Table of Contents
In today’s data-driven world, AI auditing systems are indispensable guardians of reliability. As technology advances, the need for these systems to evolve becomes even more apparent. AI auditing systems serve as meticulous overseers, meticulously examining the inner workings of AI algorithms and models.
Their primary objective is to ensure that data processing adheres to stringent standards of compliance, transparency, and fairness. By scrutinizing every aspect of the AI ecosystem, from data collection to model deployment, these systems uphold the integrity of AI-driven decision-making processes. They act as gatekeepers, filtering out biases, errors, and inaccuracies that could compromise the reliability of AI systems.
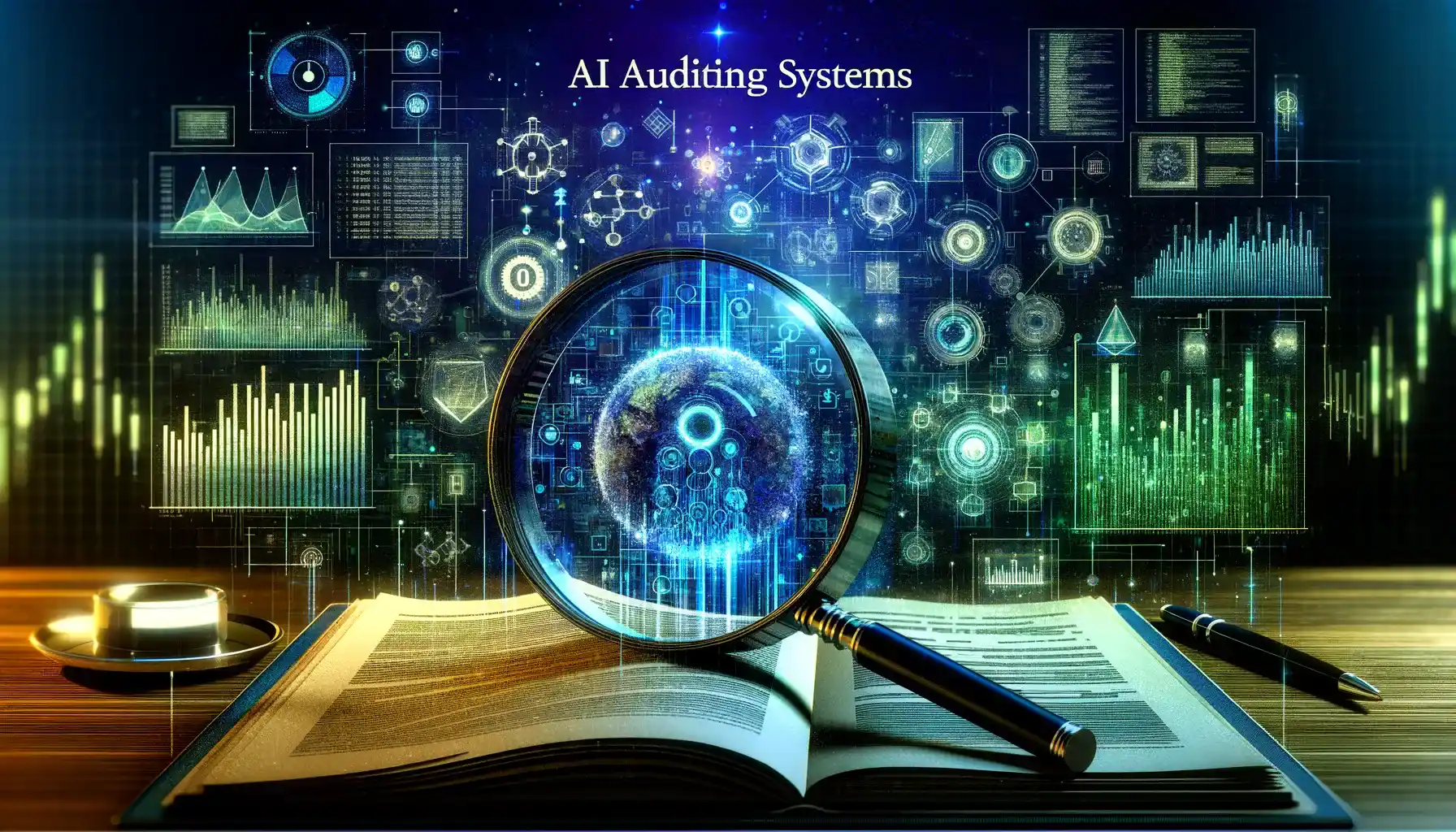
In this dynamic landscape, where the stakes are high and trust is paramount, AI auditing systems stand as sentinels, safeguarding the integrity of AI technology. Their evolution towards enhancing accuracy, with a remarkable surge of 60%, underscores their pivotal role in maintaining the trustworthiness of AI systems amidst an ever-expanding sea of data.
Challenges in Accuracy
While AI Auditing Systems have seen remarkable advancements, challenges persist in ensuring accuracy. Biases, stemming from various sources such as historical data or algorithmic design, can skew results and compromise reliability. Additionally, data quality issues, including incompleteness or inconsistency, present obstacles to precise analysis. Algorithmic complexities further exacerbate these challenges, as intricate models may introduce unforeseen errors or biases.
Addressing these hurdles is imperative to uphold the integrity and trustworthiness of AI systems. Without accurate auditing mechanisms, the potential consequences, such as erroneous decision-making or biased outcomes, could undermine the effectiveness and adoption of AI technologies. Therefore, ongoing efforts to refine AI Auditing Systems are essential to mitigate these challenges and ensure their capability to provide accurate assessments of AI algorithms and models.
The 60% Surge: Methodology and Impact
The surge of 60% in AI auditing systems’ accuracy is a testament to recent technological breakthroughs. This improvement signifies a pivotal moment, indicating a transformative shift in the capabilities of these systems. The methodology behind this surge is multifaceted, drawing upon advancements in machine learning algorithms, data preprocessing techniques, and model interpretability.
Innovations in these areas have addressed longstanding challenges, such as biases in data, quality issues, and algorithmic complexities. Furthermore, the impact of this surge extends far beyond numerical metrics. Enhanced accuracy translates into increased reliability, instilling trust among stakeholders and fostering greater confidence in AI-powered decision-making processes.
Organizations across various sectors are poised to benefit from this advancement, as AI auditing systems become more adept at ensuring compliance, mitigating risks, and optimizing operations. As these systems continue to evolve, the impact of the 60% surge reverberates throughout the AI landscape, driving innovation and paving the way for a more transparent and trustworthy future.
Technological Innovations
Technological innovations in AI auditing systems have been pivotal in driving a remarkable surge in accuracy. One such innovation lies in the advancements made in machine learning algorithms, which have enabled these systems to better analyze and interpret complex datasets.
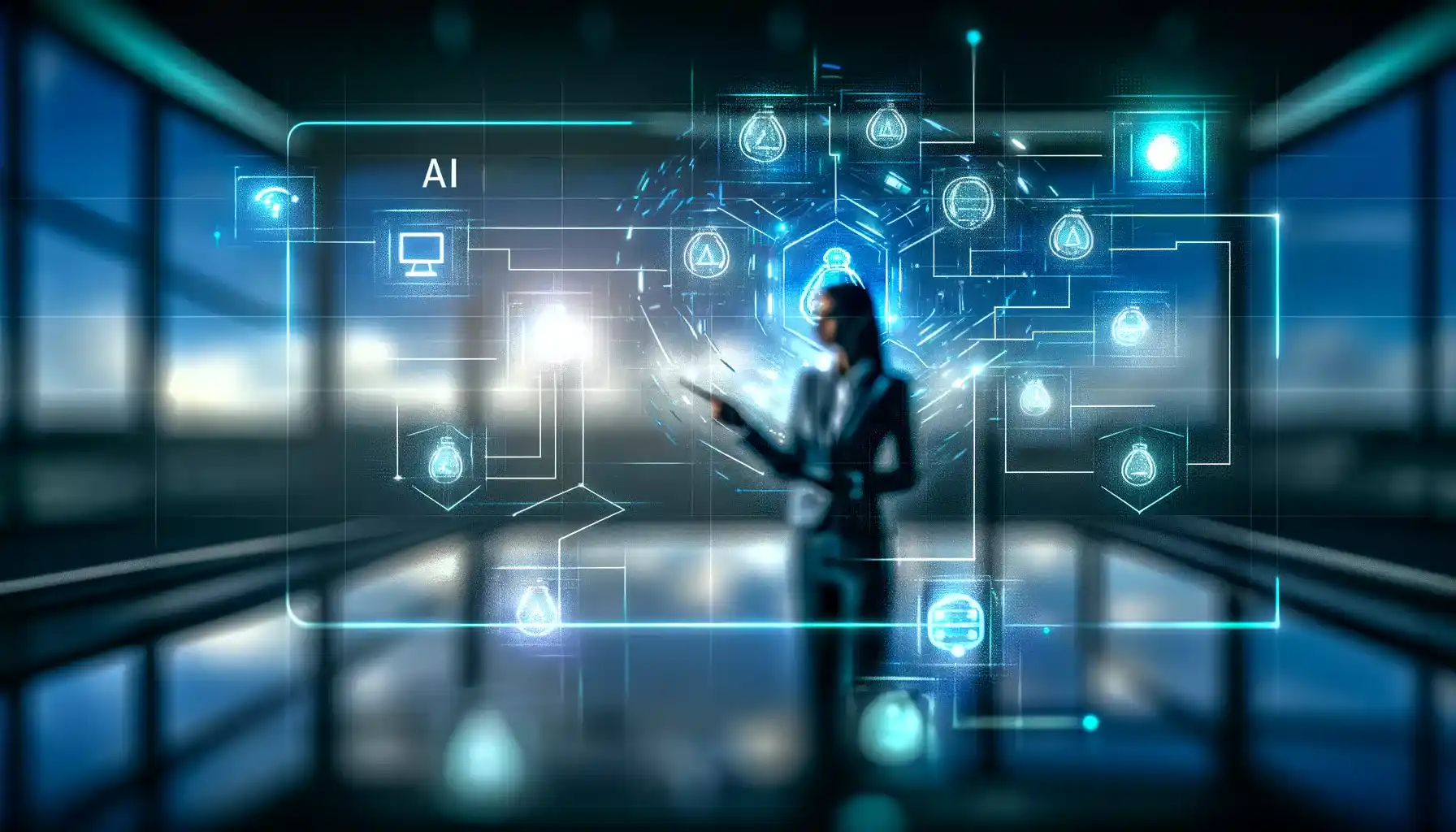
Additionally, improvements in data preprocessing techniques have played a crucial role in enhancing the quality of input data, thus leading to more reliable outcomes. Moreover, innovations in model interpretability have helped uncover and mitigate inherent biases within AI systems, ensuring fairness and transparency.
By addressing these key areas, AI auditing systems have become more adept at navigating algorithmic complexities, ultimately resulting in a substantial improvement in their overall accuracy. These technological advancements not only signify a significant leap forward in AI technology but also underscore the critical role that innovation plays in shaping the future of auditing processes.
Benefits Beyond Accuracy
Improved accuracy in AI Auditing Systems yields a myriad of benefits that transcend numerical measurements. Enhanced reliability establishes a foundation of trust among stakeholders, instilling confidence in the outputs generated by these systems. This trust is invaluable, particularly in industries where decisions are reliant on AI-generated insights. Furthermore, heightened accuracy fosters regulatory compliance, ensuring that organizations adhere to legal frameworks and industry standards.
By providing transparent and verifiable results, AI auditing systems help mitigate risks associated with non-compliance, safeguarding both businesses and consumers. Moreover, the pursuit of accuracy drives innovation in AI research and development. As organizations strive to push the boundaries of what is possible, they invest in refining algorithms, improving data quality, and enhancing interpretability.
This cycle of innovation not only enhances the performance of AI systems but also expands their applicability across various domains. Ultimately, the benefits beyond accuracy underscore the transformative potential of AI auditing systems in shaping a more reliable, compliant, and innovative AI landscape.
Future Outlook for AI Auditing Systems
The trajectory of AI auditing systems appears exceptionally promising. The continuous evolution of technology, characterized by breakthroughs in machine learning algorithms and data processing methodologies, holds immense potential for further enhancing the accuracy and reliability of these systems. Moreover, ongoing research endeavors dedicated to exploring the ethical and regulatory dimensions of AI will play a pivotal role in shaping the future landscape.
By addressing concerns surrounding biases, transparency, and accountability, these efforts will not only bolster the effectiveness of AI auditing systems but also foster greater trust and acceptance among stakeholders. With each stride forward, the horizon of possibilities expands, paving the way for a future where AI auditing systems serve as stalwarts of integrity, ensuring the ethical and responsible deployment of artificial intelligence across diverse domains.
Final Reflections: AI Auditing Systems
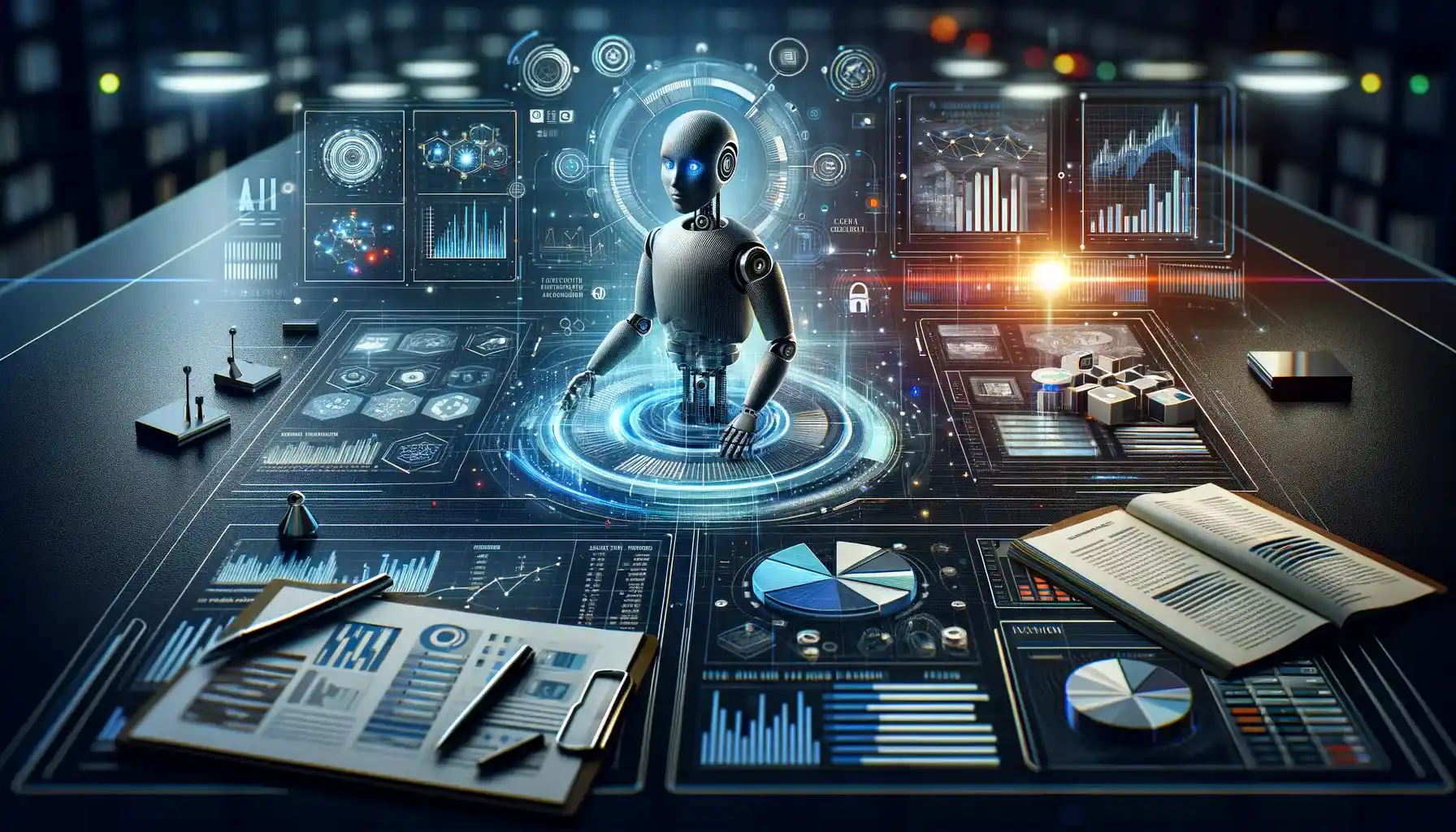
The surge in accuracy witnessed in AI auditing systems marks a pivotal moment in the advancement of AI technology. This surge signifies a substantial leap forward, addressing longstanding challenges that have hindered the reliability and trustworthiness of AI systems.
By tackling issues such as biases, data quality concerns, and algorithmic complexities, these systems are revolutionizing how AI models are evaluated and validated. Moreover, the emphasis on embracing ethical principles ensures that AI auditing systems not only deliver accurate results but also prioritize fairness, transparency, and accountability.
As a result, these systems are not just tools for improving accuracy; they are catalysts for reshaping the entire AI landscape. Through their rigorous scrutiny and validation processes, AI auditing systems are laying the groundwork for a future where AI technologies are more transparent, accountable, and ultimately trustworthy. This evolution is essential for fostering public confidence in AI applications across various sectors and driving continued innovation in the field.