DeepMind Soundtracks: Transforming Videos with AI-Generated Audio
Table of Contents
Video generation models have made significant strides, but many still produce silent outputs. DeepMind Soundtracks is addressing this limitation with its innovative video-to-audio (V2A) technology, which uses video pixels and text prompts to generate rich soundtracks, enhancing the overall audiovisual experience. This article explores how DeepMind soundtracks are created, their applications, and the technological underpinnings that make this advancement possible.
Introduction to DeepMind Soundtracks
The Evolution of Video-to-Audio Technology
DeepMind has been at the forefront of artificial intelligence research, contributing transformative technologies across various fields. Their latest breakthrough, V2A technology, brings a new dimension to video generation by adding synchronized soundtracks, thereby breathing life into otherwise silent video content. This advancement promises to revolutionize the way sound is integrated with video, enhancing immersion and realism.
The Need for Automated Soundtracks
Traditionally, creating soundtracks for videos involves manual effort, requiring sound engineers, musicians, and voice actors to collaborate extensively. This process can be time-consuming and costly. DeepMind soundtracks offer a solution by automating the generation of audio, significantly reducing production time and cost while expanding creative possibilities.
How DeepMind Soundtracks Are Generated
The Core Technology Behind V2A
DeepMind’s V2A technology utilizes advanced machine-learning techniques to generate soundtracks. The system starts by analyzing the video’s visual content to extract relevant cues. It then uses these cues, along with optional natural language prompts, to create a synchronized audio track. The main components of this process include:
- Video Encoding: The video input is compressed into a representation that captures essential visual information. This encoding is crucial for the subsequent audio generation steps.
- Diffusion Model: A diffusion-based approach refines audio from random noise. This iterative process leverages visual input and text prompts to guide the generation of realistic soundscapes. The diffusion model has proven effective in creating compelling audio that aligns closely with the visual elements of the video.
- Audio Decoding: The refined audio is then decoded into an audio waveform, synchronized with the video. This ensures that the generated soundtrack matches the on-screen action and emotional tone.
Role of Text Prompts in Audio Generation
DeepMind soundtracks can be influenced by natural language text prompts, which provide additional context for the type of audio desired. This feature allows users to specify or exclude certain sounds, offering greater control over the generated output. For example:
- Positive Prompts: Direct the AI to include specific sounds, such as “dramatic orchestral music” or “ambient city noise.”
- Negative Prompts: Guide the AI to avoid certain sounds, like “no loud noises” or “exclude vocal sounds.”
This flexibility enables rapid experimentation with different soundtracks, allowing creators to choose the best match for their videos.
Applications of DeepMind Soundtracks
Enhancing Video Content Creation
DeepMind soundtracks can significantly enhance the quality of video content across various domains. Filmmakers, for example, can quickly generate music scores and sound effects that complement their visual narratives. Content creators on platforms like YouTube can use V2A technology to add professional-level soundtracks to their videos, enhancing viewer engagement and retention.
Revitalizing Traditional Footage
One of the exciting applications of DeepMind soundtracks is in revitalizing traditional footage, including archival material and silent films. By adding synchronized soundtracks, V2A can bring new life to old videos, making them more appealing to modern audiences. This capability opens up a wealth of creative opportunities for restoring and reimagining classic content.
Integrating with Video Generation Models
DeepMind soundtracks are designed to be pairable with video generation models, such as Veo. This integration allows for the creation of complete audiovisual experiences, where the generated video is accompanied by a dynamically generated soundtrack. The result is a cohesive and immersive media output that can be used in various applications, from entertainment to virtual reality.
Technical Insights into V2A Technology
Autoregressive vs. Diffusion Approaches
In developing V2A, DeepMind experimented with different AI architectures to find the most effective method for audio generation. While autoregressive models were considered, the diffusion-based approach emerged as the superior solution. This method iteratively refines audio from random noise, producing realistic and synchronized soundscapes that align with the visual input.
Training and Annotations
To enhance the quality of DeepMind soundtracks, the training process incorporated additional information, such as AI-generated annotations with detailed sound descriptions and transcripts of spoken dialogue. By learning from these annotations, V2A technology can associate specific audio events with visual scenes, improving the accuracy and relevance of the generated soundtracks.
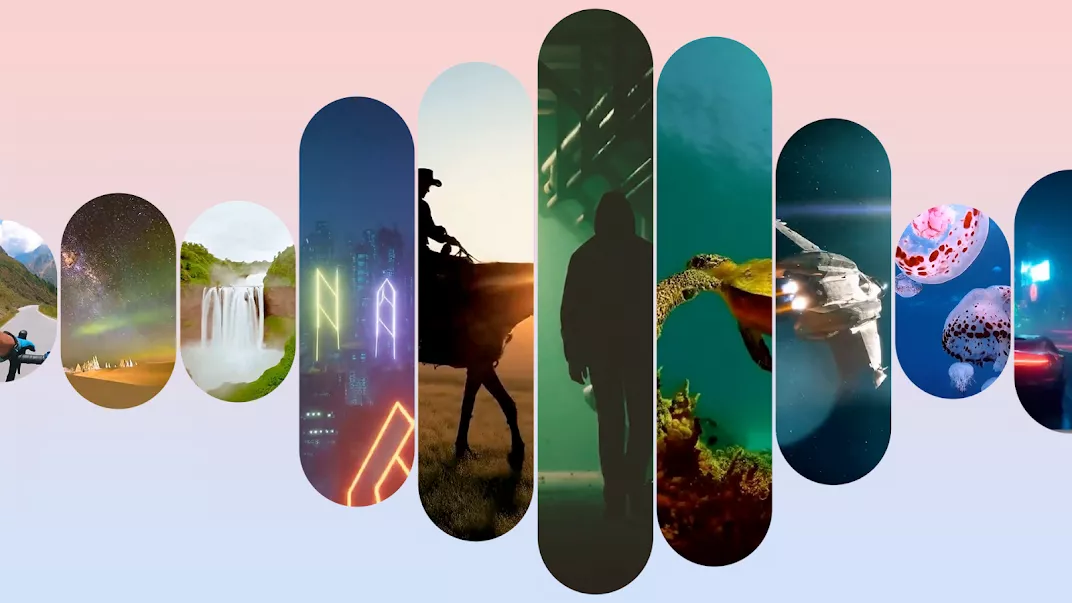
Addressing Lip Synchronization Challenges
Lip synchronization is a critical aspect of generating realistic dialogue for videos. V2A attempts to match generated speech with characters’ lip movements, but challenges arise when the video generation model does not align with the transcript. DeepMind continues to refine this aspect to achieve more natural and accurate lip-syncing, which is essential for creating believable character interactions.
Future Directions and Ethical Considerations
Ongoing Research and Development
DeepMind is committed to advancing V2A technology and addressing its current limitations. Research efforts focus on improving audio quality, especially in scenarios with suboptimal video input, and enhancing lip synchronization for videos involving speech. These improvements aim to make DeepMind soundtracks more robust and versatile across different types of video content.
Safety and Transparency
DeepMind ensures responsible development and deployment of V2A by incorporating feedback from the creative community and conducting rigorous safety assessments. The use of SynthID watermarking helps safeguard against the misuse of AI-generated content, promoting transparency and accountability.
Conclusion
DeepMind soundtracks represent a significant advancement in the field of video-to-audio technology, offering a powerful tool for generating synchronized soundscapes that enhance video content. By automating the creation of rich, realistic audio, V2A technology opens up new possibilities for filmmakers, content creators, and the entertainment industry. As research continues and technology evolves, DeepMind soundtracks are set to become an integral part of the future of audiovisual production, transforming how we create and experience media.
Featured Insights
The Impact of Data Analysis on Software Development
How a “Skeleton Key” Could Crack Open Your AI’s Secrets
Perplexity AI Search Content Scraping: Threatening the Open Web with Deception?
Supply Chain Management Services
Meta’s LLM Compiler: Amazing Game-Changer for Code Optimization?
Unveiling the LLM Native Alchemist’s Playbook: How to Craft Innovative AI Apps