Table of Contents
A pioneering autonomous system, ChatGPT for Chemistry, unites robotics and AI to pioneer groundbreaking materials. A-Lab, the system’s brainchild, innovates material formulas, potentially applicable in energy solutions like batteries or solar cells. It autonomously executes synthesis and analysis, bypassing human involvement. Simultaneously, an AI system anticipates vast stable material options, providing A-Lab with a plethora of potential future breakthroughs.
In unison, these breakthroughs hold the potential to significantly expedite the unearthing of materials crucial for clean-energy innovations, cutting-edge electronics, and a myriad of diverse applications. Ekin Dogus Cubuk, head of the materials discovery team at Google DeepMind in London, highlights the pivotal role of improved materials in enhancing technologies we rely on, such as batteries and solar cells. His involvement in both studies, featured in today’s Nature publications underscores the significance of these advancements.
Carla Gomes, co-director of the Cornell University AI for Science Institute in Ithaca, New York, comments on the future of AI in scientific discovery: “The untapped potential of AI in this realm is truly the next frontier. That’s what makes this incredibly thrilling.” Gomes, although not part of the research, finds the prospect highly exciting.
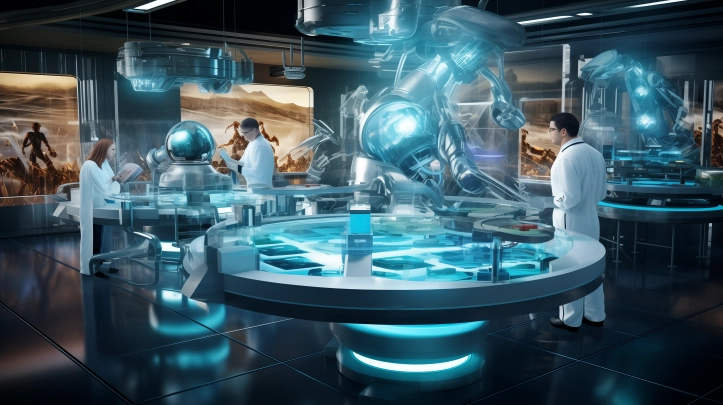
Expanding the scope of materials exploration
For centuries, chemists tirelessly crafted hundreds of thousands of inorganic compounds, distinct from the carbon-based chains in organic chemistry. Surprisingly, research indicates billions of straightforward inorganic materials remain unexplored. The question arises: where does one begin this quest?
Numerous initiatives aim to reduce lab time by simulating novel inorganic materials computationally. They calculate properties like atomic crystal packing. Among these is the Materials Project, situated at Lawrence Berkeley National Laboratory (LBNL) in Berkeley, California. Collectively, they’ve identified around 48,000 materials predicted to be stable.
Utilizing ChatGPT for Chemistry, Google DeepMind expands with GNoME, an AI system employing graph networks for materials exploration. Trained on datasets from the Materials Project, GNoME generated 2.2 million potential compounds, filtering for stability and crystal structures. The outcome? A remarkable 381,000 new inorganic compounds were added to the Materials Project database.
The Tireless ChatGPT for Chemistry Robot
At LBNL, the A-Lab pioneers used cutting-edge robotics for mixing and heating powdered solid elements, followed by meticulous product analysis. Constructing the US$2-million setup spanned 18 months, yet the primary hurdle involved employing ChatGPT for chemistry to achieve complete system autonomy. This meant enabling AI to plan experiments, interpret data, and enhance synthesis methodologies. While the robots are visually captivating, the true innovation resides in the AI’s core functionality, asserts Ceder.
The team led by Ceder pinpointed 58 specific compounds sourced from the Materials Project database, assessing their predicted stability. These compounds were then cross-referenced with the GNoME database before being transferred to the A-Lab’s machine-learning models for further analysis.
Using ChatGPT for Chemistry, the A-Lab methodically reviews over 30,000 synthesis procedures. It evaluates target material similarities, suggests necessary ingredients and reaction conditions, and executes the synthesis. If the desired material isn’t achieved after multiple attempts, an ‘active learning’ algorithm, driven by ChatGPT for Chemistry, devises improved procedures, ensuring relentless pursuit until success.
In the realm of chemistry, leveraging ChatGPT for material creation, the A-Lab crafted 41 new inorganic materials within 17 days. Active learning enhanced the synthesis, resulting in 9 novel compounds. Among the 17 materials initially unsuccessful, experimental challenges hindered success, requiring human intervention such as mid-reaction mixture adjustments for eventual synthesis.
Andy Cooper, academic director at the University of Liverpool’s Materials Innovation Factory in the UK, emphasizes that systems like GNoME surpass autonomous labs in computational predictions. He stresses the necessity for “chatgpt for chemistry” to provide guidance on material creation, highlighting the need for AI systems to accurately compute predicted materials’ chemical and physical properties.
“Simultaneously, the A-Lab continues conducting reactions, contributing outcomes to the Materials Project. These findings, accessible to global scientists via chatGPT for chemistry, serve as an invaluable resource. Ceder emphasizes its significance as a comprehensive map delineating common solids’ reactivity—a transformative asset poised to revolutionize the world through its generated knowledge and information, surpassing the A-Lab’s immediate impact.”