Table of Contents
Understanding the Importance of Peripheral Vision
Humans possess a remarkable ability to perceive shapes and objects outside their direct line of sight, thanks to peripheral vision. This expansive field of vision, although less detailed than central vision, plays a crucial role in detecting potential hazards, such as vehicles approaching from the side.
It enables us to maintain awareness of our surroundings, enhancing our ability to navigate the world safely and efficiently.
Addressing the Gap in AI: The Lack of Peripheral Vision
In contrast to humans, artificial intelligence (AI) lacks peripheral vision. While AI models excel at tasks requiring focused attention on specific objects or regions, they struggle to perceive the broader context provided by peripheral vision.
This limitation hinders their ability to detect approaching dangers and anticipate human responses in real-world scenarios. However, equipping AI models with it capabilities could significantly enhance their situational awareness and decision-making abilities, leading to safer and more effective AI systems.
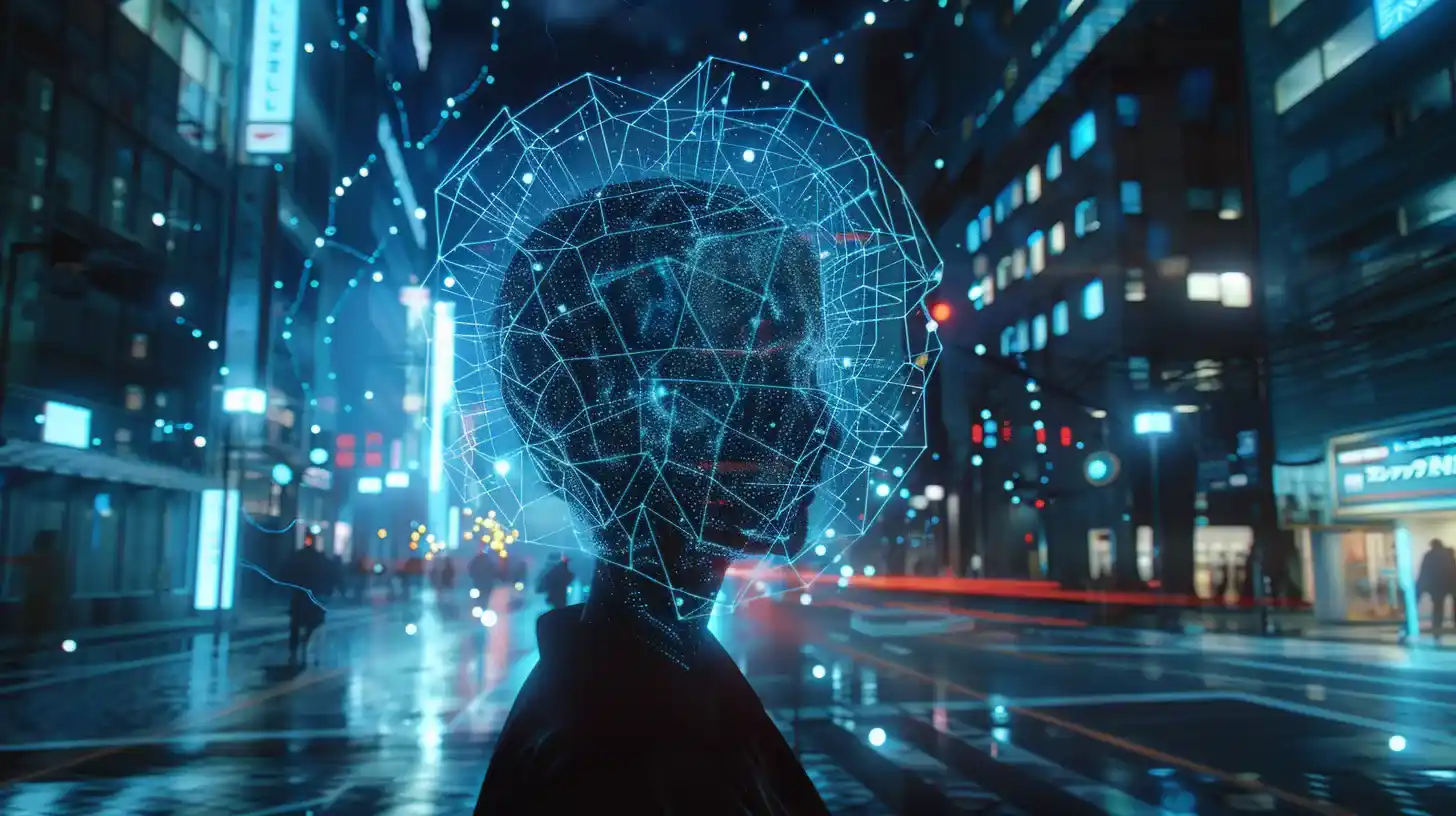
MIT Researchers Pioneer Simulation of Peripheral Vision in AI Models
In a groundbreaking study conducted at the Massachusetts Institute of Technology (MIT), researchers sought to bridge the gap between human and artificial vision by simulating AI models.
The research team, led by Anne Harrington and Vasha DuTell, developed an innovative image dataset specifically designed to mimic the characteristics of human peripheral vision.
The dataset, created through a modified technique known as the texture tiling model, accurately represents the loss of detail that occurs as visual information moves away from the central point of focus.
By generating a diverse range of transformed images that simulate peripheral vision distortions, the researchers provided AI models with the necessary training data to learn and adapt to peripheral visual cues.
Insights from the Study: Understanding the Gap Between Humans and AI
The results of the study shed light on the challenges and opportunities associated with integrating peripheral vision into AI models. While training AI models with the simulated dataset led to improvements in object detection performance, the models still fell short of human-level accuracy.
Interestingly, factors such as object size and visual clutter, which significantly impact humans, had less influence on the performance of AI models.
Identifying Challenges and Opportunities
Despite significant advancements in AI technology, accurately replicating the complexity of humans remains a formidable challenge.
While existing approaches to modeling peripheral vision in AI often rely on simplistic techniques such as blurring edges of images, the true intricacies extend beyond mere image processing.
One of the key challenges identified by the researchers is the need to develop AI models capable of leveraging contextual information in the same way as humans.
Unlike humans, AI models may not fully utilize contextual cues to enhance object detection and recognition in the visual periphery. Understanding and addressing these differences is essential for narrowing the gap between human and AI performance in peripheral vision tasks.
The Path Forward: Towards Human-Like Visual Perception in AI
Moving forward, researchers aim to bridge the gap between human and AI performance in peripheral vision tasks. By gaining a deeper understanding of the intricacies of peripheral vision, they hope to develop AI models that can predict human behavior more accurately and enhance safety in various domains.
Lead author Anne Harrington emphasizes the potential impact of modeling peripheral vision in AI models, stating, “Modeling peripheral vision, if we can really capture the essence of what is represented in the periphery, can help us understand the features in a visual scene that make our eyes move to collect more information.
” This deeper understanding could lead to significant advancements in driver safety, user experience design, and other applications reliant on visual perception.
Implications for Driver Safety and User Experience
The integration of peripheral vision capabilities into AI models holds immense potential for improving driver safety and user experience.
AI systems equipped with peripheral awareness can anticipate hazards that may go unnoticed by human drivers, leading to enhanced safety on the roads.
Additionally, AI-driven displays and interfaces designed with this in mind could offer users a more intuitive and immersive experience.
Collaboration and Knowledge Sharing
The MIT researchers have made their dataset publicly available, encouraging collaboration and further research in the field of computer vision applications.
By sharing their findings and resources, they hope to inspire other researchers to explore the complexities of its AI models.
Conclusion: A Paradigm Shift in Machine Learning
In conclusion, the pioneering work of MIT researchers in simulating AI models marks a significant advancement in the field of machine learning.
As we strive to create AI systems that perceive the world more like humans do, understanding and replicating the intricacies of it will play a pivotal role in shaping the future of AI technology.
Through continued research and collaboration, we can unlock the full potential of AI and develop more intelligent and perceptive machines.