Table of Contents
AI Evolution: In an era where artificial intelligence (AI) is evolving at an unprecedented rate, traditional evaluation methods are being pushed to their limits. This surge in AI capabilities, particularly after the groundbreaking release of OpenAI’s ChatGPT in 2022, has ignited a technological race. Major players such as Microsoft, Google, and Amazon are pouring tens of billions into the development of AI, accelerating progress beyond the capabilities of existing benchmarks.
The Obsolescence of Current Benchmarks
Aidan Gomez, the CEO of AI startup Cohere, notes the fleeting relevance of public benchmarks, which now become obsolete in months as opposed to the years it previously took. This rapid obsolescence is attributed to the advancements in AI models, which now quickly surpass the established criteria designed to assess their performance, accuracy, and safety.
The deployment of new AI models by companies like Google, Anthropic, Cohere, and Mistral highlights the intense competition in the field, all vying for supremacy in the domain of large language models (LLMs). These LLMs form the backbone of systems such as ChatGPT, demonstrating abilities that significantly exceed the parameters of existing evaluations.
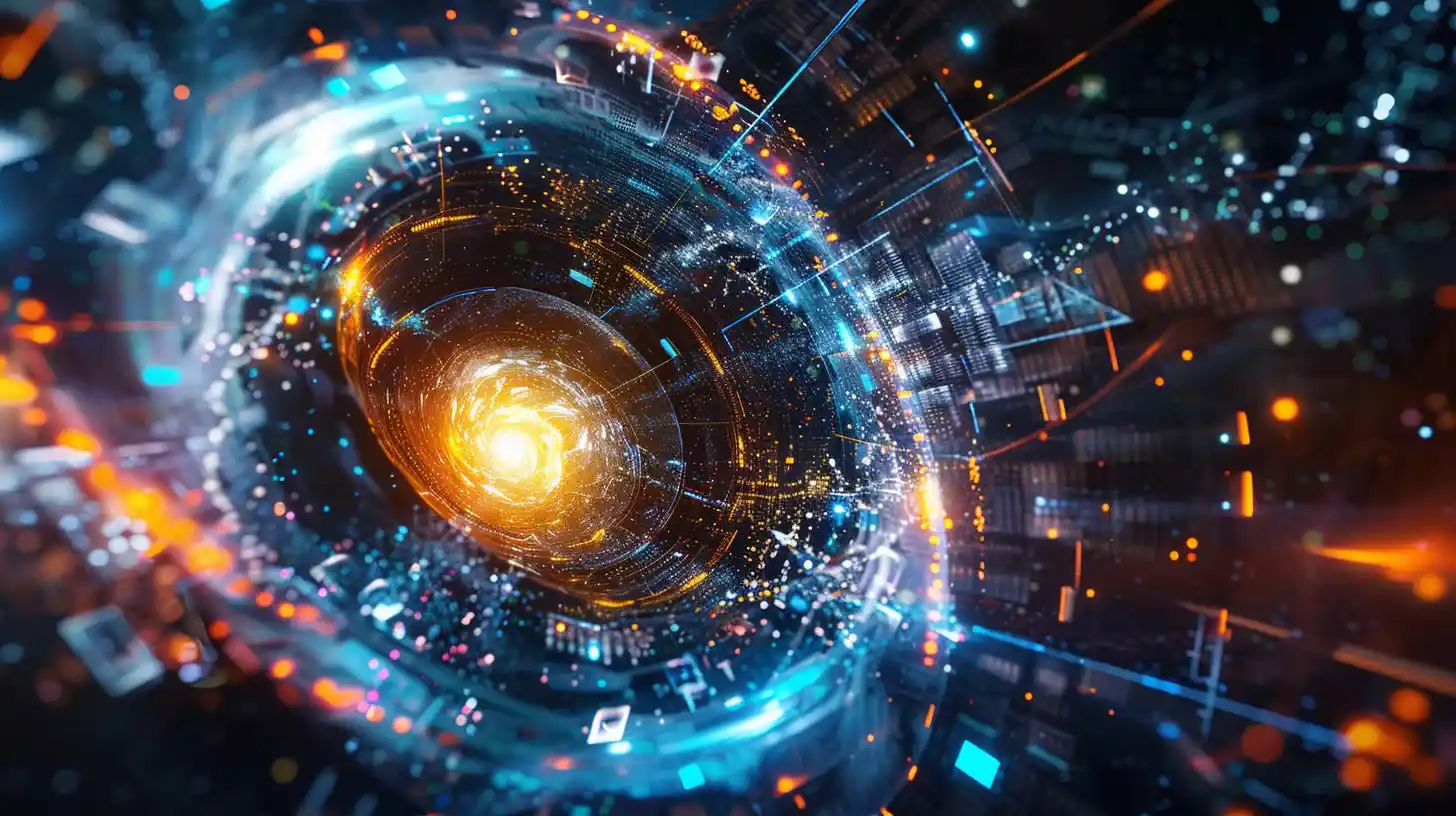
The Shift to the Boardroom of AI Evolution
The challenge of assessing LLMs has transcended academic circles, becoming a pivotal concern for corporate executives. According to a KPMG survey of over 1,300 global CEOs, generative AI has emerged as the primary investment focus for 70% of them. This shift underscores the importance of trust in technology, with Shelley McKinley of GitHub emphasizing the necessity for companies to deliver reliable products.
Moreover, governments are grappling with the deployment and risk management of contemporary AI models. This concern led to the US and UK establishing a bilateral arrangement on AI safety, reinforcing their commitment to minimizing surprises from rapid advancements in AI Evolution.
Rethinking Evaluation Criteria Of AI Evolution
The task of evaluating AI systems now demands a proactive approach to keep pace with technological advancements. Stanford’s Rishi Bommasani points out the difficulty in assessing AI models akin to human evaluation. With the development of the Holistic Evaluation of Language Models by Bommasani’s team, new criteria like reasoning, memorization, and vulnerability to misinformation are being considered.
However, the complexity of modern AI models, capable of executing interconnected tasks, presents a significant challenge to traditional controlled evaluation settings. Mike Volpi of Index Ventures highlights the complexity of evaluating AI, likening it to the multifaceted nature of human intelligence.
The Future of AI Evaluation
The contamination of models’ training data with evaluation questions and the monolithic nature of benchmarks represent growing concerns. This has led to innovative approaches like Hugging Face’s LMSys leaderboard, which offers a more user-centric evaluation by allowing bespoke tests.
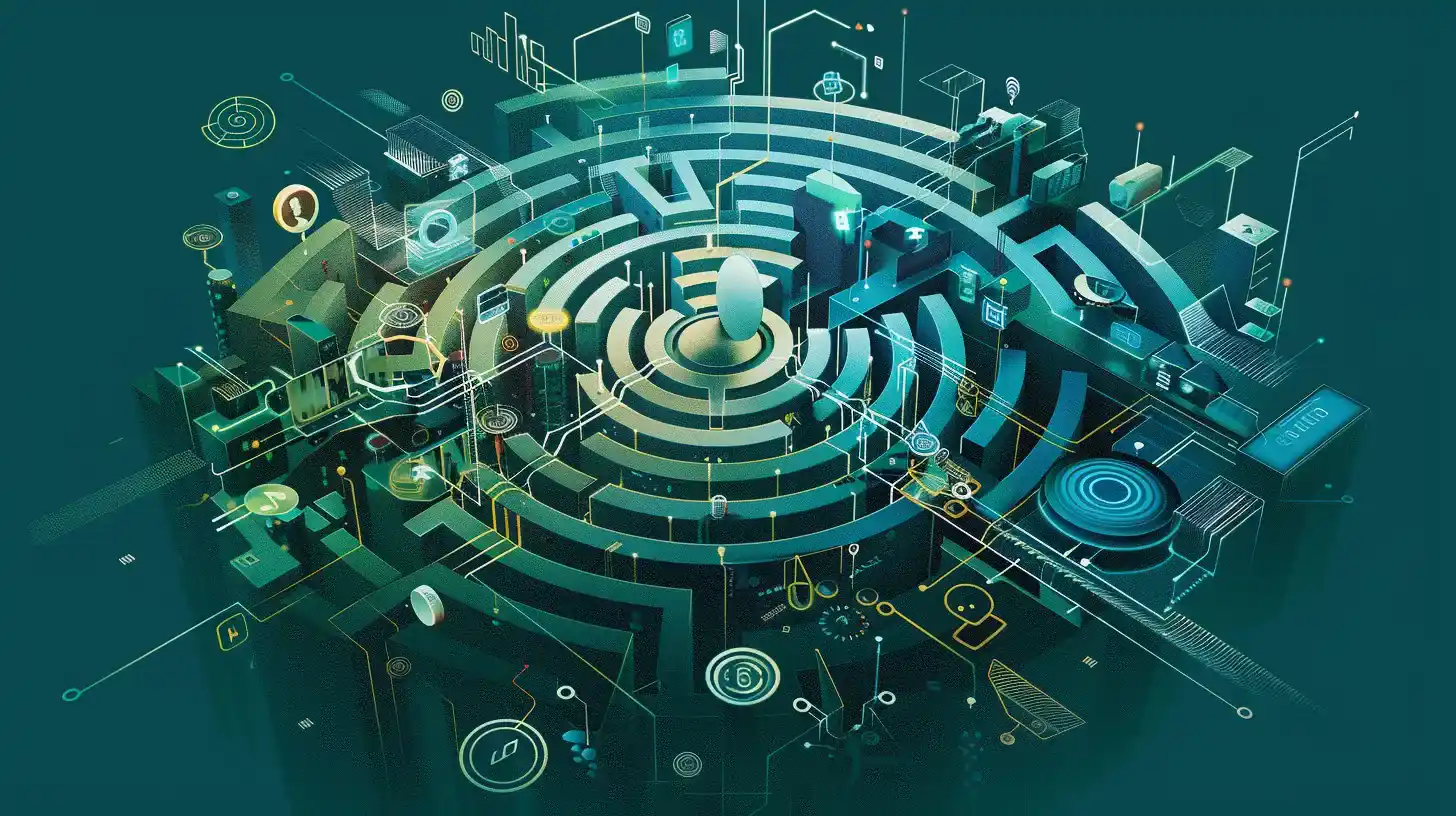
Cohere’s Gomez suggests a more tailored approach for businesses, advocating for internal test sets complemented by human evaluation. This perspective aligns with the notion that selecting AI models involves both art and science, emphasizing experience over mere metrics.
Embracing Complexity in Evaluation
The complexity of AI systems necessitates a multifaceted approach to evaluation, recognizing that no single metric or benchmark can capture the full range of an AI model’s capabilities. This realization is leading to the development of composite benchmarks that assess models across multiple dimensions, including ethical considerations, societal impact, and real-world applicability.
For instance, the Massive Multitask Language Understanding benchmark and the HumanEval system for evaluating coding ability represent steps towards more comprehensive evaluation frameworks. These systems attempt to measure AI models’ performance across a wide array of tasks and challenges, reflecting the diverse applications of AI in the real world.
Conclusion
As AI continues to evolve, the challenge of accurately assessing its capabilities becomes increasingly complex. Traditional benchmarks are proving inadequate, necessitating a shift towards more dynamic and comprehensive evaluation methods. This evolution in assessment parallels the broader transition in AI technology, requiring businesses, governments, and researchers to adapt to the rapid pace of innovation.
The journey of AI evolution is as much about the development of new technologies as it is about redefining how we understand, measure, and integrate these advancements into society.