Table of Contents
Google DeepMind, an artificial intelligence (AI) company, has ventured into the field of weather forecasting and has successfully developed a machine-learning model called GraphCast. This model has proven to outperform both traditional tools and other AI approaches in accurately predicting weather patterns. What sets GraphCast apart is its ability to make these predictions in a matter of minutes, as opposed to the hours it takes for conventional models.
Computer scientist Aditya Grover from the University of California, Los Angeles, states that GraphCast is currently leading the race among AI models.
The model’s capabilities are detailed in a publication in Science on 14 November. The task of weather prediction is known to be complex and resource-intensive. The standard method, known as numerical weather prediction (NWP), relies on mathematical models based on physical principles.
These models utilise data from buoys, satellites, and weather stations worldwide, and are processed by supercomputers. While these physical models accurately depict the movement of heat, air, and water vapour in the atmosphere, they are costly and require significant energy to operate.
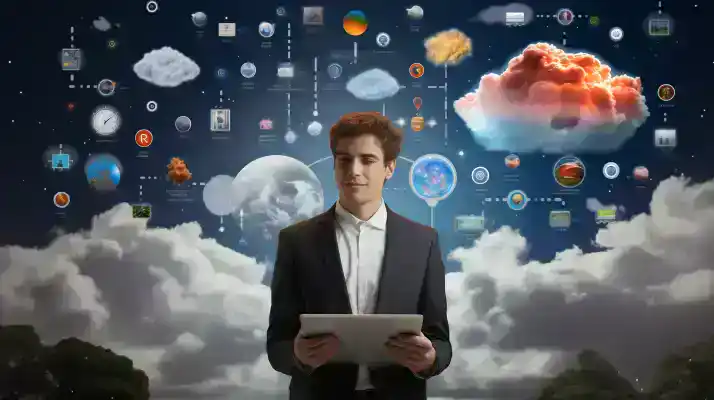
Deepmind AI’s Anticipated revolution
Several technology companies, including DeepMind, Nvidia, and Huawei, have developed machine-learning models that can quickly predict future global weather conditions based on past and current weather data. These models aim to reduce the financial and energy costs associated with forecasting. Among these companies, Huawei’s Pangu-weather model is considered a strong competitor to the gold-standard NWP system at the ECMWF in the UK, which provides leading weather predictions up to 15 days in advance.
Matthew Chantry from the ECMWF states that machine learning is revolutionizing weather forecasting, as AI models can run significantly faster than conventional models, allowing more time for interpretation and communication of predictions. GraphCast, developed by DeepMind, has shown superior performance in global weather forecasting tasks compared to both conventional and AI-based approaches.
The model was trained using estimates of past global weather and has learned the relationships between various weather variables. By utilizing the current state of global weather and estimates from 6 hours earlier, GraphCast can predict weather conditions 6 hours ahead. The model incorporates earlier predictions to make estimates further into the future.
DeepMind researchers have demonstrated that GraphCast can make accurate forecasts up to 10 days ahead in less than a minute using global weather estimates from 2018, outperforming the ECMWF’s High RESolution forecasting system.
Adverse climatic conditions
According to Remi Lam, a computer scientist at DeepMind in London, GraphCast has shown superior performance over HRES in over 99% of the 12,000 measurements conducted in the troposphere, which is the atmospheric layer closest to the surface and has the greatest impact on us. The model also outperformed HRES in 90% of weather predictions across all atmospheric levels.
According to Chantry, GraphCast was able to forecast the condition of 5 weather variables in close proximity to the Earth’s surface, including the air temperature 2-meters above the ground, as well as 6 atmospheric variables, such as wind speed, that are farther from the Earth’s surface. Additionally, it demonstrated its effectiveness in predicting severe weather occurrences, such as the routes taken by tropical cyclones, as well as extreme heat and cold spells.
The DeepMind researchers discovered that GraphCast outperformed Pangu-weather in forecasting ability, surpassing 99% of weather predictions previously described in a Huawei study. However, Chantry suggests that alternative metrics may yield varying results in future evaluations of GraphCast’s performance.
Dataset utilized for training
According to Chantry, machine-learning models have the potential to enhance specific types of weather prediction that traditional methods struggle with, rather than completely replacing them. For instance, these models could improve the accuracy of short-term rainfall forecasts. Chantry also notes that physical models are still necessary to provide the initial data for training machine-learning models. He predicts that it may take another two to five years before machine-learning approaches can be reliably used for real-world decision-making.
Meanwhile, it is crucial to address the issues associated with machine-learning methods. Unlike NWP models, the inner workings of AIs like GraphCast remain a mystery due to their decision-making processes occurring within an opaque ‘black box’, as stated by Grover. This lack of transparency raises concerns about their dependability, she adds.
Furthermore, AI models are susceptible to magnifying biases present in their training data and demand significant energy for training, albeit consuming less energy compared to NWP models, according to Grover.