Data Lake Mastery: Ignite Insights with 10 Proven Strategies
Table of Contents
In today’s data-driven landscape, businesses relentlessly pursue data utilization for informed decision-making and success. Amidst the array of available tools, data lakes stand out as potent reservoirs for managing and analyzing extensive datasets. This article embarks on an exploration of data lakes, delving into their essence and unveiling ten effective strategies to harness their potential.
From conceptual understanding to practical implementation, each strategy aims to empower organizations to unlock valuable insights from their data lakes. By embracing these strategies, businesses can navigate the complexities of data management, optimize analytical capabilities, and ultimately, thrive in an increasingly data-centric environment.
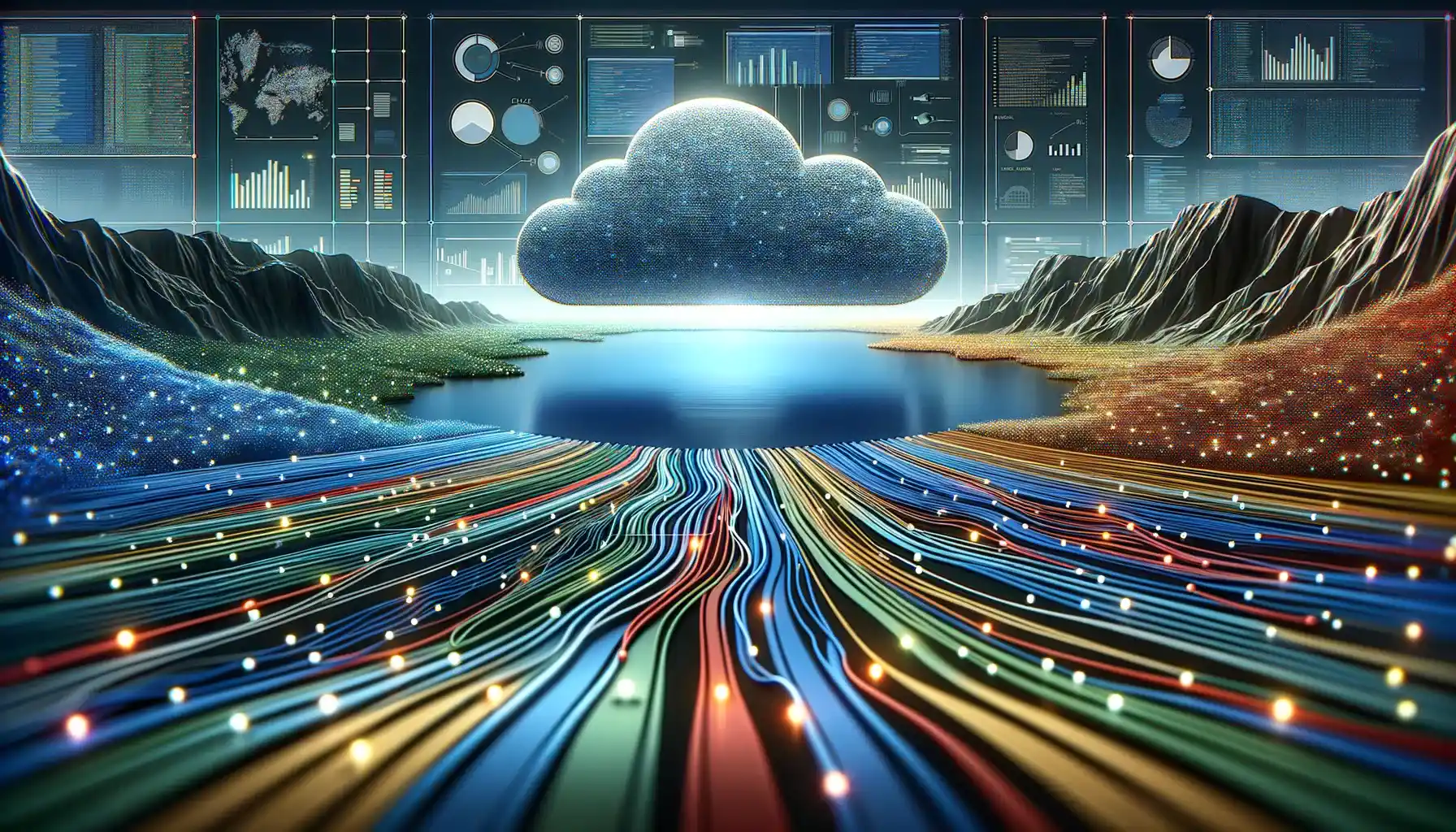
Understanding Data Lakes: A Deep Dive
Before exploring strategies for mastering data lakes, it’s essential to grasp their unique nature. Essentially, a data lake serves as a centralized reservoir where organizations can house vast amounts of data in various forms: structured, semi-structured, and unstructured.
Unlike conventional data warehouses, data lakes maintain data in its raw state, without imposing any predefined schema or format. This rawness preserves the original structure, fostering unparalleled flexibility in analysis and exploration. By retaining data in its raw form, organizations can avoid premature transformations, allowing for more agile and adaptive processing. Consequently, data lakes offer a versatile platform for extracting insights from diverse data sources, regardless of their inherent complexity or heterogeneity.
Distinguishing Data Lakes from Data Warehouses
Data lakes and data warehouses are both essential components of modern data management architectures, but they serve distinct purposes and exhibit contrasting characteristics. A data warehouse is a structured repository designed for storing, processing, and analyzing structured data derived from various operational systems.
It follows a schema-on-write approach, meaning data is organized into predefined schemas before being ingested into the warehouse. This ensures data consistency and facilitates high-performance querying but can limit flexibility and scalability.
In contrast, a data lake is a more flexible and scalable solution that stores data in its raw, unprocessed form, regardless of structure or format. Data lakes utilize a schema-on-read approach, allowing users to apply schemas and interpretations at the time of analysis.
This preserves data fidelity and enables organizations to store vast volumes of structured, semi-structured, and unstructured data without upfront transformation. While data lakes offer greater flexibility and agility for exploratory analysis and experimentation, they may require more rigorous governance and metadata management to maintain data quality and ensure usability.
1. Defining Clear Objectives
Before embarking on the journey of data lake implementation, it’s imperative to define clear objectives. These objectives act as guiding stars, directing efforts toward tangible outcomes. Whether the aim is to enhance decision-making processes, streamline operations for efficiency gains, or gain a competitive edge in the market, having well-defined objectives ensures that every step taken aligns with the overarching goals and priorities of the organization. By establishing clear objectives from the outset, stakeholders can better understand the purpose and potential impact of the data hub initiative, fostering alignment and commitment across the organization.
2. Designing an Effective Data Architecture
With objectives in place, the next step is to design an effective data architecture to support the data lake initiative. Building a robust data architecture lays the groundwork for successful data management and analysis. It involves designing a scalable and flexible framework capable of accommodating diverse data sources, formats, and types. By carefully planning the data architecture, organizations can ensure efficient data ingestion, storage, and retrieval within the data hub, facilitating seamless access to information for analysis and insights generation.
3. Implementing Data Governance Practices
Data governance plays a pivotal role in ensuring the quality, integrity, and security of data within the data lake. Implementing robust data governance practices involves establishing policies, procedures, and controls to govern the collection, usage, and management of data. This includes defining data standards, assigning data stewardship roles, and enforcing compliance with regulatory requirements such as GDPR or CCPA. By maintaining high standards of data governance, organizations can instill trust in the data and mitigate risks associated with data misuse or breaches, safeguarding the integrity and confidentiality of sensitive information.
4. Leveraging Advanced Analytics Techniques
One of the primary benefits of data lakes is their ability to support advanced data analytics techniques such as predictive modeling, machine learning, and artificial intelligence. By leveraging these techniques, organizations can extract valuable insights from the vast volumes of data stored within the data hub.
Predictive modeling enables organizations to forecast future trends and outcomes, while machine learning algorithms can identify patterns and anomalies that might otherwise go unnoticed. Artificial intelligence capabilities empower organizations to automate decision-making processes and deliver personalized recommendations to users, driving efficiency and innovation across the business.
5. Embracing Cloud-Native Solutions
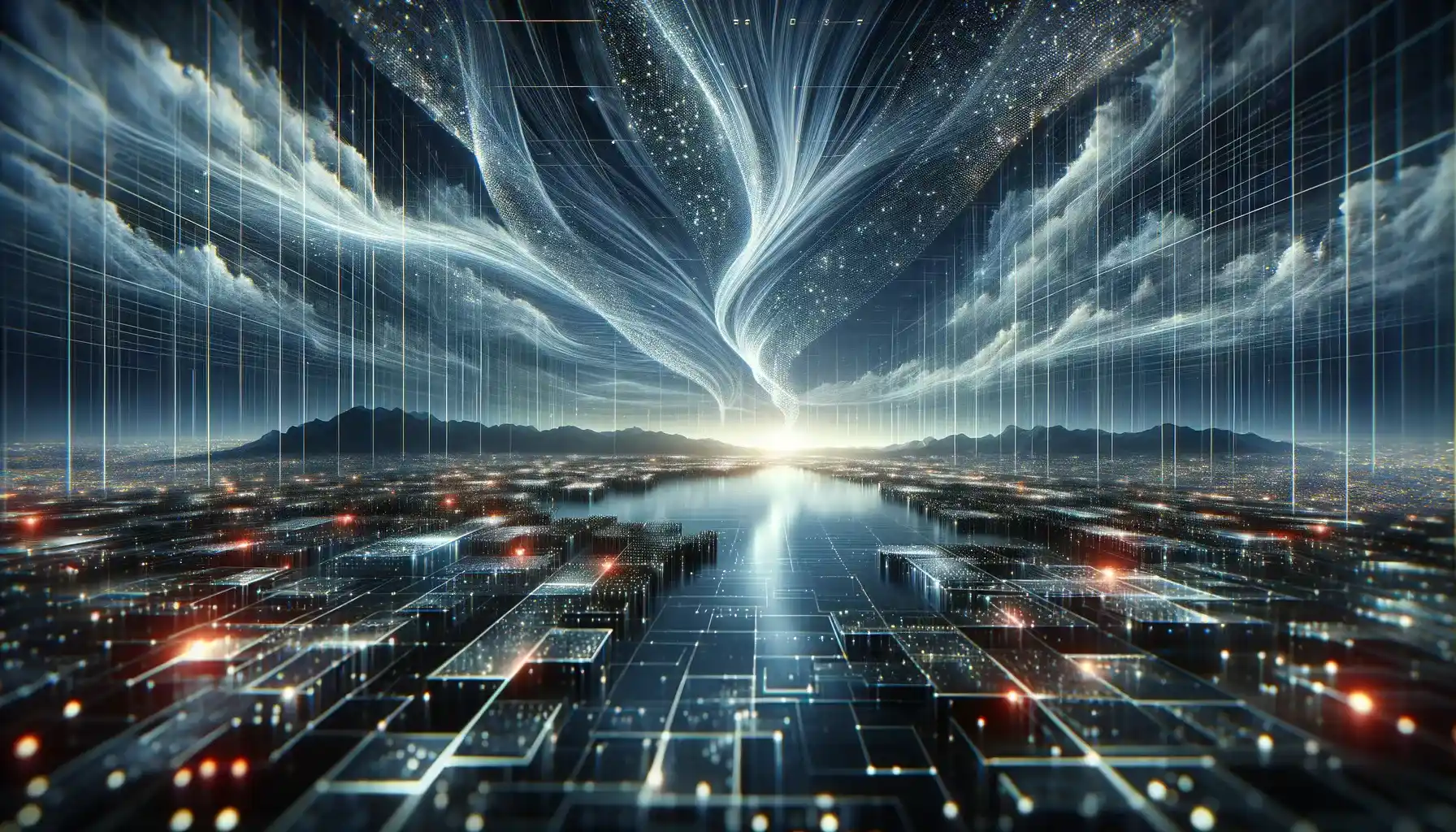
Cloud computing has revolutionized the way organizations manage and analyze data, offering scalability, agility, and cost-effectiveness. Embracing cloud-native solutions for data lakes allows organizations to harness the power of cloud infrastructure without the overhead of managing on-premises hardware and software.
Cloud-based data hub platforms such as AWS S3, Azure Data Lake Storage, or Google Cloud Storage provide scalable storage and computing resources, enabling organizations to store and analyze data at scale while only paying for what they use. By embracing cloud-native solutions, organizations can accelerate time-to-insight and drive greater agility in their data analytics initiatives.
6. Ensuring Data Security and Compliance
As custodians of vast amounts of sensitive information, data lakes must prioritize data security and compliance with regulatory requirements. Implementing robust security measures such as encryption, access controls, and auditing helps protect data from unauthorized access, ensuring its confidentiality and integrity.
Additionally, organizations must comply with data privacy regulations such as GDPR or CCPA to safeguard individual privacy rights and avoid costly penalties. By prioritizing data security and compliance, organizations can build trust with customers and stakeholders, strengthening their reputation and competitive position in the market.
7. Empowering Data Collaboration and Sharing
Data lakes serve as central repositories for data collaboration and sharing across the organization. By enabling seamless integration with data visualization tools, business intelligence platforms, and collaboration tools, organizations can empower data analysts, scientists, and business users to access and analyze data independently.
This fosters a data-driven culture where insights are democratized and innovation is encouraged. By promoting data collaboration and sharing, organizations can unlock the collective intelligence of their workforce, driving greater agility and competitiveness in the market.
8. Iterative Development and Continuous Improvement
Mastering data lakes is an iterative process that requires continuous development and improvement. By adopting agile methodologies and DevOps practices, organizations can accelerate the development and deployment of data hub solutions while also fostering a culture of experimentation and learning.
Iterative development allows organizations to quickly iterate on ideas, gather feedback, and incorporate learning into subsequent iterations. Continuous improvement ensures that data lake solutions evolve to meet changing business needs and technological advancements, driving innovation and value creation over time.
9. Monitoring and Performance Optimization
Monitoring the performance of the data lake infrastructure is essential for ensuring reliability, scalability, and efficiency. By leveraging monitoring tools and metrics, organizations can proactively identify bottlenecks, optimize resource utilization, and ensure seamless operation of the data hub environment.
Performance optimization measures such as data partitioning, indexing, and caching can help improve query performance and reduce latency, enhancing the overall user experience. By continuously monitoring and optimizing data lake performance, organizations can ensure that it remains a reliable and scalable platform for data management and analysis.
10. Driving Business Value and ROI
Ultimately, the success of a data lake initiative hinges on its ability to drive tangible business value and deliver a positive return on investment (ROI). By aligning data hub outcomes with business objectives and measuring key performance indicators (KPIs), organizations can demonstrate the value of data-driven insights to stakeholders.
Whether it’s improving operational efficiency, enhancing customer experiences, or identifying new revenue opportunities, data lakes can help organizations achieve their strategic goals and drive sustainable growth. By focusing on driving business value and ROI, organizations can secure ongoing support and investment for their data hub initiatives, ensuring their long-term success and impact on the business.
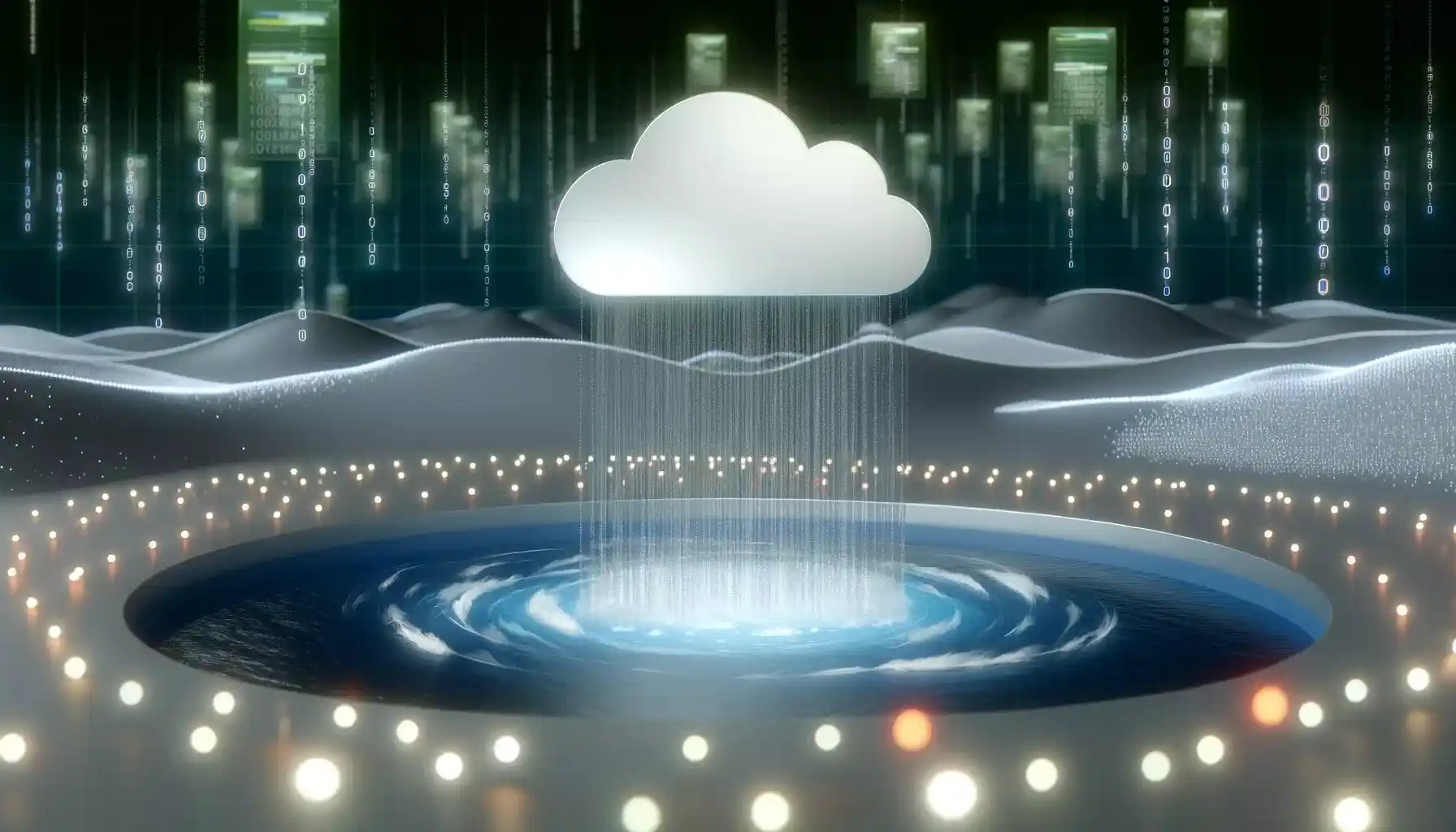
Conclusion to Mastering the Data Lake
To truly master data lakes, organizations must adopt a comprehensive approach, integrating strategic planning, technical prowess, and organizational cohesion. The outlined ten strategies serve as guiding principles, enabling organizations to extract maximum value from their data hubs. By adhering to these strategies, businesses can unleash a wealth of insights, propelling them toward sustainable success in the modern data-centric landscape.
Embracing the capabilities of data lakes empowers organizations to navigate complex data landscapes with confidence, facilitating informed decision-making and fostering a culture of data-driven excellence. Embrace the transformative potential of data hubs and embark on a journey toward unlocking unparalleled opportunities for innovation and growth.
Featured Insights
The Future is Now: AI and ML Technologies Reshaping Healthcare
Introduction to Digital Twin Technology in Manufacturing with Unprecedented Efficiency and Innovation
Adobe’s Firefly AI: New Tools for Photoshop and Illustrator
Introducing Meta Imagine Me: Your Digital Doppelganger
AI in Gaming is Transforming the Industry
Gen AI Market Boom: A $1 Trillion Industry in the Making?