Table of Contents
The sustained care needs of chronic diseases are stretching the system to breaking point. Two thirds of all US deaths are caused by five diseases, according to the National Institutes of Health: heart disease, cancer, stroke, chronic obstructive pulmonary disease, and diabetes. Additionally, the Healthcare workforce is shrinking with over 400,000 employees having already left the industry due to the covid-19 incidents. This trend is expected to persist, putting more strain on the remaining workforce.
However, Artificial Intelligence AI offers a beacon of hope, beginning with the crucial first step in effective care: diagnosis.
AI in Healthcare Diagnostics and Clinical Outcomes
Another reason for imaging and diagnostics errors can be understaffed workforces and high caseloads. According to the Society to Improve Diagnosis in Medicine, health cure errors affect more than 12 million Americans annually. These errors contribute to inaccurate treatment decisions and cost more than $100 billion annually. AI has already solved this problem since it can go through reports much faster and more accurately than people. Moreover, in several situations, AI could diagnose diseases that were impossible to determine for people.
The key to effective diagnosis lies in high-quality visual data and robust machine learning and computer vision algorithms. Trained on high-quality data, these algorithms can identify complex patterns within patient samples, leading to better condition prediction and more insightful comparisons. They can process various types of medical imagery, from MRIs and X-rays to ultrasounds, detecting subtle details that might escape human observation.
This capability is particularly useful for chronic conditions, which require accurate initial detection for long-term care. An AI system can analyze data and produce diagnoses faster than a human, potentially informing a patient about a tumor within days instead of weeks. This speed allows for immediate treatment commencement, possibly leading to improved clinical outcomes.
For healthcare providers, AI’s speed and accuracy can alleviate staff workload, enabling better quality care. An AI model can work continuously, delivering high-quality results for all samples without taking a break or showing bias (if trained well). This level of performance may not be achievable by humans who can suffer from fatigue and tiredness over time and make incorrect decisions based on their interpretations of patient reports.
Moreover, AI in health care accessibility in underserved populations and regions where skilled staff might be scarce. The models could automate tasks and reduce clinical workloads, enabling non-specialists to undertake complex duties like cardiac imaging and analysis. This capability can also bring healthcare to patients’ homes and smaller clinical settings.
AI’s Potential Across Various Domains
Despite skepticism about AI’s potential in healthcare, visual data-driven AI diagnostics are already significantly impacting different medical settings. For example, AI can assist in detecting abnormal findings on radiographs, particularly the improper positioning of catheters and tubes, which can lead to severe complications.
In busy clinical practices, there may be delays before a radiologist can thoroughly assess X-ray images. However, integrating computer vision as a second pair of eyes can be beneficial. It enables the prompt detection of misplacements, allowing for immediate attention and prioritization. Recently, AI models have shown high accuracy in detecting and locating catheters on radiographs, expediting the identification of critical issues, leading to timely interventions, and improving patient outcomes.
Furthermore, AI is addressing the diagnostic challenges posed by brain tumors, where the variability of tumor shapes and sizes necessitates early detection. Cutting-edge machine learning algorithms have shown promise in speeding up tumor localization, with various models trained to segment tumors in MRI scans demonstrating impressive accuracy levels.
Even when these tools are not perfect, they enhance doctors’ decision-making processes, leading to more timely and precise interventions for patients. Similar results have been found in AI-driven diagnosis of skin cancer, the most widespread oncology disease in the US and one that is challenging to detect due to the inherent variability of skin lesions. A 2022 research paper by set, published in Frontiers, highlighted the success of AI in this domain, demonstrating that machine learning models outperformed the average capabilities of dermatologists and could assist in the early detection of skin cancers.
The Road Ahead for AI in Healthcare Diagnostics
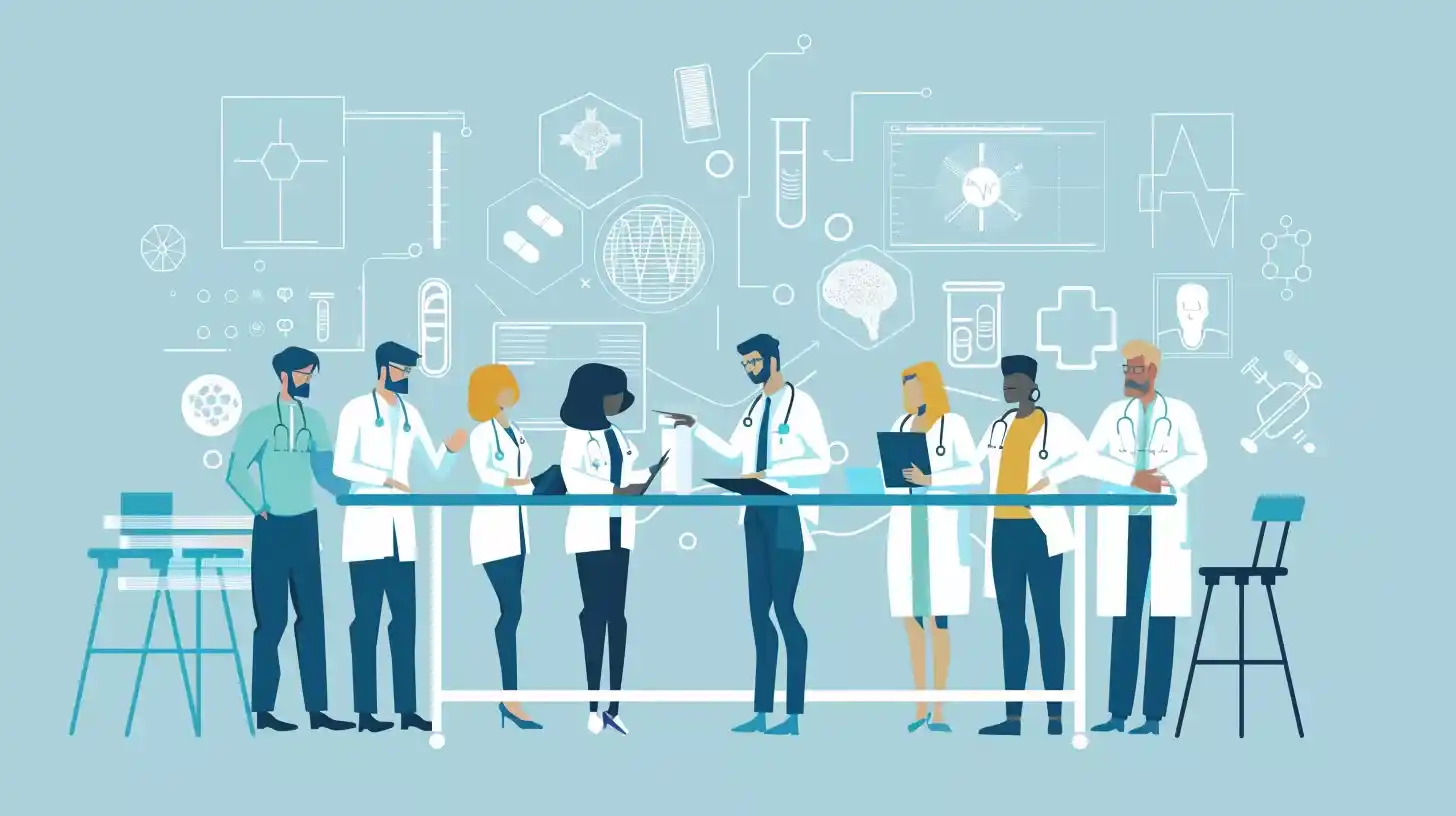
Even with such limitless potential, the successful implementation of AI and machine learning into healthcare diagnostics is fraught with issues. Healthcare providers likely will not start integrating these groundbreaking technologies until they are unquestionably assured of their performance in all types of clinical environments and 100% reliant on this model.
Policymakers can address this by proactively supporting the evaluation of machine learning diagnostic technologies across real-world scenarios, while simultaneously striving to expand access to medical data and foster collaboration among developers, providers, and regulators. Their work can lead to better models, expanded testing, and increased confidence among technology adopters.
Another challenge in adopting AI in healthcare diagnostics is the availability of high-quality training data. All teams need good quality medical data to train their AI tools/models, but obtaining it involves navigating a complex environment of ethical and regulatory restrictions. This process can be difficult and time-consuming
As a solution, Thus, data cleanrooms such as Snowflake provided, or public datasets should be used. However, even this may require some level of work to deal with issues like bias.
None of this is guaranteed; however, as it remains to be seen how much adoption of AI in healthcare diagnostics will expand in the next years. But one thing stands out in this healthcare revolution: the union of AI and computer vision. From transforming patient care to saving lives to alleviating the financial burden on the healthcare systems, the power of utilizing data and AI has already proven to be highly efficient. All stakeholders, from policymakers to healthcare professionals and technology developers, should gather and join the journey toward fully embracing it and facing new and amazing challenges.