AI in Agriculture: The Amazing Benefits for Farmers and the Environment
Table of Contents
AI in agriculture is not a new concept, but it is becoming more advanced and widespread as technology evolves. For thousands of years, farmers have relied on their intuition and experience to decide what to grow, where to grow, and how to grow. But with the challenges of climate change, population growth, and food security, farmers need more data and insights to optimize their production and efficiency.
AI in agriculture can provide farmers with powerful tools to analyze large amounts of data, detect patterns, and make predictions. AI can help farmers forecast weather, soil, and market conditions, and choose the best crops and practices for their specific locations.
AI can also help farmers monitor and manage their crops, using computer vision and machine learning to identify weeds, pests, diseases, and nutrient deficiencies. AI in Agriculture can also help farmers reduce their environmental impact, by applying inputs such as water, fertilizers, and pesticides more precisely and sparingly.
AI in agriculture is already making a difference for farmers around the world. Here are some examples of how AI is transforming the future of farming:
AI for climate-risk modeling
ClimateAI is a company that uses AI to help customers assess and improve their resilience to climate change. Will Kletter, the vice president of operations and strategy at ClimateAI, says that AI can provide “more accurate but also more localized” forecasts of climate trends. This can help agricultural investors to select sites where particular crops will grow well, or decide which crops are best suited to specific sites.
Kletter says that his clients often arrive at the same conclusions as the AI in Agriculture models but take far longer to collect and analyze data. “We’re helping customers make faster decisions, with less waste, that ultimately helps them bring maybe a new seed variety or new food crop to market more quickly,” he claims.
However, Kletter also advises farmers to use AI in Agriculture selectively, to solve specific problems. Given the economics of farming, “there isn’t a lot of excess profit to go throwing at cool ideas. They have to be really useful ideas.”
AI in Agriculture for precision spraying
Several companies have developed technology that combines high-precision cameras with machine learning algorithms that can rapidly analyze and act on the data that “computer vision” provides.
One of them is Blue River Technology, a company that was acquired by agricultural machinery giant John Deere in 2017. Blue River has developed technology that attaches cameras to crop sprayers. The camera is trained by AI to recognize weeds, meaning that the sprayer applies herbicides to a targeted area, rather than an entire field.
Blue River’s chief executive, Willy Pell, notes that farmers who intensively care for every plant on their farm can, in theory, achieve higher yields with lower inputs. The problem, he says, is this kind of intensive plant care is impossible to practice on a large farm without a vast army of laborers.
AI-based targeted spraying technology offers the possibility that farmers can “scale this micromanagement with machines that can perceive and act in real-time”, Pell says. He predicts that targeted spraying technology will become widespread, especially on large-scale farms, within the next decade.
Another company that has developed precision spraying technology is Greeneye Technology, an Israeli ag-tech startup. Its chief executive, Nadav Bocher, believes the environmental benefits will prompt regulators to incentivize farmers to adopt those technologies.
AI for crop breeding and disease detection
AI in agriculture can also play a supportive role, especially in developing countries, where millions of small-scale farmers lack access to resources and information. AI can help farmers improve their crop quality and resilience, by providing them with advice and alerts.
One example is Tumaini, a smartphone app developed by Michael Selvaraj, a senior scientist in digital agriculture at the International Centre for Tropical Agriculture. Tumaini allows banana growers to scan plants for signs of diseases and pests. The app also provides advice to farmers, including on selecting disease-resistant crop varieties that are suited to their regions.
Selvaraj adds that the app can provide an “early warning system”. Farmers scan plants on their smartphones; if this detects the presence of disease, the app can alert policymakers and support risk-mapping and epidemiological surveys.
Another example is the Artemis Project, designed by Selvaraj’s colleague, agricultural scientist David Guerena, in Tanzania. This uses an app to help with phenotyping, the process of breeding new crop varieties based on observations of plant characteristics.
“Usually, it takes about 10 years to develop a new variety of plant,” says Guerena. “Climate change is happening so fast now that 10 years is not fast enough. Breeders need to speed up this process to make sure that the varieties that are coming out are climate resilient.”
He explains that the basic approach to breeding new crop varieties, which involves humans attempting to cross plants with promising characteristics, has “remained unchanged for 10,000 years”. This is an inefficient process, involving a great deal of trial and error, he says.
“We’re looking at basically replacing what breeders are doing now with computer vision-enabled models,” says Guerena. Plant growers participating in the Artemis Project collect data by taking photos through the app. This data is then analyzed by AI-powered models to help select plant genes that are best adapted to specific locations and will be more resilient to forecasted climatic changes.
AI in Agriculture for soil carbon management
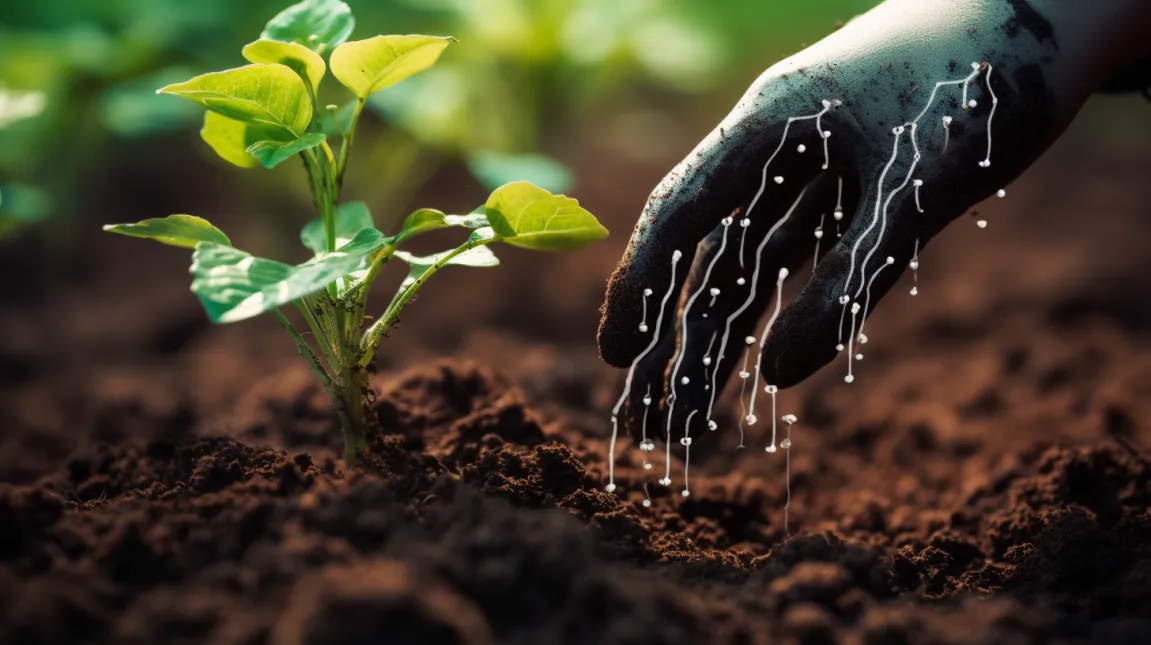
AI in agriculture can also help estimate the level of carbon stored in soils, which is crucial for mitigating climate change. Soils contain around 75% of all carbon stored on land, according to the Ecological Society of America. Soils sequester carbon mainly through organic matter such as decomposing plants and animals. Carbon can potentially remain in soils for thousands of years. However, common agricultural practices, including plowing, rotating crops, and applying fertilizers can release carbon into the atmosphere.
The first step towards managing soil carbon stocks is accurate measurement and monitoring. This, however, is far from easy, says Martha Farella, a data scientist at engineering and environmental consultancy Stantec. “Quantifying soil carbon over any given area of space is quite difficult,” she says, with climatic conditions, topographic factors, and vegetation types, along with the soil’s porosity and texture, all affecting the amount of carbon that can be stored. “There’s a lot of uncertainty that surrounds soil carbon because of that.”
The standard way to measure soil health involves ground sampling. But this is a time-consuming and costly process, that can realistically only be undertaken occasionally at a small number of locations. Farella explains that using remote sensing, combined with machine learning models that provide a picture of how certain factors influence soil health, can be a more effective approach at scale.
“We can look at climatic patterns over space, we can look at vegetation properties over space, we can look at various soil factors to some degree over space,” she tells us. “And then we can use (machine learning) to build models based on known soil carbon values and those remote sensing variables to then predict soil carbon across space for all of the areas that we don’t have direct measurements for.”
One company that uses remote sensing and machine learning techniques to provide agricultural landowners with a “digital twin” of their properties is Downforce Technologies. Its head of Asia-Pacific, Ben Wark, says that his company is generating huge interest from landowners who want to “understand and quantify the current sequestration that they have within their properties”.
Downforce’s platform is designed to allow landowners to establish a baseline of soil carbon and then monitor how changes to land-management practices affect the level of carbon sequestration.
Downforce can use this approach “to create carbon credits that can be used for insetting within businesses,” says Wark.
Having better and more timely data will ultimately help agricultural landowners to manage soil health more effectively, Ward argues. “We want to demonstrate that understanding what you’ve done in the past that was good or bad, and understanding what works where will help you to be able to create better soil health and in turn increase soil carbon.”
AI in agriculture is a game-changer for farmers, investors, and the environment. By harnessing the power of data, AI can help farmers improve crop yields, reduce inputs, and enhance soil health. AI in Agriculture can also help farmers adapt to the changing climate and market conditions, and contribute to the global efforts to reduce greenhouse gas emissions. AI in agriculture is transforming the future of farming.
Featured Insights
Integrating Real-Time Weather Data into Your Travel and Navigation App
The Future is Now: AI and ML Technologies Reshaping Healthcare
Introduction to Digital Twin Technology in Manufacturing with Unprecedented Efficiency and Innovation
Adobe’s Firefly AI: New Tools for Photoshop and Illustrator
Introducing Meta Imagine Me: Your Digital Doppelganger
AI in Gaming is Transforming the Industry