Table of Contents
AI Deciphers Brainwaves into Text
The utilization of AI Deciphers Brainwaves scalp-based system to capture the electrical signals of the brain enables the transformation of thoughts into words, aided by a comprehensive language model. However, it is important to note that the outcomes obtained are considerably imperfect.
The enhancement of a widely-used AI Deciphers Brainwaves for generating images from text now enables it to directly convert brain signals into visual representations. Nevertheless, the current implementation necessitates extensive training with cumbersome and expensive imaging apparatus, indicating that the prospect of everyday mind reading remains distant.
Shinji Nishimoto and Yu Takagi from Osaka University in Japan have devised a significantly more straightforward technique by leveraging Stable Diffusion, a text-to-image generator introduced by Stability AI Deciphers Brainwaves in August 2022. Their novel approach employs thousands of parameters instead of millions.
Stable Diffusion, when employed in its regular manner, transforms a textual prompt into an image by commencing with arbitrary visual disturbances and adjusting them to generate images that bear a resemblance to those in its training data with comparable textual descriptions.
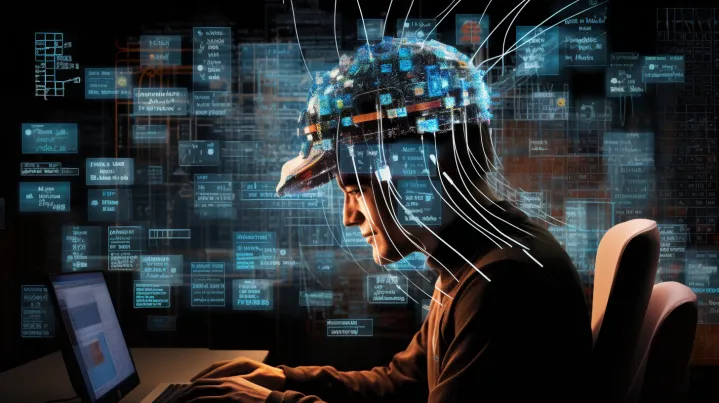
Unlocking Minds: Harnessing EEG & AI for Thought-to-Text Innovation
A group of researchers has recently revealed their groundbreaking achievement of transforming human thoughts into written words solely by utilizing a helmet equipped with various sensors and artificial intelligence technology. During the experiment, the subjects wore a cap that captured the electrical brain activity from their scalp while reading text passages. Subsequently, an advanced AI model named DeWave was employed to convert the recorded electroencephalogram (EEG) data into written text.
Chin-Teng Lin, a professor at the University of Technology Sydney (UTS) in Australia, affirms that the technology is non-intrusive, reasonably priced, and conveniently portable.
Despite its imperfections, the system has shown an accuracy of around 40 percent, according to Lin. However, recent data that is currently undergoing peer review indicates that the accuracy has improved and now exceeds 60 percent. During the presentation at the NeurIPS conference in New Orleans, Louisiana, participants were required to read the sentences out loud, even though the DeWave program does not utilize spoken words. Nevertheless, in the team’s most recent study, participants were instructed to read the sentences silently.
Jerry Tang and his team at the University of Texas at Austin achieved a comparable level of accuracy in converting thoughts to text named “AI Deciphers Brainwaves” last year. However, unlike their approach of using MRI scans to interpret brain activity, using EEG is a more practical method as it allows subjects to avoid the need to lie still inside a scanner.
Merging Mind and Machine: Training AI to Translate Brain Signals into Text
Team member Charles Zhou at UTS explains that AI Deciphers Brainwaves the DeWave model was trained by analyzing numerous instances where brain signals corresponded to particular sentences. Zhou further elaborates that when an individual contemplates uttering the word ‘hello’, specific signals are emitted by the brain. Through AI Deciphers Brainwaves’ exposure to various examples of these signals for different words or sentences, DeWave comprehends the correlation between these signals and the word ‘hello’.
Zhou explains that after gaining a thorough understanding of the brain signals, DeWave successfully integrated them with an open-source large language model (LLM), similar to the AI technology behind ChatGPT. According to Zhou, this LLM functions as an intelligent writer capable of constructing sentences. The AI Deciphers Brainwaves team instructs the writer to carefully consider the signals received from DeWave and utilize them as a framework for generating coherent sentences.
In the end, the team conducted joint training sessions for both DeWave and the language model to enhance their sentence writing abilities using the EEG data. Craig Jin from the University of Sydney expresses his admiration for the achievements of Lin’s team, stating that their work represents significant progress.
For a considerable duration, individuals have expressed their desire to convert EEG signals into text, and the team’s model is demonstrating an impressive level of accuracy. In contrast, a few years ago, the translations from EEG to text were entirely nonsensical.