturing industry, driving productivity, reducing costs, and improving product quality.
Overview of Manufacturing Processes and Their Complexities
Manufacturing processes are inherently complex, involving a multitude of operations, equipment, and workflows. These processes encompass various stages such as design, production, quality control, and maintenance. The complexity is further amplified by factors such as:
- Diverse Production Lines: Different products require specialized production lines, each with its unique set of machinery and operational protocols.
- Supply Chain Interdependencies: Manufacturing relies on a well-coordinated supply chain to ensure the timely availability of raw materials and components.
- Quality Assurance: Maintaining high standards of quality necessitates rigorous testing and inspection at multiple stages of production.
- Resource Optimization: Efficient utilization of resources, including labor, materials, and energy, is critical to maintaining profitability and sustainability.
- Dynamic Market Demands: Manufacturers must adapt to changing market demands, necessitating flexibility and agility in their processes.
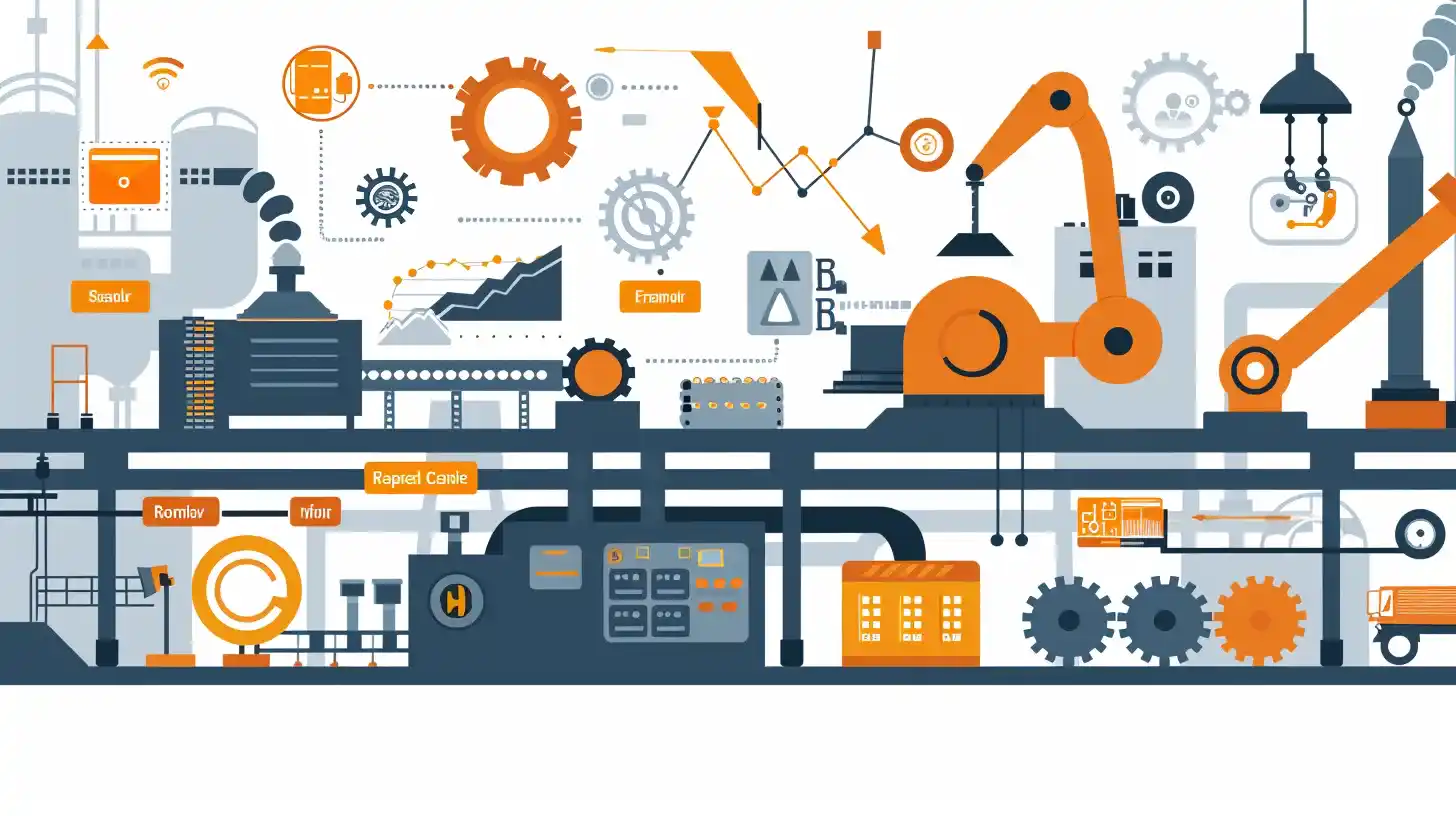
The Need for Real-Time Monitoring and Optimization
Given the complexities inherent in manufacturing, real-time monitoring and optimization are essential for several reasons:
- Operational Efficiency: Continuous monitoring of equipment and processes helps identify bottlenecks, reduce downtime, and enhance overall efficiency.
- Predictive Maintenance: Real-time data enables predictive maintenance strategies, reducing unexpected failures and extending the lifespan of machinery.
- Quality Control: Monitoring production parameters in real-time ensures adherence to quality standards, minimizing defects and rework.
- Resource Management: Optimizing the use of resources in real time helps lower costs and improve sustainability.
- Adaptability: Real-time insights allow manufacturers to quickly respond to market changes, adjusting production schedules and processes as needed.
The Potential of Digital Twin Technology to Address These Needs
Digital twin technology holds significant potential to address the challenges of modern manufacturing by providing a comprehensive, real-time view of operations. Key benefits include:
- Enhanced Decision-Making: digital twin technology offers detailed insights into the performance of systems, enabling informed and data-driven decision-making.
- Improved Efficiency: By simulating different scenarios and predicting outcomes, digital twins help optimize production processes and reduce waste.
- Reduced Downtime: Predictive analytics within digital twin technology foresee equipment failures before they occur, allowing for preemptive maintenance and reducing downtime.
- Quality Assurance: Continuous monitoring and simulation ensure consistent quality by identifying deviations and implementing corrective measures promptly.
- Cost Savings: Optimized processes, predictive maintenance, and improved resource management contribute to significant cost savings.
Understanding Digital Twin Technology
Core Components of a Digital Twin
Digital twin technology is built upon several key components that work together to create an accurate and dynamic representation of physical systems:
- Virtual Model: The virtual model is a digital replica of the physical object or system. It incorporates detailed geometry, material properties, and operational characteristics to mimic the real-world entity accurately. This model serves as the foundation for simulations and analyses.
- Data Connection: A robust data connection is essential for ensuring real-time synchronization between the physical object and its digital counterpart. This involves continuous data flow from the physical entity to the virtual model, enabling dynamic updates and accurate reflections of current states and conditions.
- Physical Object: The physical object is the real-world entity being mirrored by the digital twin technology. It can be anything from a single machine to an entire manufacturing plant. The digital twin continuously receives data from the physical object to maintain an up-to-date and precise digital representation.
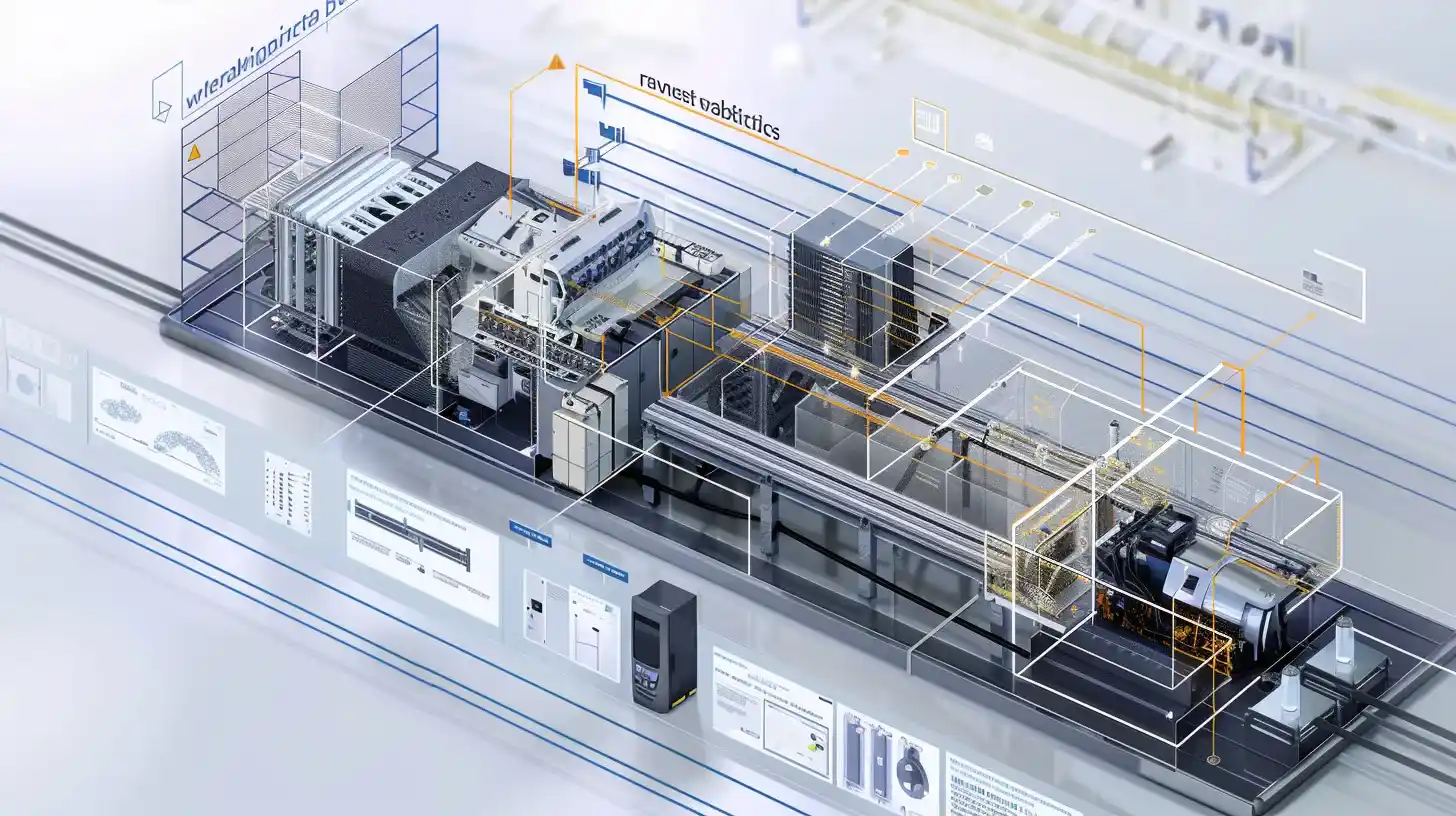
Data Acquisition and Integration
The efficacy of a digital twin relies heavily on the seamless acquisition and integration of data. This involves the following components:
- IoT Sensors: Internet of Things (IoT) sensors play a crucial role in collecting real-time data from the physical object. These sensors measure various parameters such as temperature, pressure, vibration, and performance metrics, transmitting this data to the digital twin technology.
- Data Lakes: Data lakes are centralized repositories that store vast amounts of raw data in their native format. By leveraging data lakes, organizations can aggregate and manage the data collected from IoT sensors and other sources, providing a rich dataset for the digital twin technology to utilize.
- Cloud Platforms: Cloud platforms facilitate the storage, processing, and analysis of large datasets. They offer the computational power and scalability required to handle the extensive data streams from IoT devices, making them integral to the digital twin technology infrastructure.
Modeling and Simulation Techniques
To create an effective digital twin technology, sophisticated modeling and simulation techniques are employed:
- Mathematical Models: These models use mathematical equations and algorithms to replicate the physical behaviors and interactions within the system. They provide a theoretical foundation for the digital twin’s operations and predictions.
- Finite Element Analysis (FEA): FEA is used to simulate and analyze the structural behavior of components under various conditions. This technique helps in understanding stress, strain, and deformation in materials and structures.
- Computational Fluid Dynamics (CFD): CFD models the flow of fluids (gases and liquids) within the system. It is crucial for applications involving fluid dynamics, such as cooling systems and aerodynamics.
- Agent-Based Modeling (ABM): ABM simulates the actions and interactions of autonomous agents to assess their effects on the system. It is particularly useful for complex systems with multiple interacting entities.
Visualization and Human-Machine Interface
Visualization and human-machine interfaces (HMI) are essential for interacting with and understanding the digital twin:
- 3D Visualization: Advanced 3D visualization techniques provide a realistic and immersive view of the digital twin technology. This helps engineers and operators visualize the system’s behavior, identify issues, and explore potential solutions.
- Dashboards: Interactive dashboards display key performance indicators (KPIs), real-time data, and predictive analytics. They offer a comprehensive overview of the system’s status and performance, aiding in decision-making.
- Augmented Reality (AR) and Virtual Reality (VR): AR and VR technologies enhance the visualization capabilities of digital twin technology. They allow users to interact with the virtual model in a more intuitive and immersive manner, facilitating better understanding and training.
- Human-Machine Interface (HMI): HMIs provide user-friendly interfaces for operators to interact with the digital twin technology. These interfaces enable users to monitor real-time data, run simulations, and control the physical system remotely.
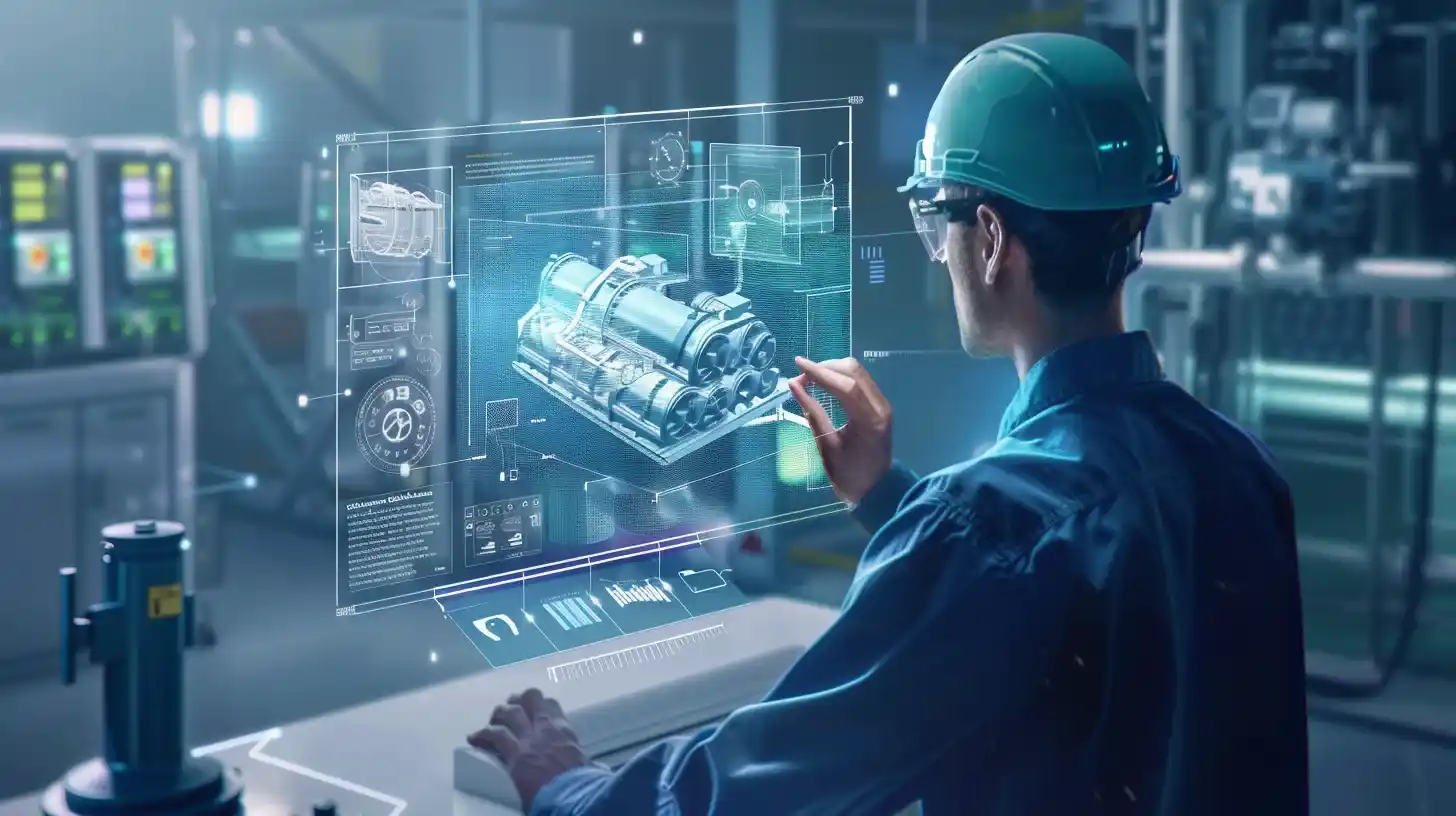
Application of Digital Twins in Manufacturing
Real-Time Monitoring of Production Processes
digital twin technology enables real-time monitoring of production processes by continuously collecting and analyzing data from various sensors and devices within the manufacturing environment. This real-time insight allows manufacturers to track the status of machines, monitor production rates, and ensure that operations are running smoothly.
By visualizing the entire production process in real time, manufacturers can quickly identify and address any deviations or issues that may arise.
Key Performance Indicators (KPIs) Tracking
digital twin technology facilitates the tracking of key performance indicators (KPIs) by providing a comprehensive view of manufacturing operations. KPIs such as production output, machine uptime, cycle times, and defect rates can be monitored and analyzed through the digital twin. This enables manufacturers to measure performance against targets, identify trends, and make informed decisions to improve overall efficiency and productivity.
Anomaly Detection and Root Cause Analysis
One of the significant benefits of digital twin technology is its ability to detect anomalies and perform root cause analysis. By continuously monitoring data and using advanced analytics, digital twins can identify deviations from normal operating conditions. When an anomaly is detected, the digital twin can simulate various scenarios to determine the root cause, enabling quick and effective resolution of issues. This helps prevent downtime, reduce waste, and maintain product quality.
Predictive Maintenance
Predictive maintenance is a critical application of digital twin technology in manufacturing. By analyzing historical data and real-time sensor information, digital twins can predict when equipment is likely to fail or require maintenance. This allows manufacturers to schedule maintenance activities proactively, reducing unplanned downtime and extending the lifespan of machinery. Predictive maintenance also helps optimize maintenance schedules and reduce maintenance costs.
Optimization of Manufacturing Processes
digital twin technology plays a crucial role in optimizing manufacturing processes. By simulating different production scenarios and analyzing their outcomes, manufacturers can identify the most efficient ways to operate their processes. This includes optimizing production schedules, minimizing energy consumption, and reducing material waste. Digital twins enable continuous improvement by providing insights into how changes in process parameters can impact overall performance.
Process Simulation and Modeling
digital twin technology allows manufacturers to simulate and model various aspects of their production processes. These simulations can include everything from the behavior of individual machines to the flow of materials through the entire production line.
By creating a virtual representation of the manufacturing process, manufacturers can test new strategies, evaluate the impact of changes, and validate process improvements before implementing them in the real world. This reduces risks and accelerates innovation.
Identifying Bottlenecks and Inefficiencies
One of the significant advantages of digital twin technology is its ability to identify bottlenecks and inefficiencies in manufacturing processes. By analyzing data from the digital twin technology, manufacturers can pinpoint areas where production is slowing down or where resources are not being utilized effectively.
Once identified, these bottlenecks can be addressed through process optimization, equipment upgrades, or workflow adjustments, leading to smoother and more efficient operations.
Improving Resource Allocation and Utilization
Digital twin technology helps manufacturers improve resource allocation and utilization by providing detailed insights into how resources are being used. This includes labor, materials, and equipment.
By analyzing data from the digital twin, manufacturers can identify areas where resources are underutilized or overburdened and make adjustments to balance workloads and optimize resource usage. This leads to cost savings and more efficient production processes.
Enhancing Product Quality and Consistency
Ensuring product quality and consistency is a critical concern for manufacturers. digital twin technology contributes to this by monitoring and controlling production parameters in real time. By maintaining optimal conditions and promptly addressing any deviations, digital twins help reduce defects and ensure that products meet quality standards.
Additionally, digital twins can simulate the impact of process changes on product quality, enabling manufacturers to make data-driven decisions that enhance consistency and reliability.
Benefits and Challenges of Digital Twin Implementation
Benefits of Digital Twin Implementation
Increased Productivity and Efficiency
- Optimized Operations: Digital twins enable the continuous monitoring and optimization of production processes, leading to more efficient operations.
- Resource Utilization: By providing detailed insights into resource usage, digital twins help manufacturers allocate labor, materials, and equipment more effectively.
- Process Automation: Automated adjustments based on real-time data can reduce manual intervention, streamline workflows, and increase throughput.
Improved Product Quality and Consistency
- Real-Time Quality Control: Digital twins monitor production parameters in real time, ensuring that products meet quality standards consistently.
- Defect Reduction: By identifying and addressing anomalies early in the production process, digital twins help reduce the occurrence of defects.
- Simulation and Testing: Manufacturers can simulate and test process changes within the digital twin to evaluate their impact on product quality before implementation.
Reduced Downtime and Maintenance Costs
- Predictive Maintenance: Digital twins predict equipment failures and maintenance needs based on historical and real-time data, enabling proactive maintenance scheduling.
- Minimized Unplanned Downtime: Predictive insights reduce the likelihood of unexpected equipment failures, minimizing production disruptions.
- Extended Equipment Lifespan: Timely maintenance based on predictive analytics helps extend the lifespan of machinery, reducing replacement costs.
Enhanced Decision-Making
- Data-Driven Insights: Digital twins provide a wealth of data and analytics, enabling manufacturers to make informed decisions based on accurate, real-time information.
- Scenario Analysis: Manufacturers can use digital twins to simulate different scenarios and evaluate potential outcomes, supporting strategic planning and risk management.
- Improved Collaboration: Digital twins facilitate better communication and collaboration among teams by providing a unified view of production processes and performance.
Challenges in Digital Twin Adoption
Data Management and Security
- Data Volume: Managing the vast amounts of data generated by IoT sensors and other sources can be challenging.
- Data Integration: Integrating data from disparate systems and ensuring data consistency requires sophisticated data management strategies.
- Security Concerns: Protecting sensitive data from cyber threats and ensuring compliance with data privacy regulations are critical concerns.
Model Development and Validation
- Complex Modeling: Developing accurate and comprehensive digital models that reflect real-world systems is a complex task requiring specialized expertise.
- Continuous Validation: Digital twins need to be continuously validated and updated to ensure they remain accurate and relevant over time.
- Resource Intensive: Model development and validation can be resource-intensive, requiring significant time and investment.
Integration with Existing Systems
- Legacy Systems: Integrating digital twins with existing legacy systems and infrastructure can be challenging and may require significant modifications.
- Interoperability: Ensuring interoperability between different systems and technologies is essential for seamless data flow and functionality.
- Change Management: Implementing digital twins often involves changes in processes and workflows, requiring effective change management strategies to ensure smooth adoption.
Return on Investment (ROI)
- Initial Costs: The initial costs of implementing digital twin technology, including hardware, software, and expertise, can be substantial.
- Measuring ROI: Quantifying the benefits and calculating the return on investment can be challenging, particularly in the early stages of implementation.
- Long-Term Gains: Achieving a positive ROI may take time, as the benefits of digital twins often accrue gradually through ongoing optimization and improvements.
Case Studies of Digital Twin Implementation in Manufacturing
Successful Implementation of Digital Twins in Manufacturing Industries
Case Study 1: General Electric (GE) Aviation
Implementation: General Electric (GE) Aviation has implemented digital twin technology to monitor and optimize the performance of its aircraft engines. Each engine has a digital twin that collects real-time data from various sensors during flight.
Results and Impact:
- Increased Efficiency: By analyzing the data, GE can predict maintenance needs and optimize engine performance, leading to significant improvements in fuel efficiency.
- Reduced Downtime: Predictive maintenance has reduced unplanned engine removals by up to 25%.
- Cost Savings: GE estimates that digital twins have saved airlines millions of dollars in maintenance and operational costs.
Case Study 2: Siemens Amberg Electronics Plant
Implementation: Siemens implemented digital twins at its Amberg Electronics Plant to create a highly automated and digitalized manufacturing environment. The plant produces Programmable Logic Controllers (PLCs) and uses digital twins to simulate and optimize production processes.
Results and Impact:
- Productivity Gains: The digital twin technology has increased the plant’s productivity by 75%, with minimal human intervention required.
- Quality Improvement: The defect rate has been reduced to nearly zero, significantly enhancing product quality.
- Efficiency: The plant operates with a 99.99885% reliability rate, thanks to the predictive and real-time monitoring capabilities of digital twins.
Case Study 3: Tesla’s Gigafactory
Implementation: Tesla uses digital twins in its Gigafactory to streamline the production of lithium-ion batteries. The digital twins monitor and optimize every aspect of the manufacturing process, from material handling to final assembly.
Results and Impact:
- Enhanced Production Efficiency: Tesla has achieved a 30% improvement in production efficiency due to the real-time insights provided by digital twins.
- Predictive Maintenance: The digital twin technology helps Tesla predict and prevent equipment failures, minimizing downtime.
- Increased Output: The optimized production processes have enabled Tesla to scale up battery production rapidly to meet increasing demand.
Case Study 4: Unilever’s Consumer Goods Manufacturing
Implementation: Unilever has implemented digital twin technology across its global manufacturing operations to improve efficiency and sustainability. The digital twins are used to model and optimize production processes, energy usage, and supply chain logistics.
Results and Impact:
- Energy Savings: Unilever has achieved a 15% reduction in energy consumption across its manufacturing sites.
- Waste Reduction: The use of digital twins has helped Unilever reduce waste by 20% through better process control and optimization.
- Operational Efficiency: The technology has improved overall operational efficiency by 10%, leading to cost savings and enhanced sustainability.
Case Study 5: BMW’s Digital Factory
Implementation: BMW has integrated digital twin technology into its digital factory initiative. The technology is used to simulate and optimize the production of automotive components and vehicles.
Results and Impact:
- Production Flexibility: Digital twins have enabled BMW to increase production flexibility, allowing the company to switch between different vehicle models with minimal downtime.
- Quality Control: The technology has improved quality control processes, resulting in a 30% reduction in production defects.
- Cost Reduction: By optimizing manufacturing processes and resource utilization, BMW has achieved significant cost savings.
Quantifiable Results and Impact on Business Performance
1. Increased Efficiency and Productivity:
- General Electric: 25% reduction in unplanned engine removals.
- Siemens: 75% increase in productivity at the Amberg Electronics Plant.
- Tesla: 30% improvement in production efficiency.
2. Cost Savings:
- General Electric: Millions of dollars saved in maintenance and operational costs.
- Unilever: 15% reduction in energy consumption and 20% reduction in waste.
3. Improved Quality and Reduced Defects:
- Siemens: Near-zero defect rate.
- BMW: 30% reduction in production defects.
4. Reduced Downtime:
- General Electric: Significant reduction in unplanned downtime due to predictive maintenance.
- Tesla: Minimized equipment failures and downtime.
5. Enhanced Sustainability:
- Unilever: Improved sustainability through reduced energy consumption and waste.
Future Trends and Considerations in Digital Twin Technology
Advancements in Digital Twin Technology
Artificial Intelligence (AI) and Machine Learning (ML):
- Enhanced Predictive Capabilities: AI and ML algorithms will continue to improve the predictive capabilities of digital twins. These technologies can analyze vast amounts of data to identify patterns and predict outcomes with greater accuracy.
- Automated Decision-Making: AI-driven digital twins will enable more autonomous decision-making processes, reducing the need for human intervention and increasing operational efficiency.
- Adaptive Learning: Future digital twins will leverage machine learning to adapt and evolve based on new data and changing conditions, making them more resilient and capable of handling complex scenarios.
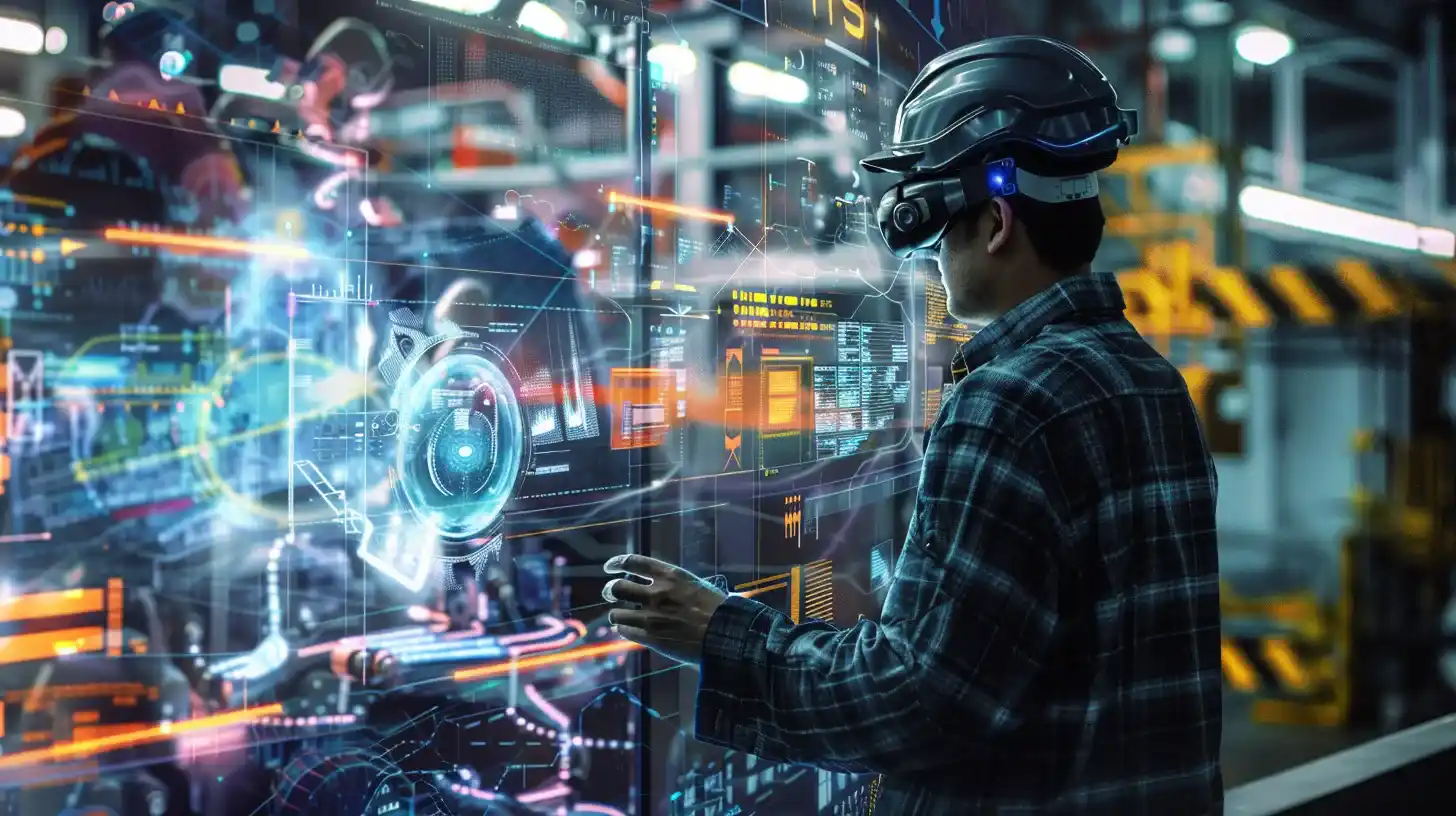
Augmented Reality (AR):
- Immersive Visualization: AR will enhance the visualization capabilities of digital twins by providing immersive, real-time views of physical objects and systems. This can be particularly useful for remote monitoring, training, and maintenance.
- Interactive Interfaces: AR-enabled digital twins will allow users to interact with virtual models in a more intuitive and engaging manner, improving understanding and collaboration.
- Real-Time Data Overlay: AR can overlay real-time data from digital twins onto physical objects, providing contextual information and insights to operators and engineers.
Emerging Applications in Manufacturing
Supply Chain Optimization:
- End-to-End Visibility: Digital twins will provide end-to-end visibility of the supply chain, enabling manufacturers to monitor and optimize every stage from raw material sourcing to product delivery.
- Risk Management: By simulating different supply chain scenarios, digital twins can help identify potential risks and disruptions, allowing companies to develop more robust contingency plans.
- Inventory Management: Digital twins can optimize inventory levels by predicting demand patterns and ensuring the right materials and products are available at the right time.
Sustainability:
- Energy Efficiency: Digital twins can monitor and optimize energy consumption in manufacturing processes, helping companies reduce their carbon footprint and achieve sustainability goals.
- Resource Management: By providing insights into resource utilization, digital twins can help manufacturers minimize waste and use resources more efficiently.
- Lifecycle Assessment: Digital twins can be used to assess the environmental impact of products throughout their lifecycle, from production to disposal, enabling more sustainable product design and manufacturing practices.
Ethical Implications and Data Privacy
Data Privacy:
- Sensitive Data Protection: As digital twins rely on vast amounts of data, ensuring the privacy and security of this data is critical. Manufacturers must implement robust data protection measures to safeguard sensitive information.
- Compliance with Regulations: Companies must comply with data privacy regulations such as GDPR and CCPA, which govern the collection, storage, and use of personal and sensitive data.
- Transparency and Consent: Organizations should maintain transparency with stakeholders regarding data collection practices and obtain explicit consent for the use of personal data.
Ethical Implications:
- Bias in AI and ML: The AI and ML algorithms powering digital twins must be designed and trained to avoid biases that could lead to unfair or discriminatory outcomes. Ensuring fairness and accountability in these systems is essential.
- Job Displacement: The increased automation and efficiency enabled by digital twins may lead to job displacement in certain roles. Companies should consider strategies for workforce reskilling and support to address these challenges.
- Decision-Making Responsibility: As digital twins become more autonomous, it is important to define clear guidelines and accountability for decision-making processes. Ensuring human oversight and ethical considerations in automated decisions is crucial.
Conclusion
Recap of the Key Points
Digital twin technology represents a significant advancement in the field of manufacturing, offering a dynamic and real-time virtual representation of physical systems. The core components of digital twins include virtual models, data connections, and physical objects, which together enable continuous monitoring and optimization.
Data acquisition through IoT sensors, data lakes, and cloud platforms, along with advanced modeling and simulation techniques, forms the backbone of this technology. Visualization tools and human-machine interfaces further enhance the usability and effectiveness of digital twins.
The application of digital twins in manufacturing encompasses several critical areas:
- Real-time Monitoring: Continuous oversight of production processes.
- KPI Tracking: Monitoring key performance indicators for better performance management.
- Anomaly Detection: Identifying and addressing deviations to prevent issues.
- Predictive Maintenance: Anticipating and scheduling maintenance to minimize downtime.
- Process Optimization: Improving efficiency through data-driven insights.
- Process Simulation: Testing and validating changes before implementation.
- Bottleneck Identification: Pinpointing and resolving inefficiencies.
- Resource Utilization: Enhancing the allocation and use of resources.
- Quality Improvement: Ensuring consistent product quality.
The benefits of digital twins are substantial, leading to increased productivity and efficiency, improved product quality, reduced downtime and maintenance costs, and enhanced decision-making capabilities. However, the adoption of digital twin technology also presents challenges, such as data management and security, model development and validation, integration with existing systems, and measuring return on investment (ROI).
Emphasize the Transformative Potential of Digital Twins in Manufacturing
Digital twin technology has the transformative potential to revolutionize manufacturing. By providing a comprehensive and real-time view of operations, digital twins enable manufacturers to achieve unprecedented levels of efficiency, precision, and agility. The integration of AI, machine learning, and augmented reality further enhances the capabilities of digital twins, making them indispensable tools for modern manufacturing.
As the technology continues to evolve, digital twins will play an increasingly critical role in optimizing supply chains, enhancing sustainability efforts, and driving innovation. The ability to simulate, predict, and optimize processes in real-time positions digital twins as a cornerstone of Industry 4.0, paving the way for smarter, more responsive, and more sustainable manufacturing practices.
Call to Action for Adoption and Further Research
The time to embrace digital twin technology is now. Manufacturers that adopt digital twins can gain a competitive edge by optimizing their operations, reducing costs, and improving product quality. However, successful implementation requires careful planning, investment in the right technologies, and a commitment to data security and ethical considerations.
Further research and development are essential to unlocking the full potential of digital twins. This includes advancing AI and machine learning algorithms, enhancing data integration and management techniques, and exploring new applications and use cases. Collaboration between industry, academia, and technology providers will be crucial in driving innovation and overcoming the challenges associated with digital twin adoption.