Table of Contents
In today’s tech landscape, AI is ubiquitous, enhancing efficiency across sectors. Yet, ensuring fairness, transparency, and accountability is critical. AI auditing tools address this need, offering functionalities to assess, monitor, and mitigate biases in AI systems. They allow organizations to scrutinize models, ensuring compliance with ethical standards and regulations.
By leveraging these tools, stakeholders gain insights into AI systems, identifying and rectifying bias. Moreover, they facilitate ongoing monitoring, helping organizations adapt to evolving ethical standards. In this article, we explore six top AI auditing tools, their features, applications, and significance in promoting ethical AI solutions. Through their adoption, organizations can instill trust, accountability, and transparency in AI technologies.
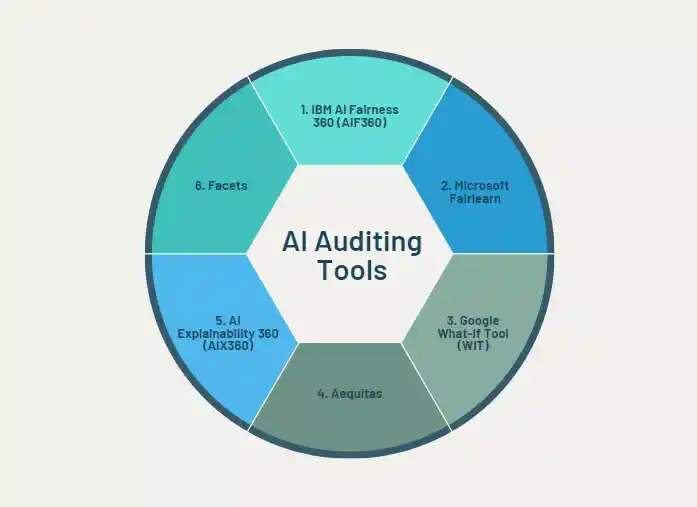
1. IBM AI Fairness 360 (AIF360)
IBM AI Fairness 360 (AIF360) is a robust toolkit engineered to identify and rectify biases within machine learning models and datasets. Equipped with an extensive range of pre-established fairness metrics and bias mitigation algorithms, AIF360 facilitates a meticulous examination of model fairness across diverse demographic segments and application domains.
Applications:
AIF360 boasts widespread applicability across industries such as finance, healthcare, hiring, and criminal justice, where the need for fairness and accountability in AI systems is paramount. In finance, for instance, it can aid in mitigating biases in lending decisions. Similarly, in healthcare, AIF360 can ensure fairness in diagnostic algorithms, thus promoting equitable treatment for all patient demographics.
How to Use:
AIF360’s features enable users to detect biases, develop fairness-aware models, and mitigate biases effectively. Leveraging its pre-built metrics and algorithms, users can thoroughly assess model fairness, implement fairness-aware training procedures, and customize adjustments to suit specific use cases. This empowers users to tailor the AI auditing process to their unique requirements, ensuring comprehensive and effective bias mitigation.
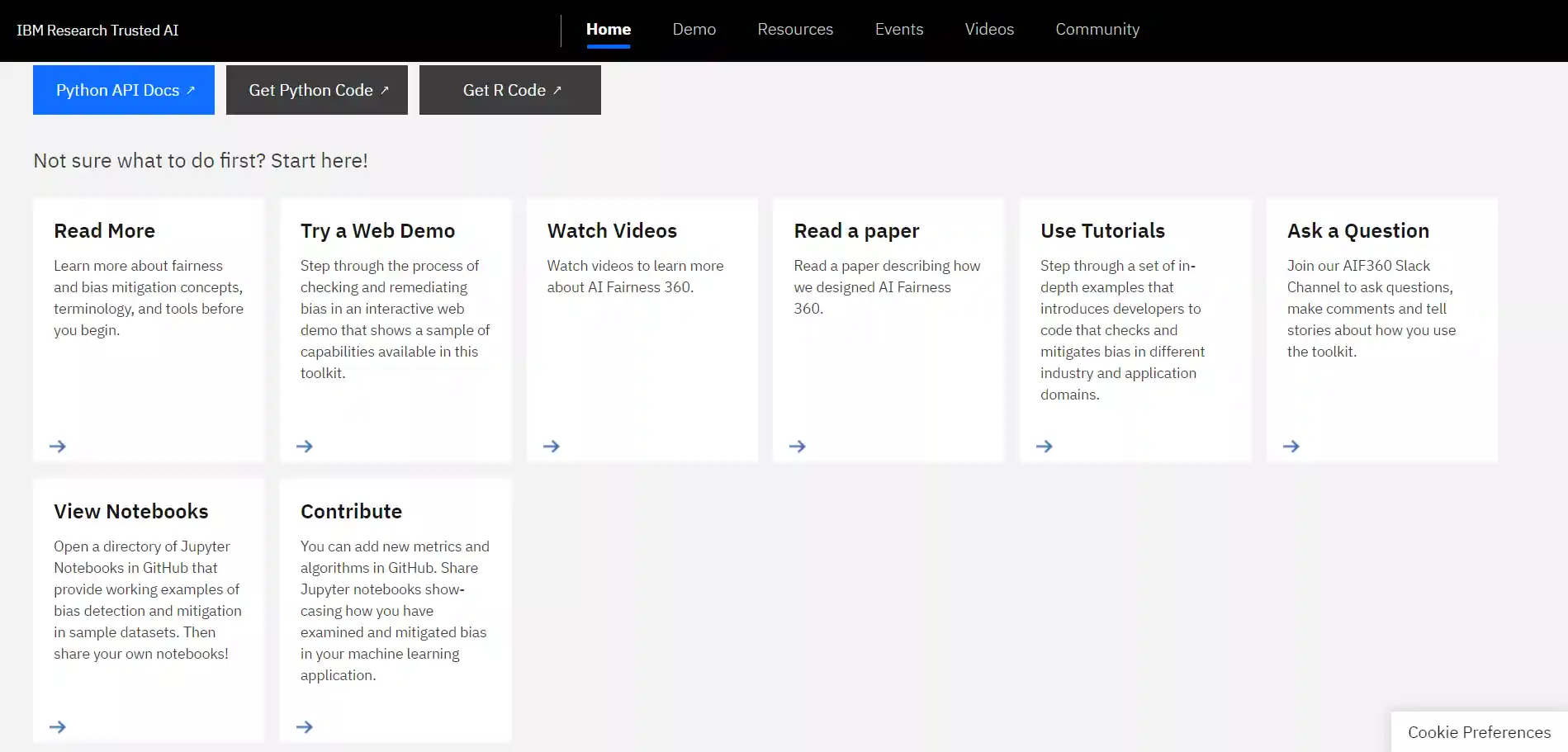
2. Microsoft Fairlearn
Microsoft Fairlearn, a potent AI auditing tool, offers comprehensive functionalities to assess and mitigate unfairness in machine learning models. Through innovative techniques for fairness comparison, mitigation, and model selection, Fairlearn empowers users to navigate ethical considerations in AI development. Leveraging metrics like group fairness and individual fairness, Fairlearn facilitates a nuanced understanding of model biases, enabling informed decision-making across diverse applications.
Applications:
Fairlearn is utilized in various industries, especially where fairness is crucial. In lending, it identifies and rectifies biases affecting demographic groups. In hiring, it mitigates biases to prevent discrimination. In predictive policing, it helps ensure AI models avoid perpetuating biases in crime prediction and interventions. Fairlearn’s applications underscore its role as an essential AI auditing tool in promoting fairness and equity.
How to Use:
To utilize Microsoft Fairlearn for auditing, and integrate it into machine learning workflows. Fairlearn offers user-friendly interfaces and documentation for guidance. Start by assessing model fairness with built-in metrics like disparate impact. Explore mitigation strategies, adjusting parameters, or retraining datasets. Fairlearn aids in model selection by comparing performance and fairness, fostering the adoption of ethical AI solutions.
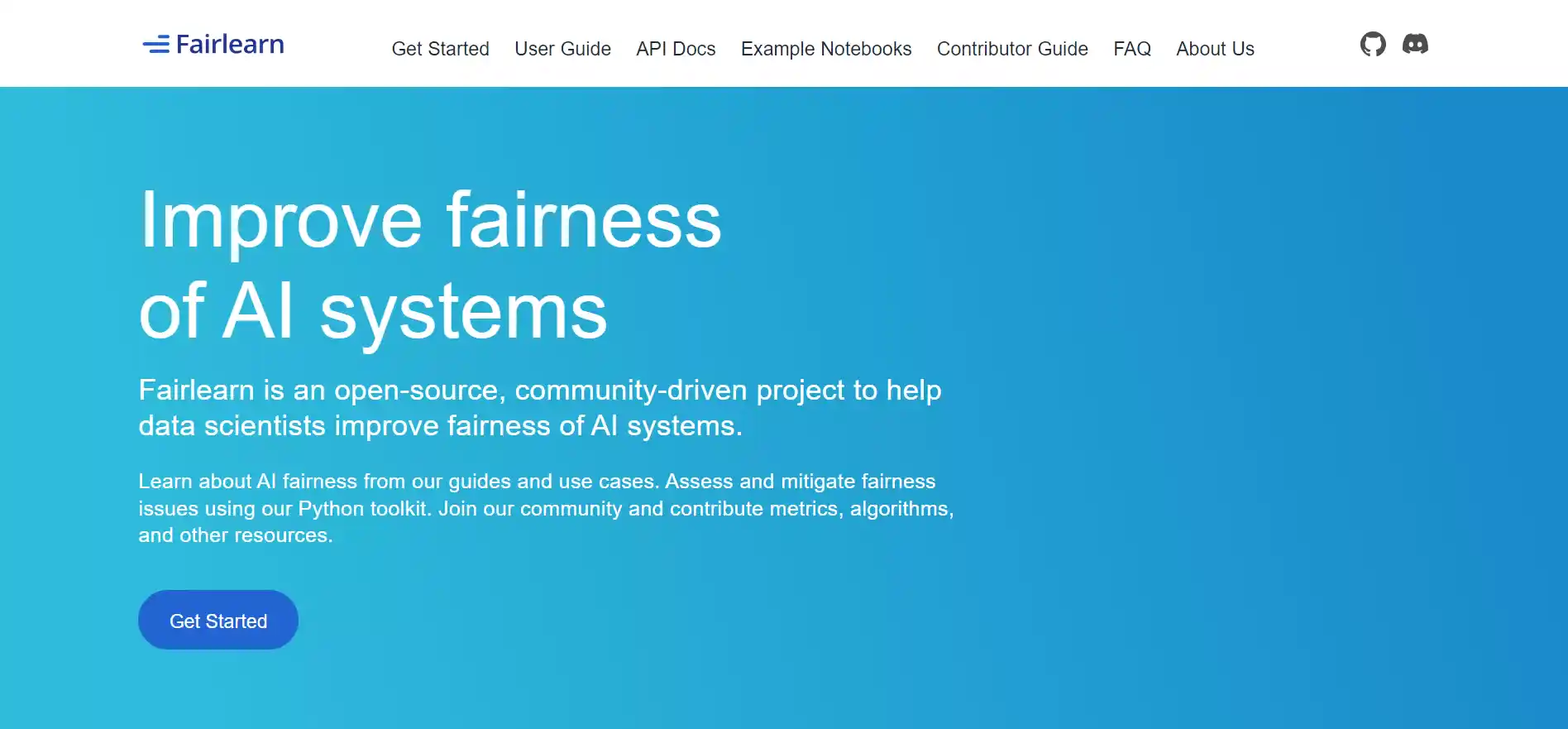
3. Google What-If Tool (WIT)
Google’s What-If Tool (WIT) aids AI auditing through interactive exploration and visualization of model predictions. Users manipulate input features to observe prediction changes, revealing biases or inconsistencies. In healthcare, for instance, adjusting patient demographics highlights how the model responds, aiding in bias detection. WIT’s interactive features facilitate a deeper understanding of model behavior, crucial for ensuring fairness and transparency in AI systems.
Applications:
WIT excels in bias detection and fairness evaluation, pivotal in AI auditing. Users analyze disparities in model outcomes across demographic groups like gender or ethnicity. Visualizing and comparing predictions between groups, stakeholders assess fairness. In hiring, users evaluate if recommendations differ based on protected characteristics, aiding bias mitigation. This analysis identifies and mitigates biases, promoting fairness and equity in decision-making.
How to Use:
Utilize AI auditing tools by inputting datasets and selecting models. Explore predictions, adjust features, and compare multiple models to assess biases across demographic groups. Analyze disparities to ensure fairness, iterating through scenarios to optimize performance. Share insights for transparency and accountability. By following these steps, users effectively leverage AI auditing tools like WIT to promote fairness and reliability in AI systems.
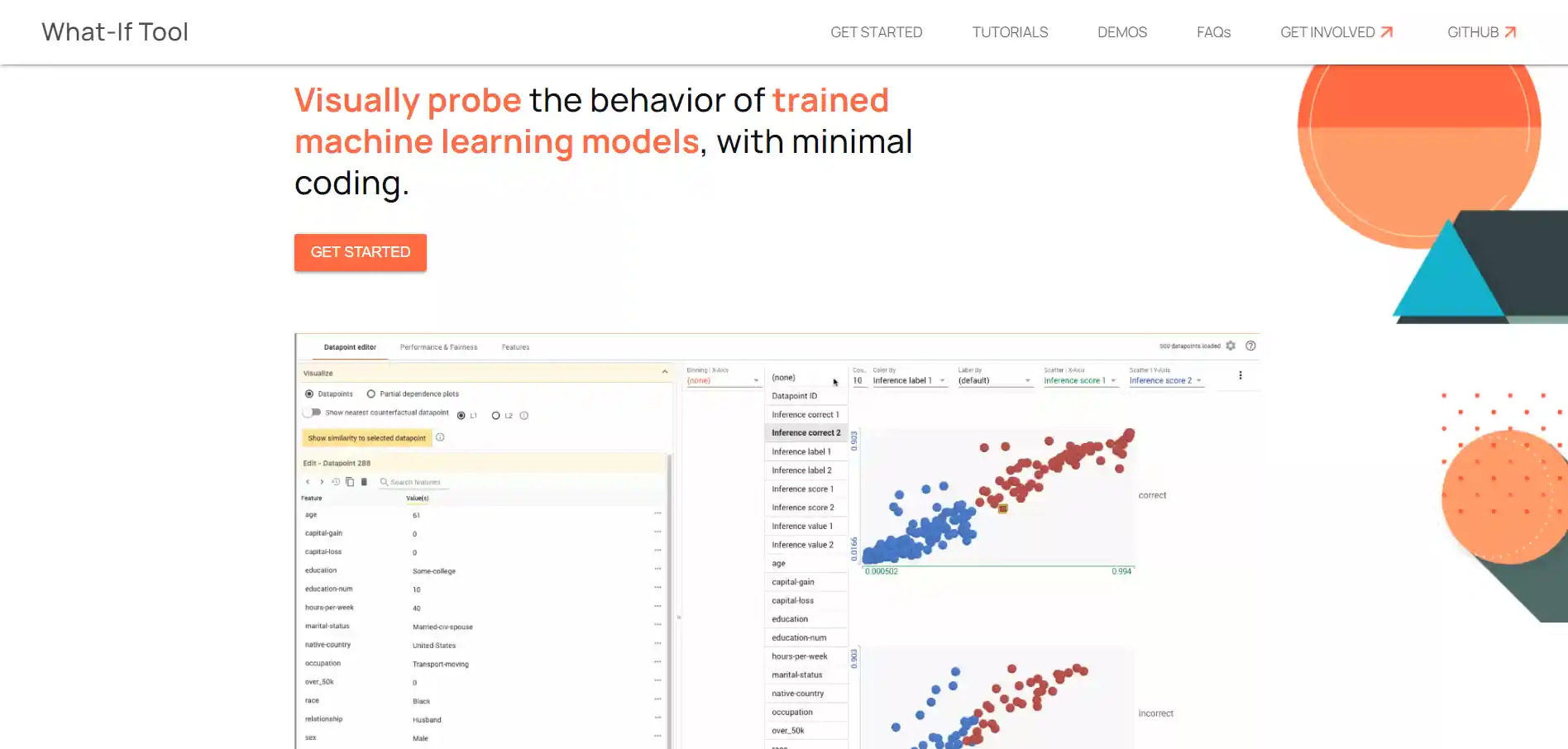
4. Aequitas
Aequitas, an open-source bias audit toolkit, assesses and mitigates bias in machine learning models. Its features include fairness metrics computation, visualization, and bias detection across diverse demographic groups. Users can identify biases in their AI systems and take proactive steps to mitigate them, fostering fairness and equity. Aequitas empowers users to ensure ethical and responsible AI solutions.
Applications:
Aequitas is utilized across sectors like lending, hiring, criminal justice, and healthcare. In lending, it ensures fair access to financial services. For hiring, it eliminates biases in candidate selection. In criminal justice, Aequitas identifies and rectifies biases in risk assessment tools. In healthcare, it promotes fairness and equity in medical decision-making processes. AI Auditing Tool Aequitas fosters fairness across diverse domains.
How to use:
To utilize Aequitas, input machine-learning models into the toolkit. It computes fairness metrics and visualizes biases. Examine metrics across demographics to spot disparities. Armed with insights, implement bias mitigation like adjusting features or adding fairness constraints in training. Regular audits with Aequitas maintain ongoing fairness and accountability in AI systems.
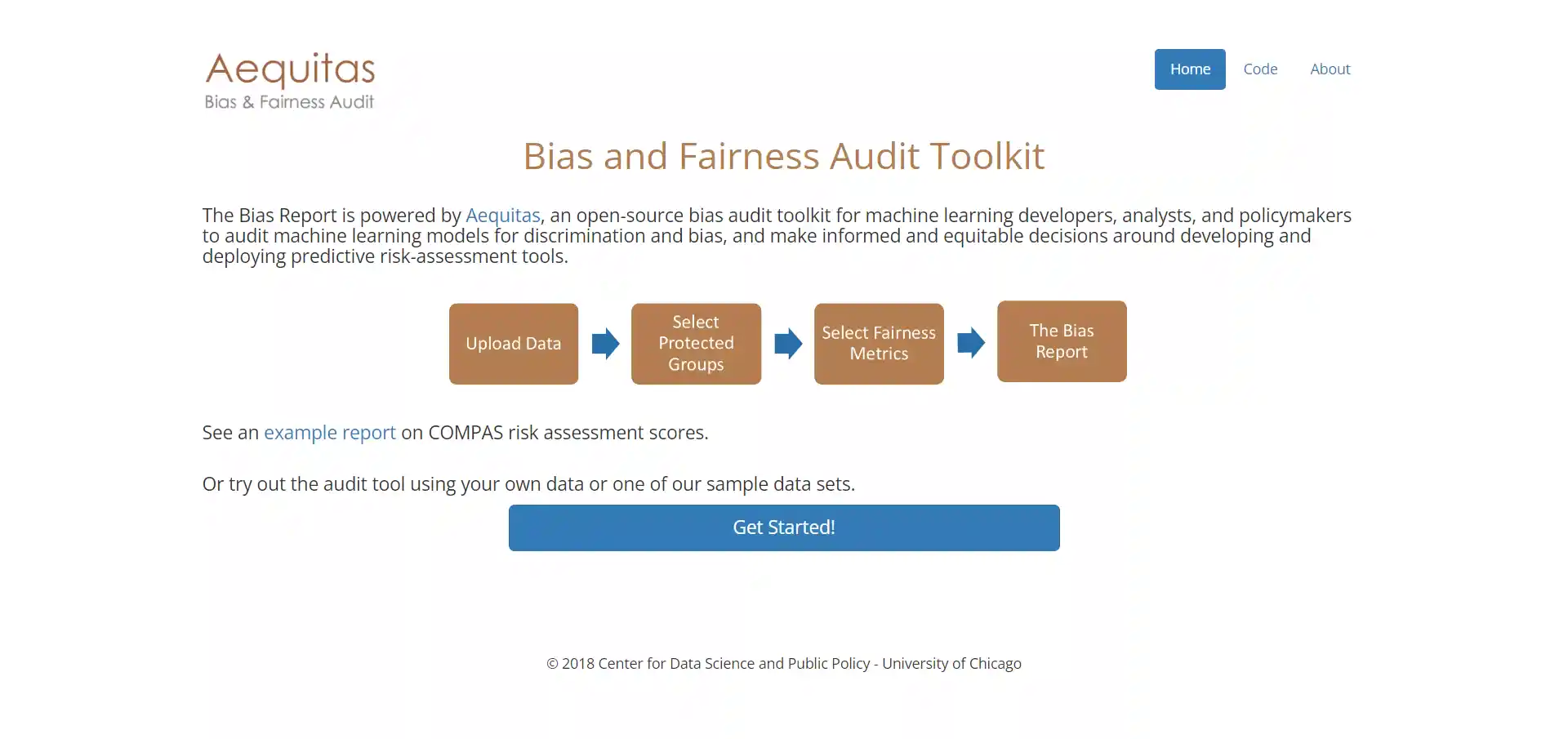
5. AI Explainability 360 (AIX360)
AI Explainability 360 (AIX360) enhances machine learning model interpretability with various tools and algorithms. It enables users to delve into model decisions, revealing how features influence outcomes. By aiding in the interpretation of predictions, AIX360 ensures transparency and accountability in AI systems. This AI auditing tool is vital for promoting understanding and trust in AI technologies.
Applications of AIX360 in AI Auditing
AIX360 is a widely used AI auditing tool, especially in transparent and accountable domains. For example, in model debugging, it identifies biases, aiding refinement. In regulatory compliance, AIX360 offers explanations for decisions, ensuring adherence to ethical and legal standards. Its role in auditing strengthens AI systems, fostering trust and integrity in their deployment across various sectors.
Utilizing AIX360 for Trust-building
AIX360 is pivotal in trust-building for AI technologies. Providing explanations for individual predictions, fosters user and stakeholder trust by revealing decision-making processes. This transparency enhances confidence in AI system reliability and fairness. Across domains like healthcare diagnostics and financial risk assessment, AIX360 empowers users with clear, interpretable explanations, reinforcing trust in AI systems.
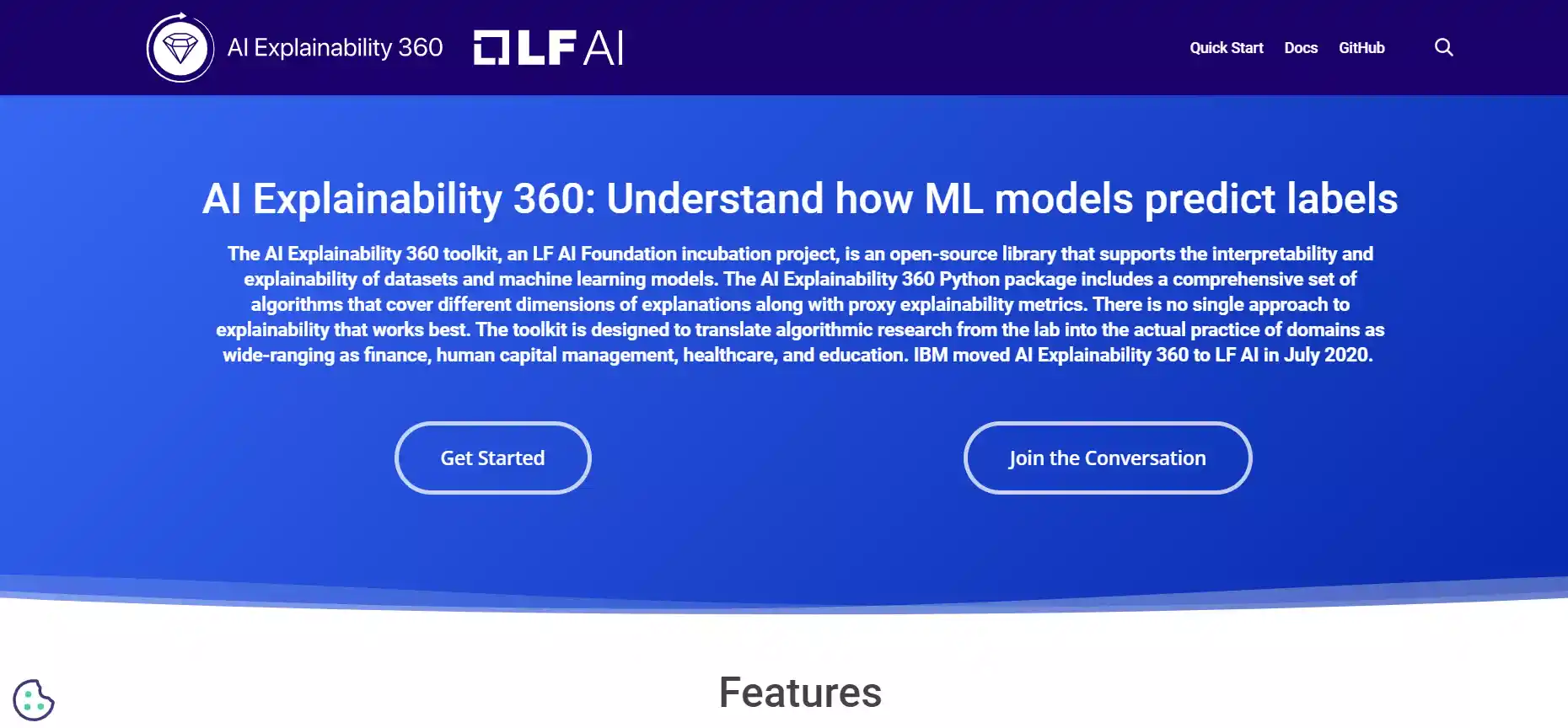
6. Facets
Facets stands as a robust suite offering visualization tools crucial for comprehending and scrutinizing machine learning datasets. Its intuitive interface empowers users to delve into dataset statistics, distributions, and inter-feature relationships with ease. This accessibility makes Facets an indispensable ally in the realm of data auditing and exploration within AI systems.
Applications: Facets find extensive utility across the AI landscape, serving as a cornerstone for data preprocessing, cleaning, and quality assessment. By providing deep insights into dataset biases and structural intricacies, it plays a pivotal role in ensuring data integrity—a fundamental prerequisite for building reliable and robust AI systems.
Utilization: To leverage Facets effectively, users can upload datasets and explore various facets of the data using the interactive visualizations provided. Through this process, stakeholders can identify patterns, anomalies, and potential biases within the dataset, enabling informed decision-making and enhancing the overall reliability of AI auditing tools.
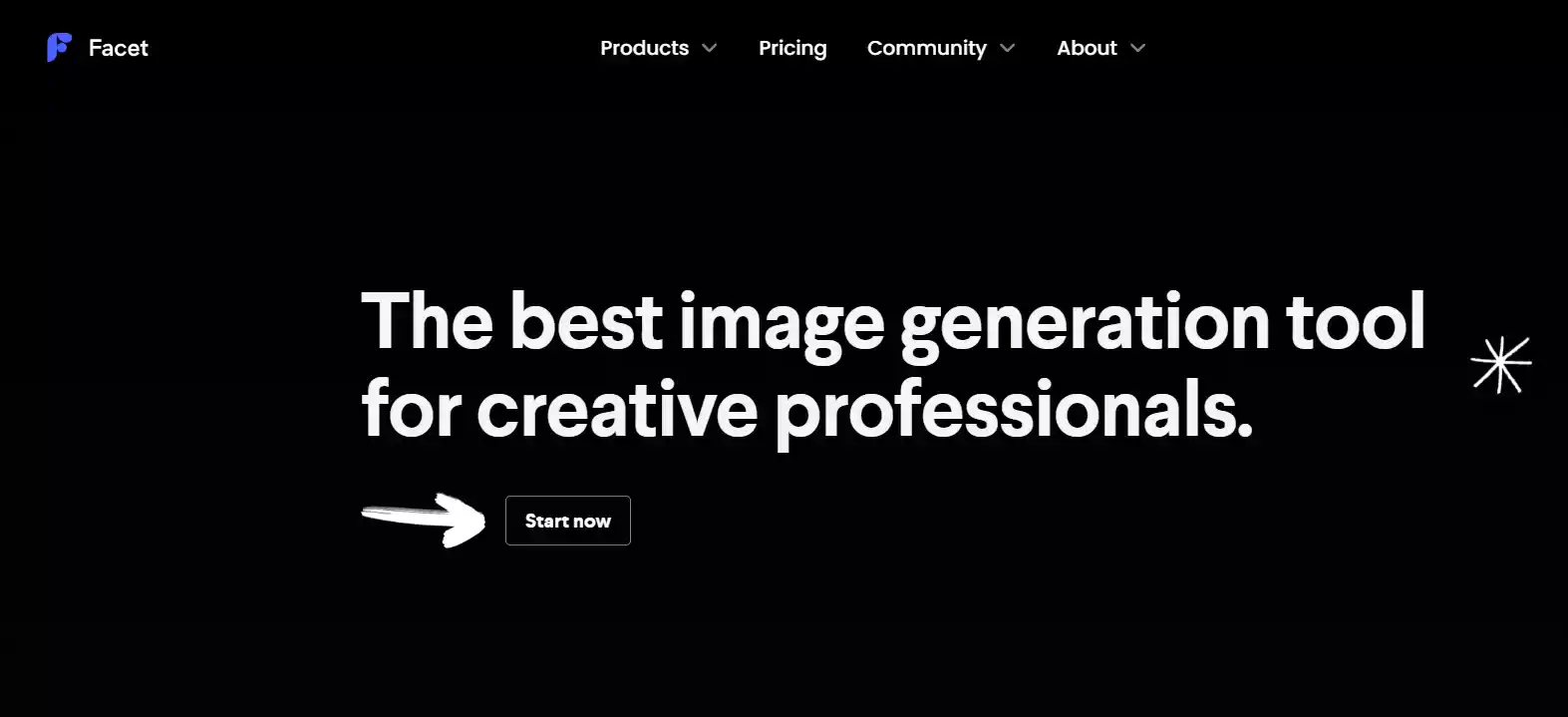
Related Article:
AI Auditing Systems: Improving Accuracy with 60% Surge
Wrapping Up: The Role of AI Auditing Tools
AI auditing tools are vital for fairness, transparency, and accountability in AI systems. They detect and mitigate biases, explain model decisions, and ensure data integrity. Empowering organizations, they foster ethical AI solutions, free from discrimination.
As AI permeates society, adopting these tools becomes imperative to prevent unintended consequences and promote ethical AI use. Leveraging these tools, organizations advance towards equitable and trustworthy AI systems. Incorporating AI auditing tools demonstrates a commitment to ethical AI and societal well-being.