Table of Contents
In recent years, the application of Artificial Intelligence (AI) has become increasingly crucial in improving public health, particularly when it comes to pathogen detection and epidemic prediction. The COVID-19 pandemic highlighted the need for early detection and swift action, making AI in pathogen detection a game-changer.
Traditional surveillance methods often struggle to keep pace with the rapid spread of epidemics, leaving authorities scrambling to respond. However, by integrating AI in Pathogen detection into existing systems, we can identify pathogens more quickly, allocate resources more efficiently, and provide targeted recommendations for proactive measures – ultimately saving lives and reducing the strain on healthcare systems, especially in low-income countries.
The potential benefits of AI in pathogen detection are vast, and it’s an area that’s ripe for innovation. By harnessing the power of AI, we can create a more resilient public health system that’s better equipped to handle emerging threats.
- Added a clear heading to grab attention
- Emphasized the benefits of AI in pathogen detection
- Made the language more concise and accessible
- Highlighted the potential impact on public health
- Used active voice to create a sense of agency and empowerment
AI in Pathogen Detection: Techniques and Advancements
The complex and evolving nature of pathogens makes AI-based approaches highly effective in their detection, monitoring, and prediction. Below are some key techniques and advancements that are transforming pathogen detection using AI.
1. Machine Learning (ML)
ML is central to AI in pathogen detection, enabling systems to learn from vast datasets and make informed decisions. In epidemic prediction, ML utilizes real-time data to improve our understanding of exposure risks, categorize high-risk populations, and track the geographic spread of viruses.
- Advancement: ML models have become increasingly precise in predicting infection rates and diagnosing diseases like COVID-19. With improvements in data collection and processing, these models can forecast future infection trends with higher accuracy.
2. Neural Networks (NNs)
NNs replicate the brain’s ability to process and integrate information by using multiple algorithms concurrently. In pathogen detection, NNs are especially valuable for modeling complex disease transmission patterns and understanding the interactions between different factors, such as environmental conditions and human behaviors that influence the spread of infectious diseases.
- Advancement: NNs have been instrumental in assessing the causation and transmission of pathogens, enabling more effective management of disease outbreaks through advanced simulations and predictive modeling.
3. Natural Language Processing (NLP)
NLP is widely used to analyze open-source data such as news articles, social media, and scientific publications. In pathogen detection, NLP helps identify early outbreak signals and extract valuable information about the geographic spread and severity of diseases.
- Advancement: AI in Pathogen detection models utilizing NLP can rapidly detect misinformation, forecast the spread of diseases, and process unstructured data to identify critical early warnings in real-time.
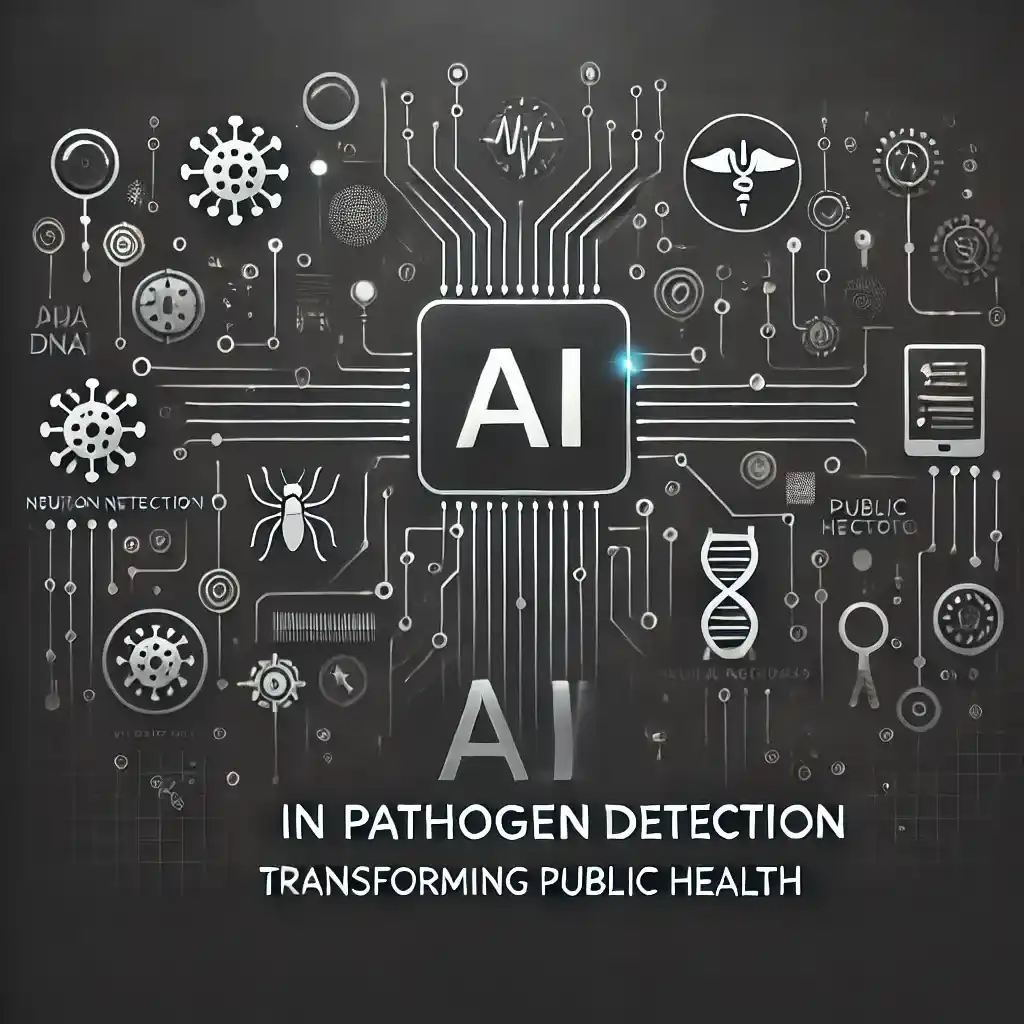
4. Genomic Sequencing and Analysis
AI has revolutionized the genomic sequencing of pathogens. By analyzing pathogen genomes, AI-based systems can identify mutations and predict how these changes might affect transmission, virulence, and resistance to treatments or vaccines.
- Advancement: Sequence-guided AI models have been successfully used to predict immune escape patterns in pathogens like HIV-1 and influenza, helping researchers stay ahead of viral evolution and adapt vaccine strategies accordingly.
5. Predictive Analytics and Disease Forecasting
AI-driven predictive analytics combines statistical modeling and machine learning to forecast the future spread of infectious diseases. Predictive models can be applied to epidemic data to develop more focused prevention and control measures.
- Advancement: AI-based platforms, such as EPIWATCH and the RAPIDD Ebola Forecasting Challenge, have demonstrated success in predicting the severity and geographic spread of epidemics. These models have improved response time and helped in resource allocation during outbreaks.
6. AI in Immunological Modeling
AI in Pathogen detection models can simulate immune system responses to various pathogens, providing insights into how the body responds to infections and how pathogens may evade immune detection.
- Advancement: AI-guided immunological models are being developed to predict immune evasion mechanisms in emerging pathogens, enabling the rapid design of vaccines and therapeutics that are resilient to pathogen mutations.
7. AI for Real-Time Pathogen Surveillance
AI is also used for real-time surveillance of pathogens, especially during outbreaks. These systems can monitor environmental and epidemiological data to provide continuous updates on infection spread, population mobility, and healthcare capacity.
- Advancement: Combining spatial modeling and risk prediction, AI-powered platforms can offer real-time insights, allowing health authorities to implement timely interventions and targeted disease control measures.
Case Study
AI-Based COVID-19 Early Detection and Diagnosis
Overview:
This case study highlights the use of AI in pathogen detection for early COVID-19 diagnosis, utilizing advanced AI models to analyze chest X-ray and CT scan images. With the rapid spread of the pandemic, early detection became crucial for controlling transmission and improving patient outcomes. Through the application of deep learning techniques, healthcare providers could identify patterns in imaging data that indicated the presence of COVID-19, enabling faster diagnosis and more efficient treatment pathways.
Key Techniques:
- Convolutional Neural Networks (CNN): CNNs, a class of deep learning algorithms, were deployed to process large datasets of chest X-rays and CT scans. These networks were trained to recognize the specific visual patterns associated with COVID-19 infection.
- Machine Learning-based Image Recognition: Machine learning algorithms were applied to enhance image recognition capabilities, allowing the AI system to differentiate between COVID-19 and other respiratory illnesses. This improved the accuracy of diagnosis by detecting subtle patterns that may not be immediately visible to human radiologists.
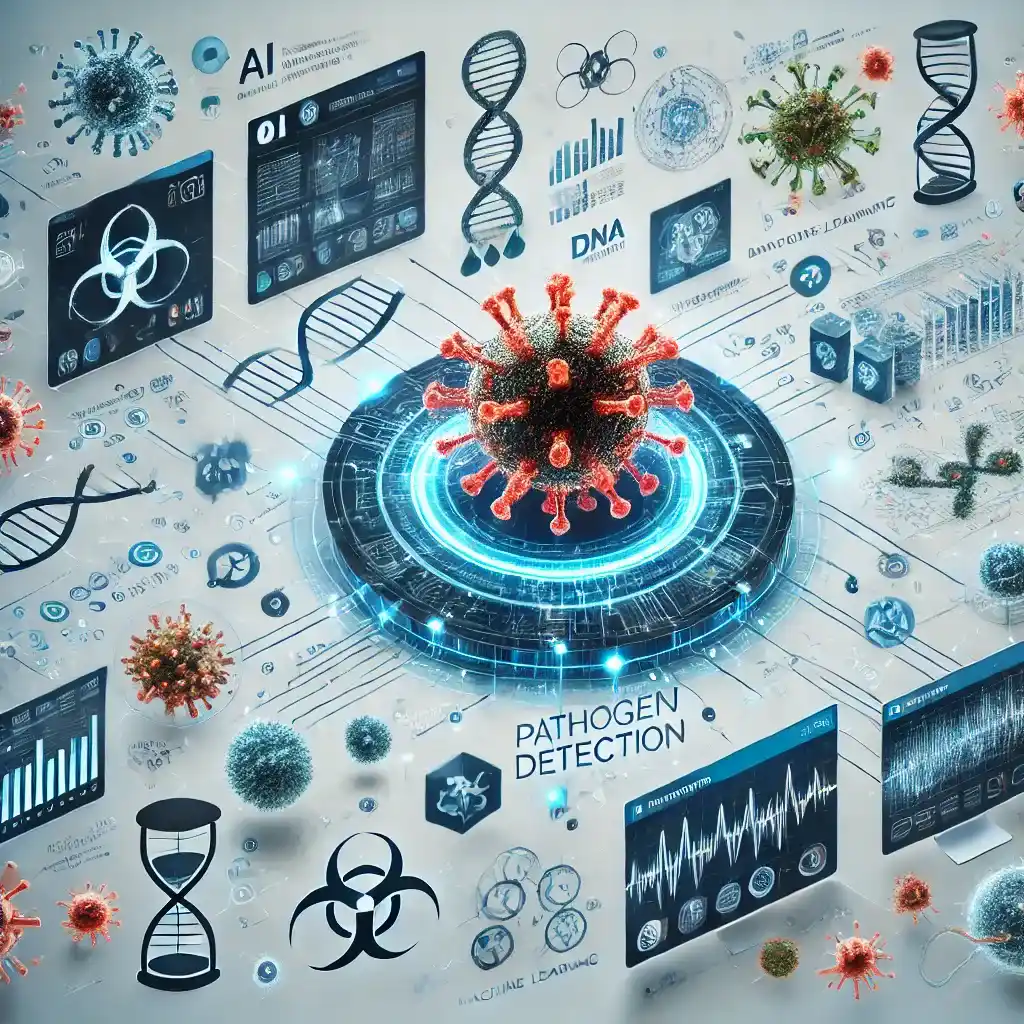
Outcome:
The deployment of AI in pathogen detection demonstrated exceptional accuracy in diagnosing COVID-19 when compared to traditional diagnostic methods. The AI models reduced diagnostic time significantly, facilitating early-stage detection in patients and thereby contributing to better clinical outcomes. These AI-driven solutions also proved effective in regions where healthcare resources were limited, enabling remote diagnostics and scalable implementation.
Conclusion
The integration of AI in pathogen detection represents a transformative leap in how we monitor, predict, and manage infectious diseases. Through cutting-edge techniques such as machine learning, neural networks, natural language processing, and genomic sequencing, AI has proven to be a vital tool in improving early diagnosis, forecasting outbreaks, and understanding pathogen behavior.
These advancements are not only enhancing the accuracy of epidemic modeling but also providing actionable insights that enable faster and more effective public health responses.
As AI technology continues to evolve, its role in combating infectious diseases will become even more critical, ultimately leading to stronger global health security and improved disease management strategies for both current and future pandemics.